Image analysis to automatically classify anemia based on Famacha score in sheep using ocular conjunctiva images
TRANSLATIONAL ANIMAL SCIENCE(2023)
摘要
Haemonchus contortus is the most pathogenic blood-feeding parasitic in sheep, causing anemia and consequently changes in the color of the ocular conjunctiva, from the deep red of healthy sheep to shades of pink to practically white of non-healthy sheep. In this context, the Famacha method has been created for detecting sheep unable to cope with the infection by H. contortus, through visual assessment of ocular conjunctiva coloration. Thus, the objectives of this study were (1) to extract ocular conjunctiva image features to automatically classify Famacha score and compare two classification models (multinomial logistic regression-MLR and random forest-RF) and (2) to evaluate the applicability of the best classification model on three sheep farms. The dataset consisted of 1,156 ocular conjunctiva images from 422 animals. RF model was used to segment the images, i.e., to select the pixels that belong to the ocular conjunctiva. After segmentation, the quantiles (1%, 10%, 20%, 30%, 40%, 50%, 60%, 70%, 80%, 90%, and 99%) of color intensity in each image channel (red, blue, and green) were determined and used as explanatory variables in the classification models, and the Famacha scores 1 (non-anemic) to 5 (severely anemic) were the target classes to be predicted (scores 1 to 5, with 162, 255, 443, 266, and 30 images, respectively). For objective 1, the performance metrics (precision and sensitivity) were obtained using MLR and RF models considering data from all farms randomly split. For objective 2, a leave-one-farm-out cross-validation technique was used to assess prediction quality across three farms (farms A, B, and C, with 726, 205, and 225 images, respectively). The RF provided the best performances in predicting anemic animals, as indicated by the high values of sensitivity for Famacha score 3 (80.9%), 4 (46.2%), and 5 (60%) compared to the MLR model. The precision of the RF was 72.7% for Famacha score 1 and 62.5% for Famacha score 2. These results indicate that is possible to successfully predict Famacha score, especially for scores 2 to 4, in sheep via image analysis and RF model using ocular conjunctiva images collected in farm conditions. As expected, model validation excluding entire farms in cross-validation presented a lower prediction quality. Nonetheless, this setup is closer to reality because the developed models are supposed to be used across farms, including new ones, and with different environments and management conditions. It is possible to successfully predict Famacha score, especially for scores 2 to 4, in sheep via image analysis combined with a random forest classification model using ocular conjunctiva images collected in farm conditions. An extremely useful tool for identifying parasitized animals with Haemonchus contortus through the diagnosis of anemic is the Famacha method, which provides scores based on visual assessment of ocular conjunctiva coloration. However, this method is error-prone, since it requires a subjective evaluation from the professionals performing the analysis. Image analyses can help to obtain more accurate identification of parasitized animals, eliminating human subjectivity in its assessment. Thus, the objectives of this study were to extract ocular conjunctiva image features to automatically classify anemia based on Famacha score, compare two classification models, and evaluate the applicability of the best classification model on three sheep farms. Among the classification models, the random forest had the best performance. As expected, model validation excluding entire farms in cross-validation presented a lower prediction quality. It was demonstrated that it is possible to successfully predict Famacha score, especially for scores 2 to 4, in sheep via image analysis and random forest classification model using ocular conjunctiva images collected in farm conditions.
更多查看译文
关键词
gastrointestinal nematodes, machine learning, ovis aries, random forest
AI 理解论文
溯源树
样例
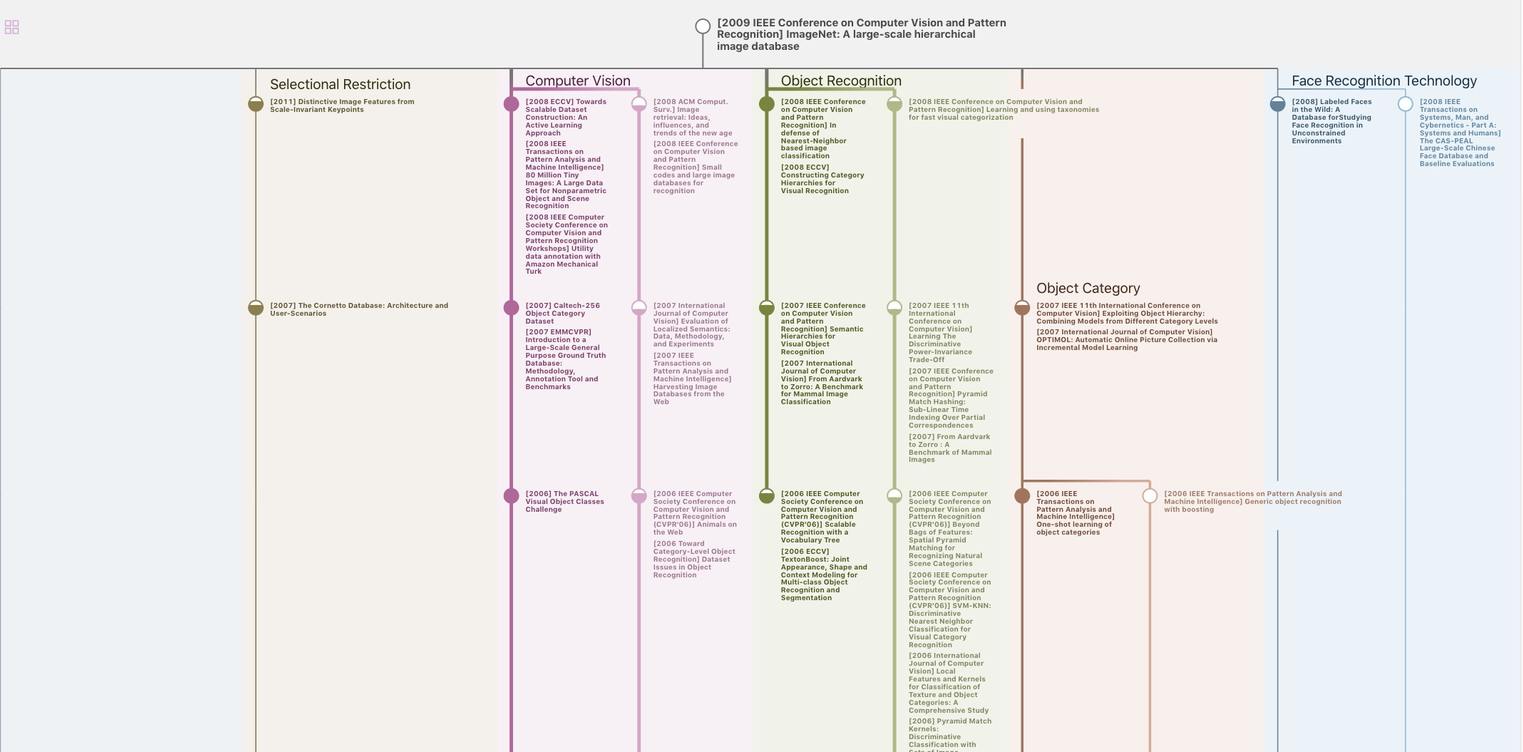
生成溯源树,研究论文发展脉络
Chat Paper
正在生成论文摘要