MViTv2: Improved Multiscale Vision Transformers for Classification and Detection
2022 IEEE/CVF Conference on Computer Vision and Pattern Recognition (CVPR)(2022)
摘要
In this paper, we study Multiscale Vision Transformers (MViTv2) as a unified architecture for image and video classification, as well as object detection. We present an improved version of MViT that incorporates decomposed relative positional embeddings and residual pooling connections. We instantiate this architecture in five sizes and evaluate it for ImageNet classification, COCO detection and Kinetics video recognition where it outperforms prior work. We further compare MViTv2s' pooling attention to window attention mechanisms where it outperforms the latter in accuracy/compute. Without bells-and-whistles, MViTv2 has state-of-the-art performance in 3 domains: 88.8% accuracy on ImageNet classification, 58.7 AP
box
on COCO object detection as well as 86.1% on Kinetics-400 video classification. Code and models are available at https://github.com/facebookresearch/mvit.
更多查看译文
关键词
Recognition: detection,categorization,retrieval, Deep learning architectures and techniques, Representation learning, Video analysis and understanding
AI 理解论文
溯源树
样例
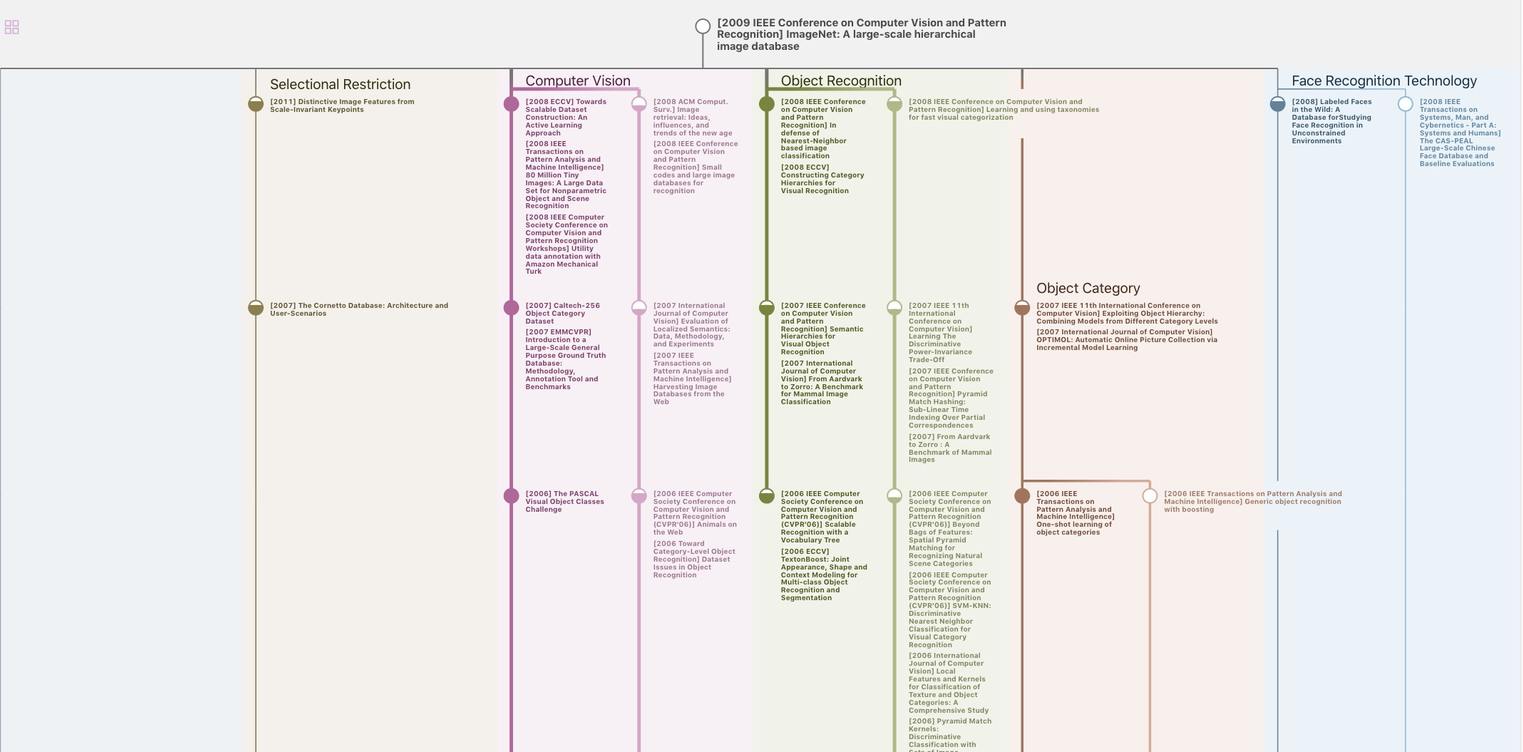
生成溯源树,研究论文发展脉络
Chat Paper
正在生成论文摘要