On learning the structure of sum-product networks
2017 IEEE Symposium Series on Computational Intelligence (SSCI)(2017)
摘要
LearnSPN is the standard unsupervised learning algorithm for sum-product networks (SPNs). It is based upon a “chop” operation for splitting features (columns) and a “slice” operation for clustering instances (rows). However, a number of techniques can be applied to chop and slice meaning that LearnSPN can learn a wide variety of SPNs from the same dataset. In this paper, we perform an empirical study of LearnSPN. We consider g-test and mutual information for chopping and k-means and Gaussian mixture models for slicing. Our experiments, conducted on 20 real-world datasets, suggest that the deepest SPNs tend to be learned when using mutual information for chopping and k-means for slicing. This is important, since SPNs are the only deep learning model where it is provably the case that deeper models are more expressive than shallow models. Second, our results show that the pair of g-test and Gaussian mixture models tends to yield the most accurate SPNs, especially on larger datasets. These results suggest that the particular combination of mutual information and k-means may be prone to overfitting. Lastly, we examine the sparseness of the learned SPN. Our experiments show that the pair of g-test and Gaussian mixture models regularly yields SPNs with fewer edges. This knowledge is beneficial to SPN learning algorithms that penalize networks with more edges. Our study then extends the SPN deep learning literature in both practical and theoretical directions.
更多查看译文
关键词
sum-product networks,LearnSPN,standard unsupervised learning algorithm,chop operation,splitting features,slice operation,clustering instances,SPNs,SPN learning algorithms,shallow models,deeper models,deep learning model,deepest SPNs,real-world datasets,slicing,Gaussian mixture models,chopping,mutual information
AI 理解论文
溯源树
样例
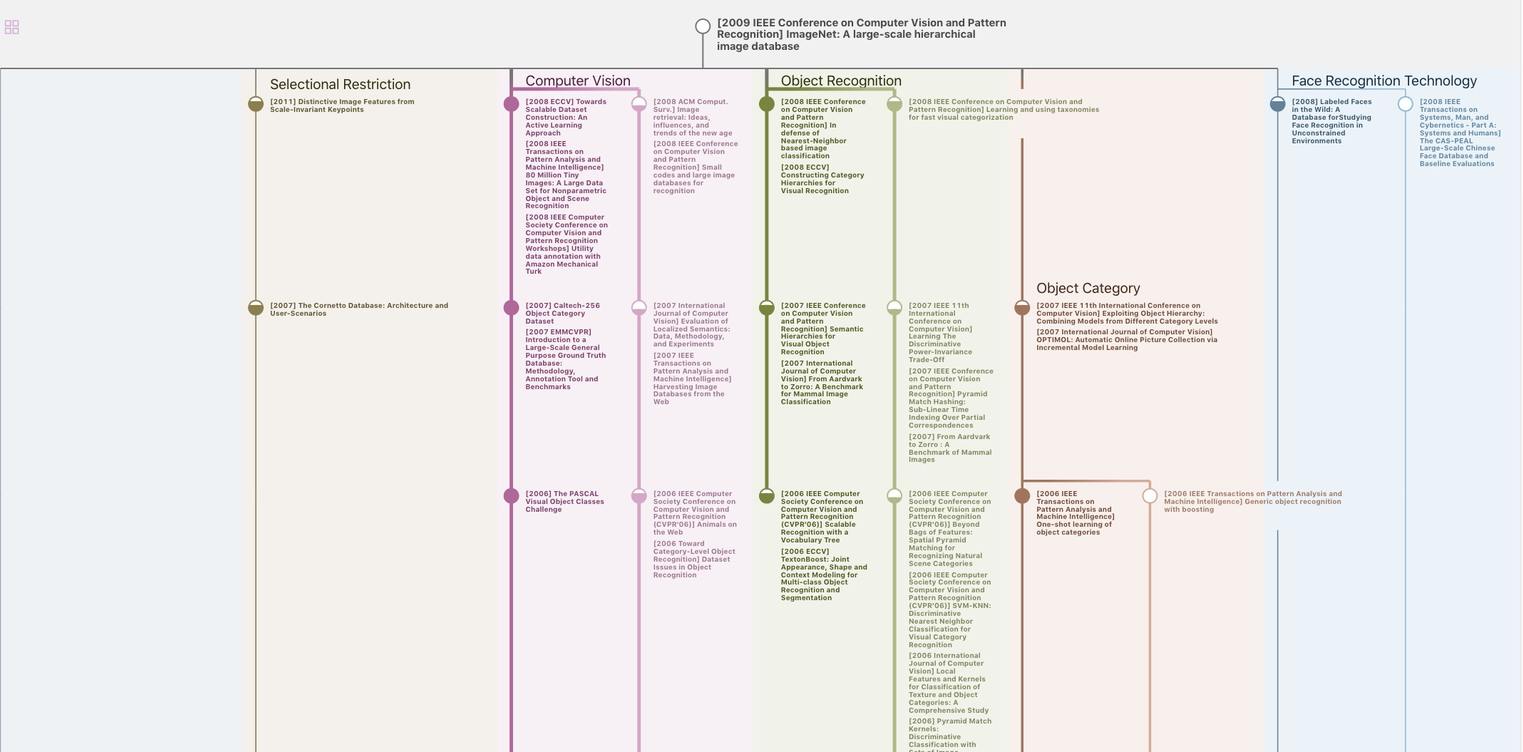
生成溯源树,研究论文发展脉络
Chat Paper
正在生成论文摘要