Do preferences differ based on respondent experience of a health issue and its treatment? A case study using a public health intervention
The European Journal of Health Economics(2022)
摘要
Objectives Preference information is increasingly being elicited to support decision-making. Although discrete choice experiments (DCEs) are commonly used, little is known about how respondents’ relative experience of a health issue, and its treatment, might impact the results of preference studies. The aim of this study was to explore how preferences differ between groups of individuals with varying levels of experience of a health issue and its treatment, using a weight loss maintenance (WLM) programme as a case study. Methods An online DCE survey was provided to four groups, each differing in their level of experience with weight loss and WLM programmes. One group was recruited from a randomised controlled trial of a WLM programme (ISRCTN14657176) and the other three from an online panel. Choice data were analysed using mixed logit models. Relative attribute importance scores and willingness-to-pay (WTP) estimates were estimated to enable comparisons between groups. Results Preferences differed between the groups across different attributes. The largest differences related to the outcome (weight re-gain) and cost attributes, resulting in WTP estimates that were statistically significantly different. The most experienced group was willing to pay £0.35 (95% CI: £0.28, £0.42) to avoid a percentage point increase in weight re-gain, compared with £0.12 (95% CI: £0.08, £0.16) for the least experienced group. Conclusion This study provides evidence in a public health setting to suggest that preferences differ based on respondent experience of the health issue and its treatment. Health preference researchers should therefore carefully consider the appropriate composition of their study samples.
更多查看译文
关键词
Discrete choice experiment, Willingness-to-pay, Experience, Public health intervention, Weight loss maintenance, 110
AI 理解论文
溯源树
样例
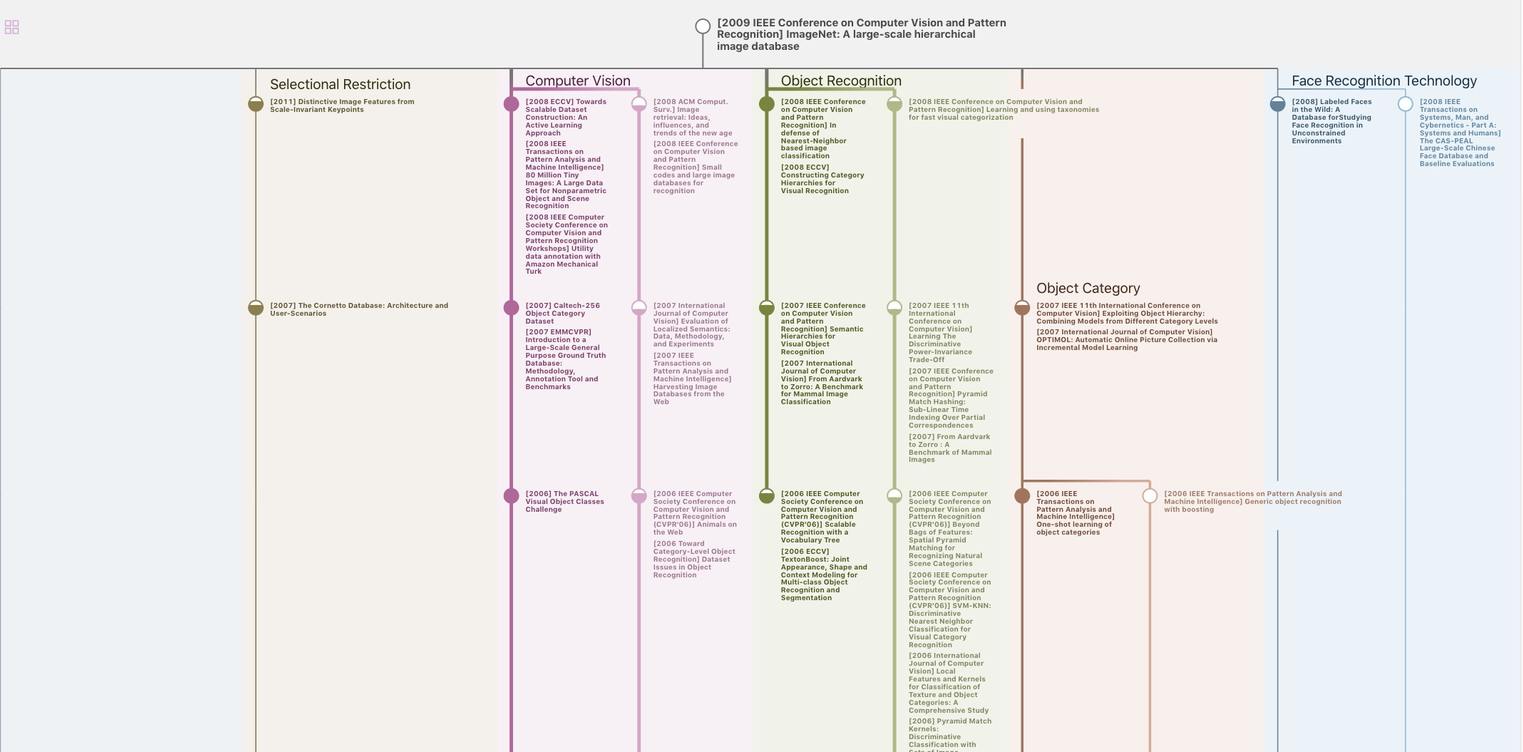
生成溯源树,研究论文发展脉络
Chat Paper
正在生成论文摘要