3DSF-MixNet: Mixer-Based Symmetric Scene Flow Estimation from 3D Point Clouds
ICRA 2024(2024)
摘要
The scene flow estimation aims at accurately achieving the motion of 3D points, imposing challenges like mis-registration, object occlusions, and non-uniform upsampling. This paper introduces a scene flow estimation framework featuring a unified scene flow estimator, a symmetric cost volume approach, and a geometric/semantic feature based upsampling strategy. The novelty of this work is threefold: (1) developing a novel progressive framework which integrates the cost volume module and scene flow estimator, enhancing scene flow estimation. (2) developing a symmetric inter-frame correlation feature extraction method through CV estimation using MLP-Mixer operations; (3) developing an upsampling strategy based on both the semantic and geometric feature similarities between sparse and dense samples. Experiment results show that our method outperforms state-of-the-art baseline methods, especially in scenarios involving challenging conditions, the improvements of our method achieving at most 0.1094m/0.089m/0.091m in EPE3D, 54.23%/53.67%/74.1% in AS, 32.75%/21.87%/40.25% in AR, and 70.981%/58.06%/43.56% in outliers, when tested on FlyingThings3D (FT3D_S, FT3D_H) and KITTI_H datasets, respectively.
更多查看译文
关键词
Deep Learning for Visual Perception,Computer Vision for Transportation
AI 理解论文
溯源树
样例
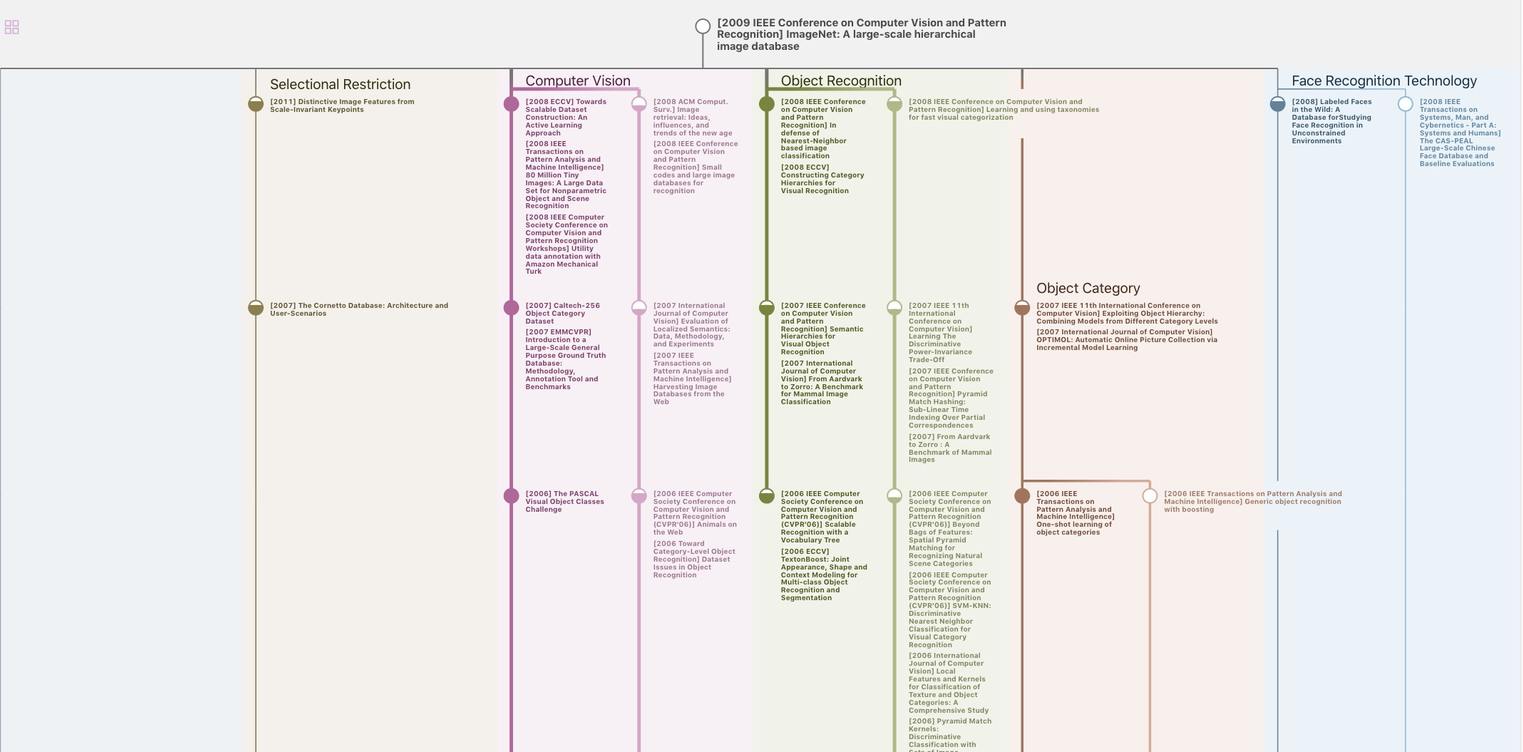
生成溯源树,研究论文发展脉络
Chat Paper
正在生成论文摘要