Invariance-informed reduced-order sparse modeling of partial differential equations with DCA-ℓ1−2 algorithm
2022 34th Chinese Control and Decision Conference (CCDC)(2022)
摘要
Learning invariant subspaces is a critical step in the construction of accurate reduced-order models for partial differential equations. In this work, we propose a fully data-driven modeling method for learning the finite-dimensional representations of partial differential equations, by restricting partial differential operator to a linear invariant subspace forming a fundamental set of solutions of an ordinary differential equation. To identify a set of functions forming the linear invariant subspace, we use the proper orthogonal decomposition method to detect the spatial basis functions from data, which sequently results in a reduced dimensional surrogate model. Further, we propose an approach using the sparse learning method to discover the reduced dimensional model from data. Particularly, we formulate the sparse learning problem into a
1−2
minimization problem and use an iterative computational method based on the difference of convex functions algorithm to solve the optimization problem. We present several numerical tests, including the Schrödinger equation and the semi-linear non-local parabolic equation, to illustrate our approach and to show the effectiveness of the method in comparison to exact solutions.
更多查看译文
关键词
Partial differential equations,Data-driven,Reduced-order modeling,Sparse optimization
AI 理解论文
溯源树
样例
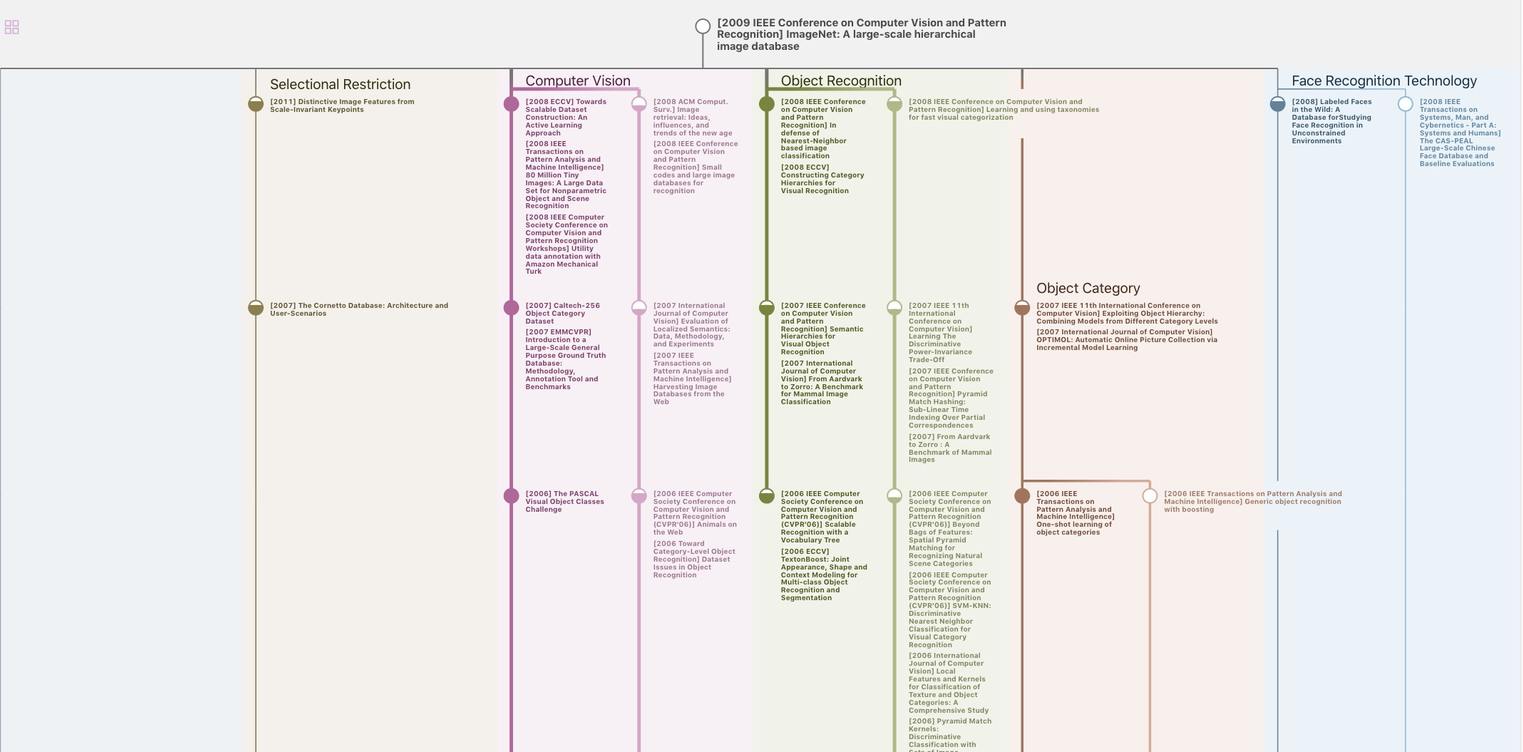
生成溯源树,研究论文发展脉络
Chat Paper
正在生成论文摘要