Automated Anatomical Feature Detection for Completeness of Abdominal FAST Exam
2023 IEEE International Ultrasonics Symposium (IUS)(2023)
Abstract
The Focused Assessment with Sonography in Trauma (FAST) exam is a crucial tool for swiftly identifying intraperitoneal hemorrhage in trauma patients. Accurate interpretation of FAST results relies on clinicians’ capacity to thoroughly visualize regions corresponding to potential fluid accumulation across three abdominal zones: the right upper quadrant, left upper quadrant, and suprapubic zones. To ensure comprehensive zones, it is imperative to visualize the essential organs within these zones. Automating the identification of key organs can guide all users in capturing complete zone and enhance diagnostic precision, particularly for less-experienced practitioners. In this study, we propose a deep learning-based approach for both classifying zones and localizing key organs during abdominal FAST examinations. We introduce two distinct methods for zone classification and organ detection. Initially, we build a mobile classification network for processing multi-frame inputs. For organ detection, we employ a single-stage detector to identify key anatomical features in 2D frames. Finally, we report that a combining the outputs from these two approaches results in a model with improved diagnostic accuracy.
MoreTranslated text
Key words
FAST Exam,Trauma,Ultrasound Imaging Analysis,Computer Vision,Deep Learning
AI Read Science
Must-Reading Tree
Example
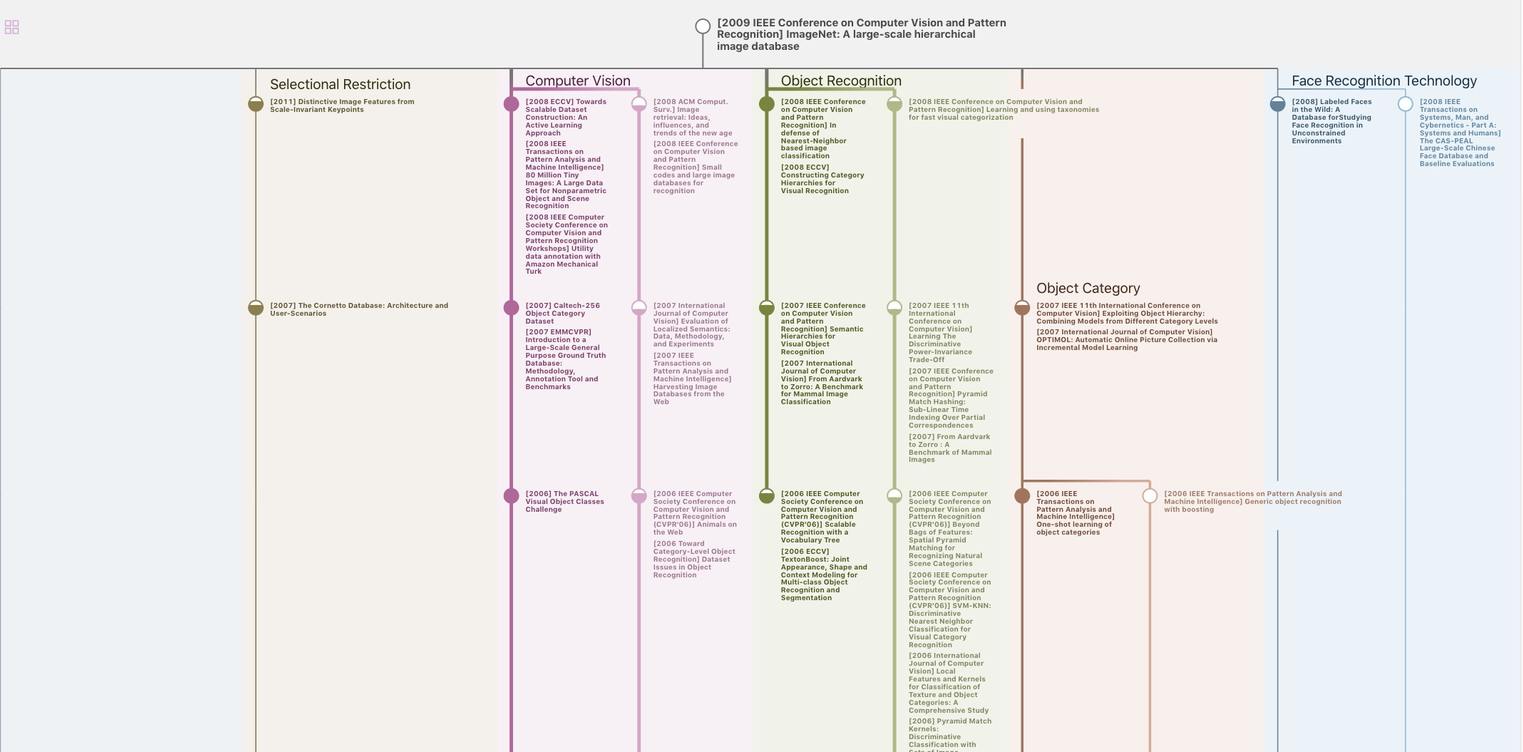
Generate MRT to find the research sequence of this paper
Chat Paper
Summary is being generated by the instructions you defined