ClustML: A measure of cluster pattern complexity in scatterplots learnt from human-labeled groupings
INFORMATION VISUALIZATION(2024)
摘要
Visual quality measures (VQMs) are designed to support analysts by automatically detecting and quantifying patterns in visualizations. We propose a new VQM for visual grouping patterns in scatterplots, called ClustML, which is trained on previously collected human subject judgments. Our model encodes scatterplots in the parametric space of a Gaussian Mixture Model and uses a classifier trained on human judgment data to estimate the perceptual complexity of grouping patterns. The numbers of initial mixture components and final combined groups quantify visual cluster patterns in scatterplots. It improves on existing VQMs, first, by better estimating human judgments on two-Gaussian cluster patterns and, second, by giving higher accuracy when ranking general cluster patterns in scatterplots. We use it to analyze kinship data for genome-wide association studies, in which experts rely on the visual analysis of large sets of scatterplots. We make the benchmark datasets and the new VQM available for practical use and further improvements.
更多查看译文
关键词
Visual quality measure,cluster pattern,data-driven,Gaussian mixture model,perceptual data
AI 理解论文
溯源树
样例
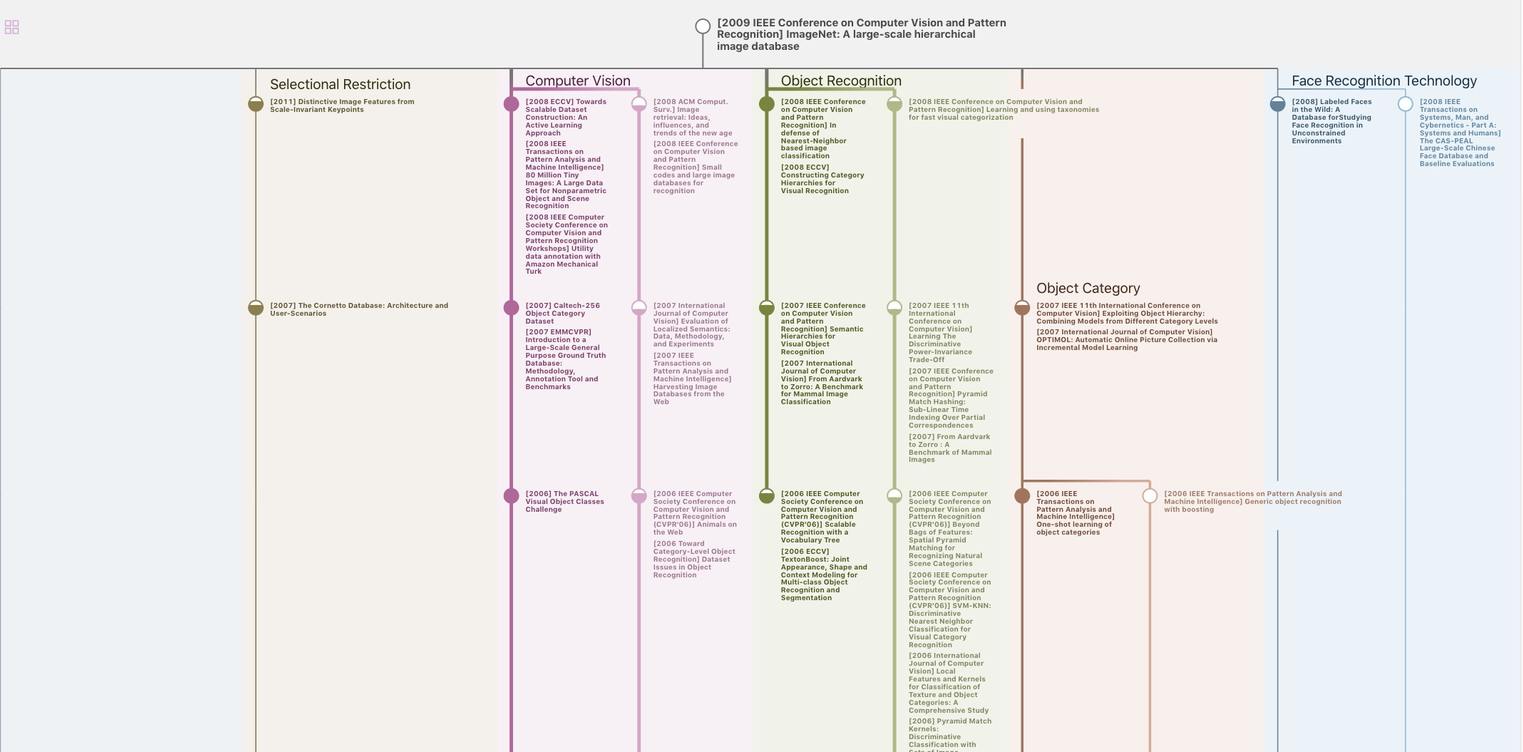
生成溯源树,研究论文发展脉络
Chat Paper
正在生成论文摘要