A Machine Learning Framework for Data Filtering: A Case Study on Chandrayaan-1 SIR-2 Data
2021 IEEE International India Geoscience and Remote Sensing Symposium (InGARSS)(2021)
摘要
Machine learning is emerging as a promising technology with immense applications in various stages of planetary missions. Our focus is to use reflectance data obtained remotely from the Moon surface that can be used for detailed compositional mapping. These datasets need to be adequately preprocessed by removing noise and artifacts before performing an analysis. A traditional way of data preprocessing is to define a set of rules to remove unwanted effects but these methods totally rely on specific information available to the user. Such an approach can significantly improve the quality of the data but unidentified effects may still persist and influence any further analysis. To mitigate this problem, here, we propose to use a new approach which uses Machine Learning algorithms (MLAs) for the preprocessing of all kind of remote sensing data which identified sand filter out unsuitable spectra in the data. We applied MLAs to hyperspectral datasets obtained by the Infrared Spectrometer SIR-2 onboard Chandrayaan-1. In this work, data preprocessing is implemented as a supervised classification problem which aims to classify an input spectrum into desirable/undesirable ones labeled as "GOOD’ and "BAD". The approach was used to filter good spectra from the set of 173 SIR-2 orbits comprising of a total of ~2 million spectra. The methodology followed was a two-stage methodology. In the first stage, subset (~1 million) of data was sampled using systematic sampling. The spectra in the sub-set was manually annotated and different supervised learning approaches namely Logistic regression, Decision tree, KNN, ensemble were applied on this labeled data. On comparing their efficiency using performance metrics, we found five-algorithm voting based ensemble method to be best in terms of accuracy (99.8%) as well as reliability. In the second stage this approach was applied on the remaining unlabeled data to predict the class of spectrum and decide on the usability of that spectrum. As a final outcome, the five-algorithm ensemble approach, classified a total of ~1.35 million spectra as „Good spectra’ and remaining ~1.04 million spectra as ‘Bad spectra’. One of the strength of this approach is that we can obtain comparative performance using very less number of labeled samples. This work demonstrates that our proposed method is suitable for the preprocessing of huge datasets obtained from different missions and of varied nature.
更多查看译文
关键词
data filtering,machine learning framework,machine learning
AI 理解论文
溯源树
样例
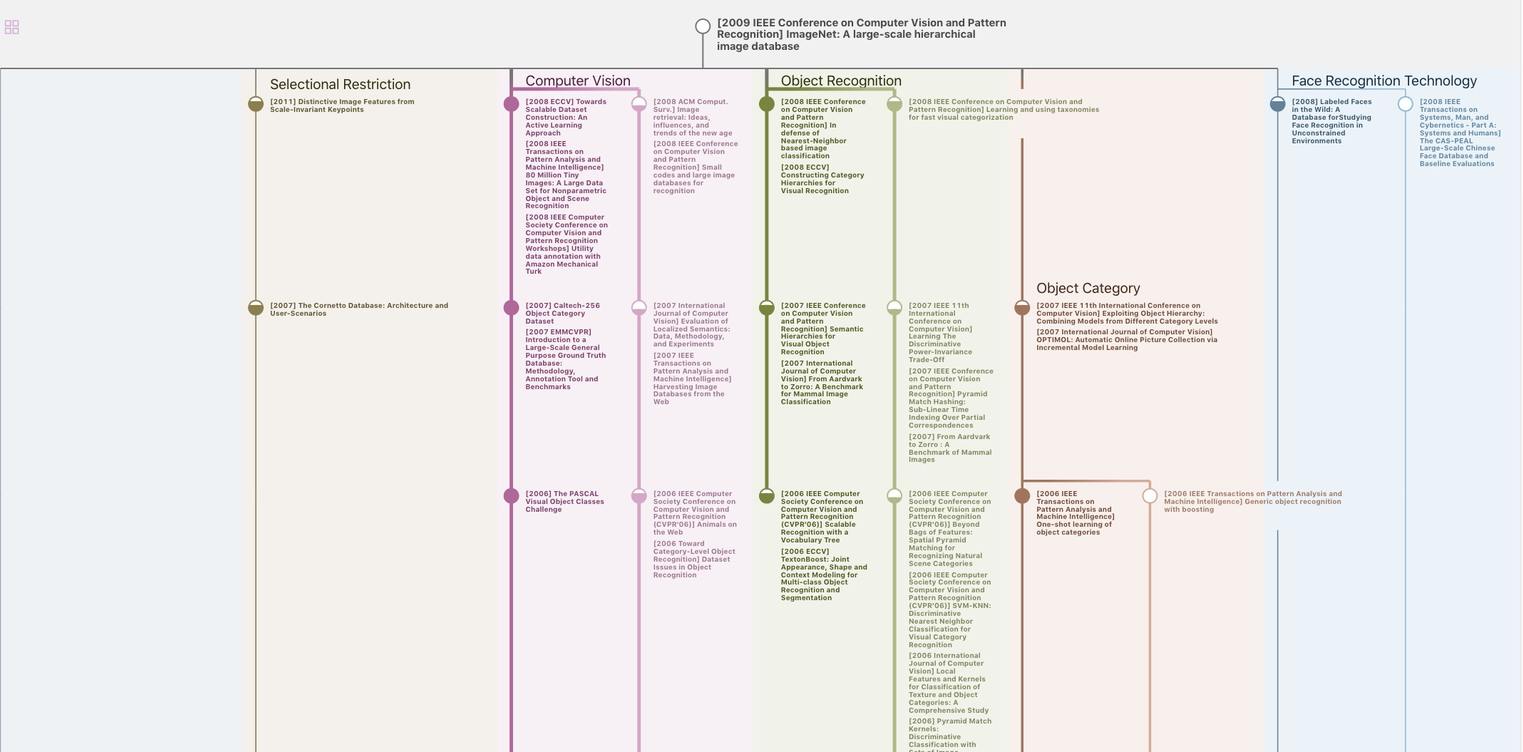
生成溯源树,研究论文发展脉络
Chat Paper
正在生成论文摘要