SATHUR: Self Augmenting Task Hallucinal Unified Representation for Generalized Class Incremental Learning
2023 IEEE/CVF INTERNATIONAL CONFERENCE ON COMPUTER VISION WORKSHOPS, ICCVW(2023)
摘要
Class Incremental Learning (CIL) is inspired by the human ability to learn new classes without forgetting previous ones. CIL becomes more challenging in real-world scenarios when the samples in each incremental step are imbalanced. This creates another branch of problem, called Generalized Class Incremental Learning (GCIL) where each incremental step is structured more realistically. Grow When Required (GWR) network, a type of Self-Organizing Map (SOM), dynamically creates and removes nodes and edges for adaptive learning. GWR performs incremental learning from feature vectors extracted by a Convolutional Neural Network (CNN), which acts as a feature extractor. The inherent ability of GWR to form distinct clusters, each corresponding to a class in the feature vector space, regardless of the order of samples or class imbalances, is well suited to achieving GCIL. To enhance GWR's classification performance, a high-quality feature extractor is required. However, when the convolutional layers are adapted at each incremental step, the GWR nodes corresponding to prior knowledge are subjected to near-invalidation. This work introduces the Self Augmenting Task Hallucinal Unified Representation (SATHUR), which re-initializes the GWR network at each incremental step, aligning it with the incrementally updated feature extractor. Comprehensive experimental results demonstrate that our proposed method significantly outperforms other state-of-the-art GCIL methods on CIFAR-100 and CORe50 datasets.
更多查看译文
关键词
Continual Learning,Class Incremental Learning,Transfer Learning,Representation Learning
AI 理解论文
溯源树
样例
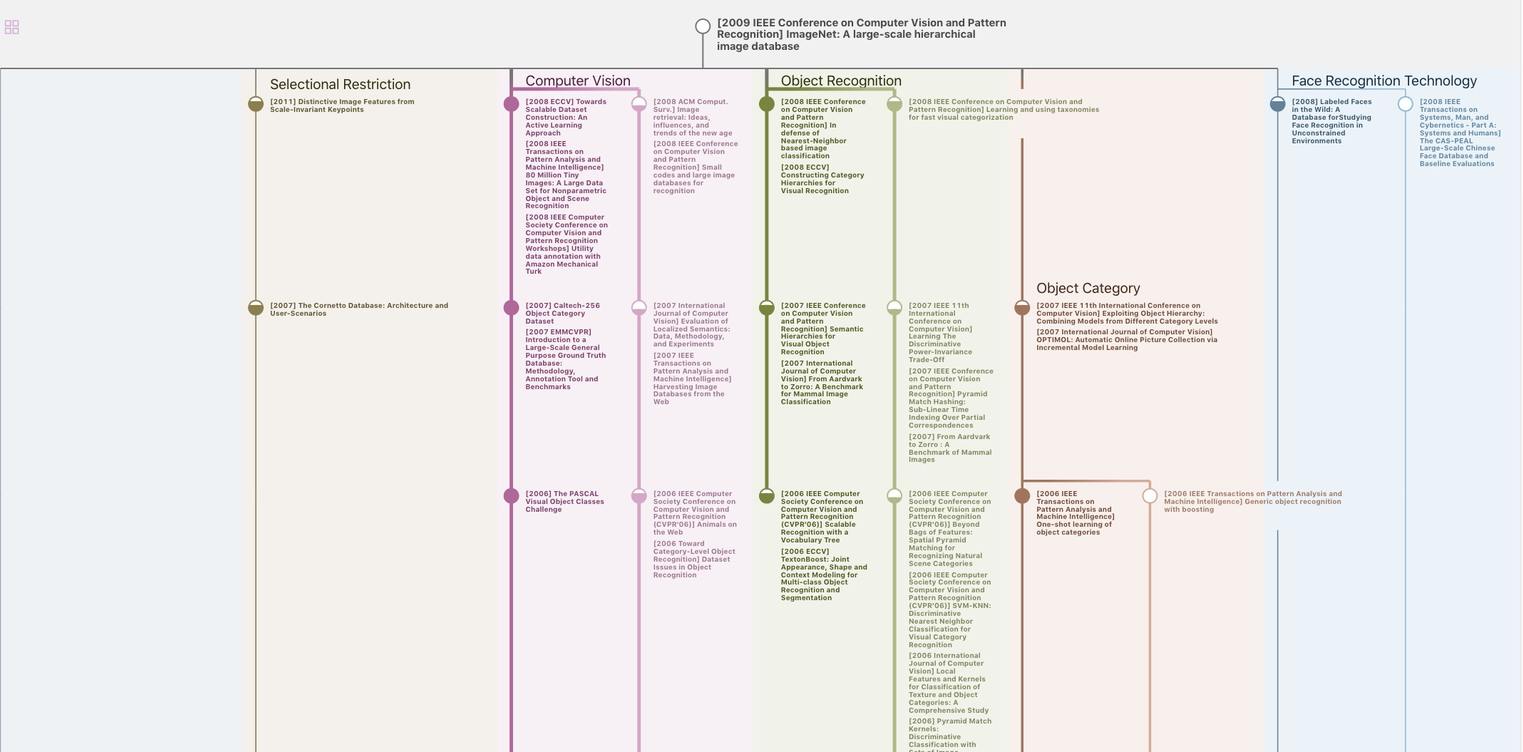
生成溯源树,研究论文发展脉络
Chat Paper
正在生成论文摘要