Hyperspectral Data Assimilation and Road Material Extraction
2021 IEEE International India Geoscience and Remote Sensing Symposium (InGARSS)(2021)
摘要
Hyperspectral Remote Sensing is a sub-branch of remote sensing, which captures information in contiguous spectral bands over a very narrow wavelength range. Hyperspectral data cube captures a large number of datasets in the form of multiple contiguous bands. Data Classification divides the datasets into multiple classes for feature extraction, material identification and temporal change analysis. Selection of proper classification technique is vital for higher accuracy. Single stage classifications sometimes cannot extract sub features within a single class with required accuracy. The current study deploys a multi staged classification approach for the extraction of built-up and road material over a study region. The study compares the performance between various classification techniques like Support Vector Machines (SVM), Maximum Likelihood, Minimum Distance and Mahalanobis Distance. Out of all classification techniques, Support Vector Machines provided the highest accuracy of nearly 90% in both the classification stages. First stage classification using SVM delivered 89.3% accuracy and further 2nd stage classification i.e., Material Identification delivered 90.0% accuracy.
更多查看译文
关键词
Hyperspectral,Classification,Support Vector Machines,Road Extraction
AI 理解论文
溯源树
样例
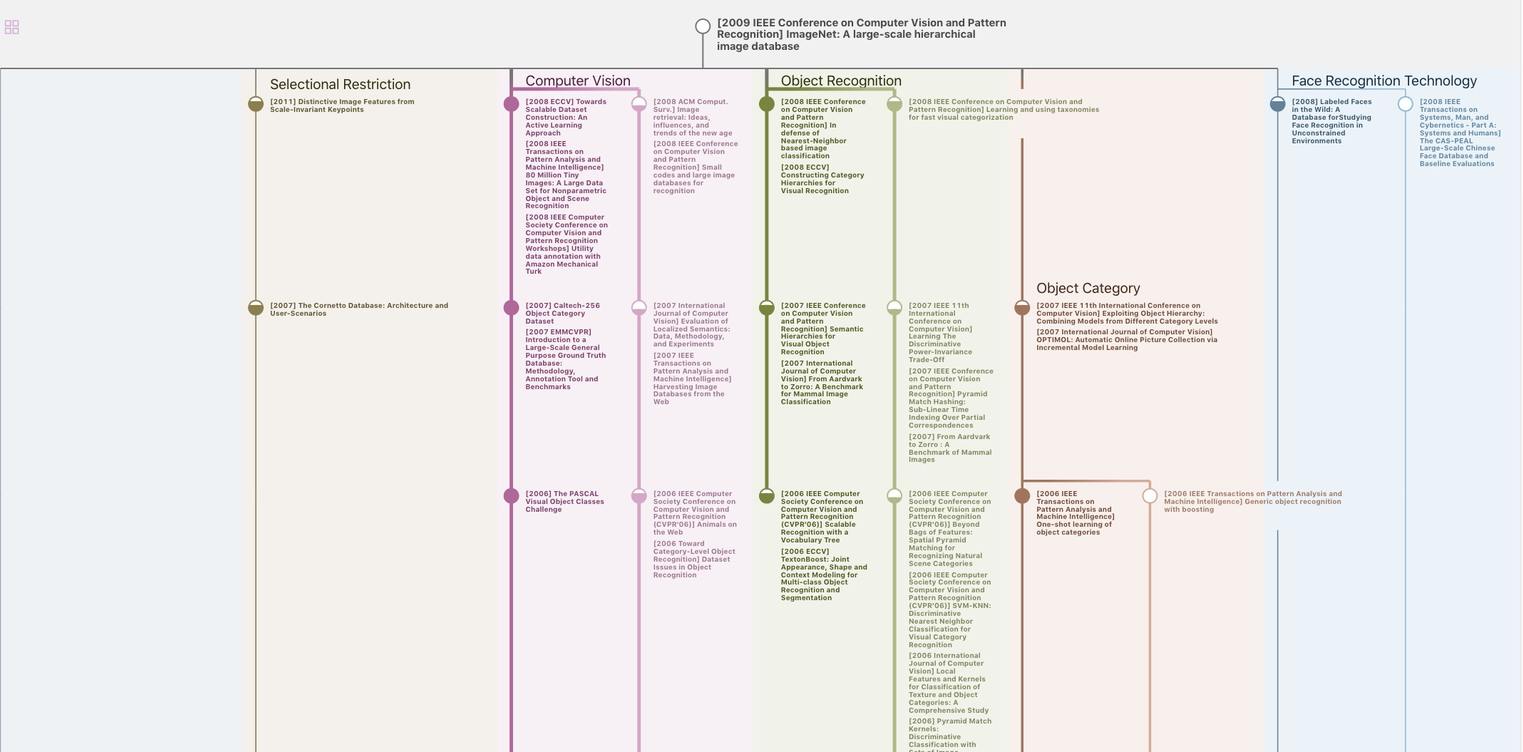
生成溯源树,研究论文发展脉络
Chat Paper
正在生成论文摘要