KTGAT: Improving the Robustness of Knowledge-enhanced Text Generation via Adversarial Training
2023 4th International Conference on Computer Engineering and Application (ICCEA)(2023)
摘要
The shortage of information in text generation has been a prominent area of research in Natural Language Processing (NLP). Current research endeavors aim to combine pre-trained models with rich open-world knowledge from external sources to increase the priori information and thereby enhance the informativeness of text generation. While recent studies suggest that integrating open-world and task-specific knowledge can improve text generation by addressing specific knowledge gaps in downstream tasks, the inherent semantic ambiguity in natural language remains a significant challenge that may impede knowledge acquisition and text generation. To overcome this challenge and improve the model's semantic comprehension and overall robustness, we propose a novel framework, the Knowledge Augmentation Text Generation model via Adversarial Training (KTGAT). Our method adds perturbations to the embedding layer, which are equivalent to constructing unstable samples. This approach improves the model's robustness to adversarial samples and the generalization performance of the original samples. Our experiments demonstrate that the proposed KTGAT framework outperforms the baseline model, thus proving its effectiveness in improving text generation. The generated text cases illustrate that our method enhances the model's semantic comprehension and enables it to search for knowledge items more effectively and accurately.
更多查看译文
关键词
Text generation,Pre-trained models,Knowledge fusion,Adversarial training
AI 理解论文
溯源树
样例
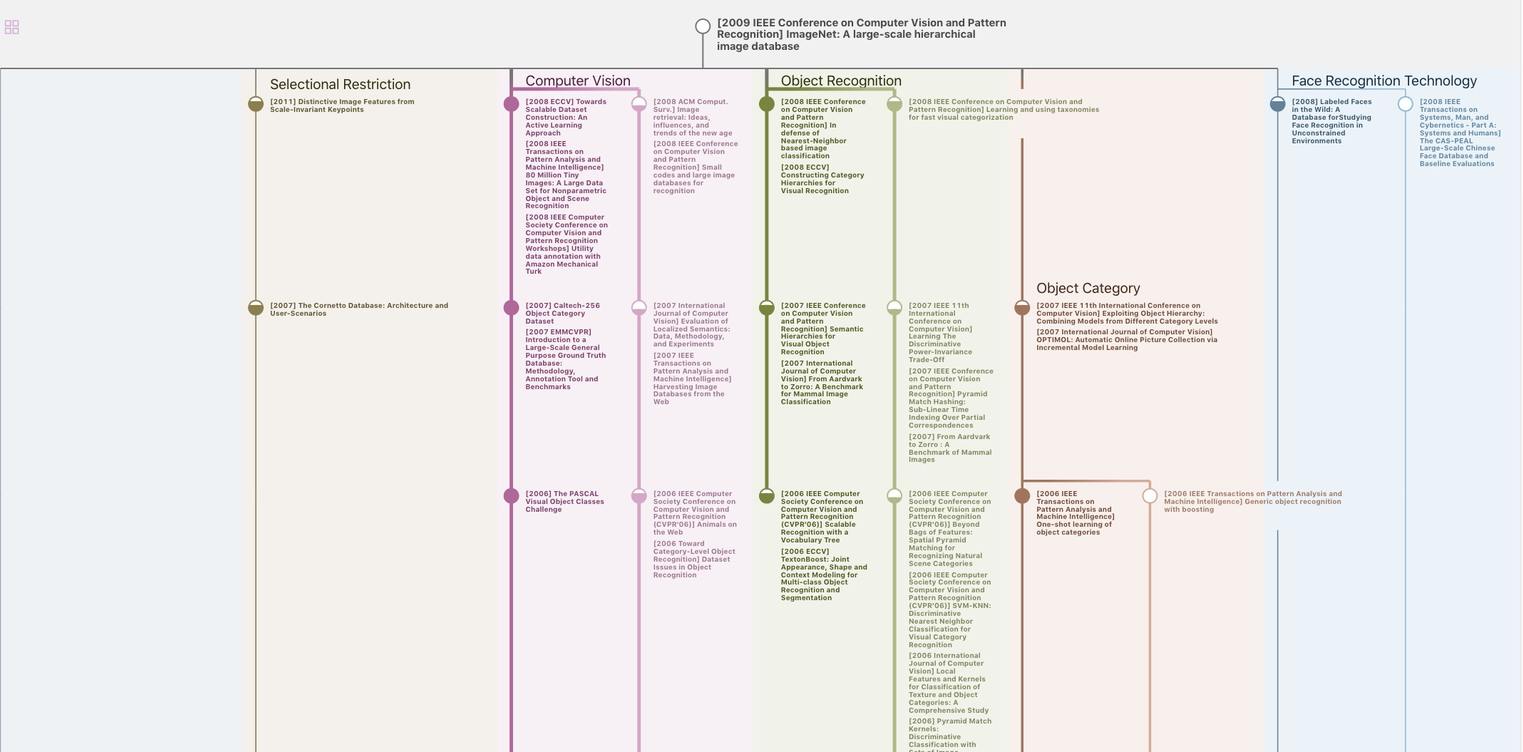
生成溯源树,研究论文发展脉络
Chat Paper
正在生成论文摘要