Fourier Image Transformer
2022 IEEE/CVF Conference on Computer Vision and Pattern Recognition Workshops (CVPRW)(2022)
摘要
Transformer architectures show spectacular performance on NLP tasks and have recently also been used for tasks such as image completion or image classification. Here we propose to use a sequential image representation, where each prefix of the complete sequence describes the whole image at reduced resolution. Using such Fourier Do-main Encodings (FDEs), an auto-regressive image completion task is equivalent to predicting a higher resolution out-put given a low-resolution input. Additionally, we show that an encoder-decoder setup can be used to query arbitrary Fourier coefficients given a set of Fourier domain observations. We demonstrate the practicality of this approach in the context of computed tomography (CT) image reconstruction. In summary, we show that Fourier Image Trans-former (FIT) can be used to solve relevant image analysis tasks in Fourier space, a domain inherently inaccessible to convolutional architectures.
更多查看译文
关键词
Fourier domain encodings,auto-regressive image completion task,encoder-decoder,Fourier domain observations,computed tomography image reconstruction,Fourier image transformer,NLP tasks,sequential image representation,image analysis tasks,FDEs,CT image reconstruction,image classification,FIT
AI 理解论文
溯源树
样例
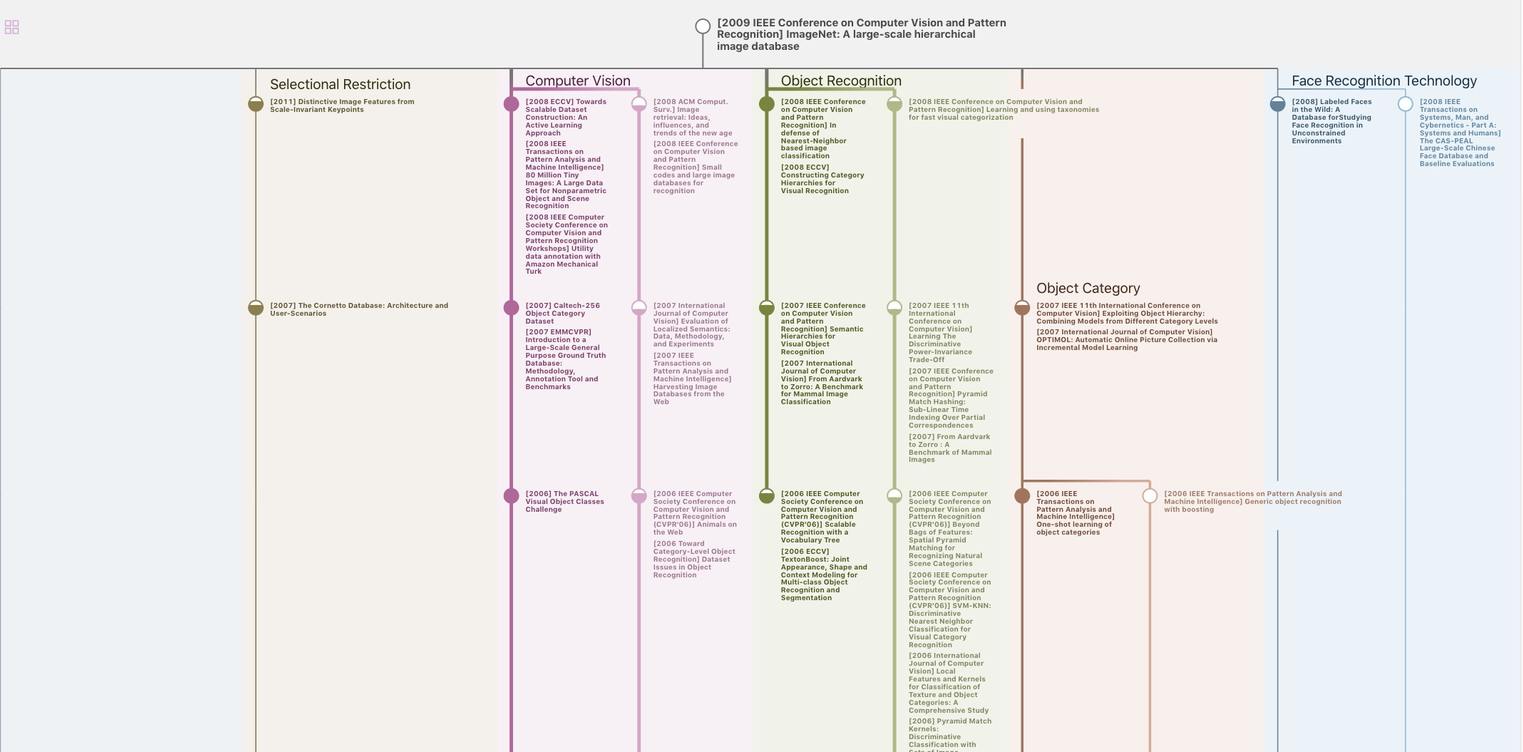
生成溯源树,研究论文发展脉络
Chat Paper
正在生成论文摘要