Soft sensor for predicting indoor PM2.5 concentration in subway with adaptive boosting deep learning model
JOURNAL OF HAZARDOUS MATERIALS(2024)
摘要
Public health depends on indoor air quality (IAQ), hence soft measurement techniques must be implemented in the subway environment for more precise and reliable monitoring of indoor particulate matter concentration levels. Adaptive boosting (AdaBoost), an ensemble learning technique, is simple to code and less prone to overfitting. Compared to a single model, it is better able to take into consideration the intricate elements included in air quality data. It is suggested to use an adaptive boosting of long short-term memory (AdaBoostLSTM) model and kernel principal component analysis (KPCA) for ensemble learning. The kernel function and PCA are first coupled to create KPCA, which is a nonlinear dimensionality reduction method for IAQ. This removes the negative impacts of noise interference. The learning performance of LSTM is then enhanced using AdaBoost as an ensemble learning technique. The KPCA-AdaBoost-LSTM model can deliver higher modeling performance, according to the results. The R2 reached 0.9007 and 0.8995 when predicting PM2.5 in the hall and platform. SHapley Additive exPlanations (SHAP) analysis was used to interpret the input contributions of the model, enhancing the interpretability and transparency of the proposed soft sensor.
更多查看译文
关键词
Adaptive boosting,Ensemble learning,Indoor air quality,Long short-term memory,Soft sensor
AI 理解论文
溯源树
样例
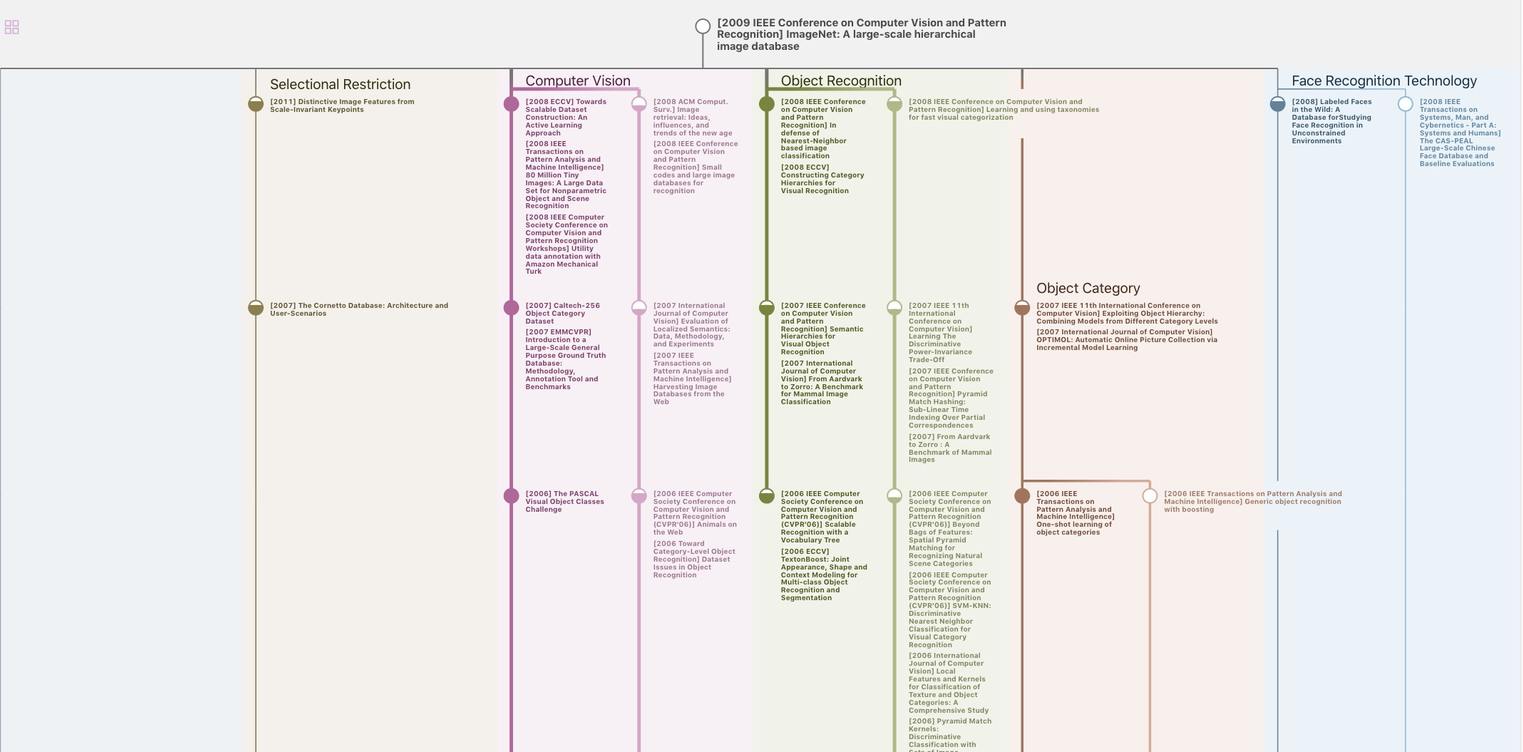
生成溯源树,研究论文发展脉络
Chat Paper
正在生成论文摘要