S3: Neural Shape, Skeleton, and Skinning Fields for 3D Human Modeling
2021 IEEE/CVF Conference on Computer Vision and Pattern Recognition (CVPR)(2021)
摘要
Constructing and animating humans is an important component for building virtual worlds in a wide variety of applications such as virtual reality or robotics testing in simulation. As there are exponentially many variations of humans with different shape, pose and clothing, it is critical to develop methods that can automatically reconstruct and animate humans at scale from real world data. Towards this goal, we represent the pedestrian’s shape, pose and skinning weights as neural implicit functions that are directly learned from data. This representation enables us to handle a wide variety of different pedestrian shapes and poses without explicitly fitting a human parametric body model, allowing us to handle a wider range of human geometries and topologies. We demonstrate the effectiveness of our approach on various datasets and show that our reconstructions outperform existing state-of-the-art methods. Furthermore, our re-animation experiments show that we can generate 3D human animations at scale from a single RGB image (and/or an optional LiDAR sweep) as input.
更多查看译文
关键词
neural shape,skeleton,3D human modeling,virtual reality,pose clothing,neural implicit functions,human parametric body model,human geometries,re-animation experiments,3D human animations,pedestrian shapes,skinning field,human reconstruction,RGB image
AI 理解论文
溯源树
样例
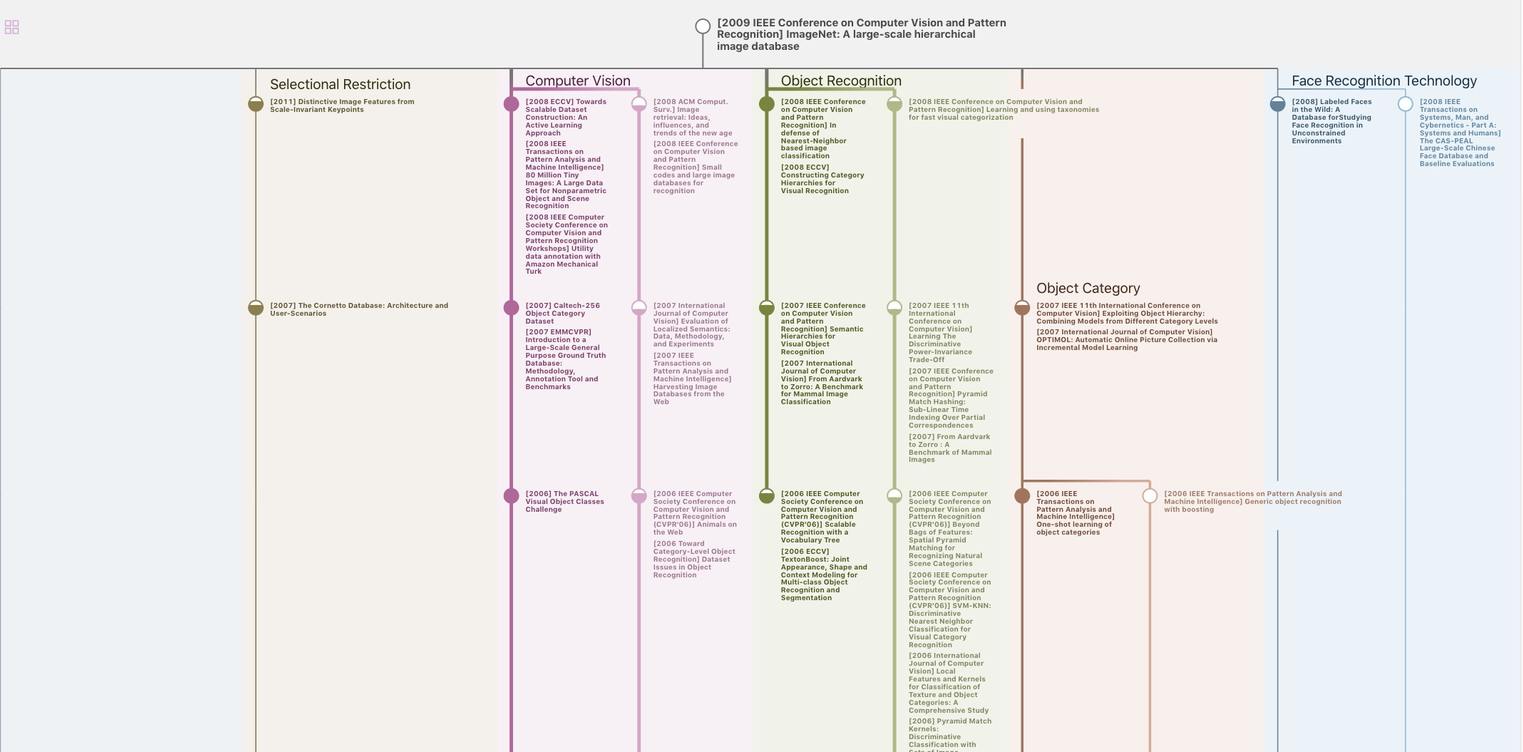
生成溯源树,研究论文发展脉络
Chat Paper
正在生成论文摘要