Learning Clear Class Separation for Open-set 3D Detector in Autonomous Vehicle via Selective Forgetting
2023 32ND IEEE INTERNATIONAL CONFERENCE ON ROBOT AND HUMAN INTERACTIVE COMMUNICATION, RO-MAN(2023)
摘要
A trustworthy 3D detector is essential in the perception system of autonomous vehicles, ensuring accurate detection of their surroundings. However, autonomous vehicles have to operate in ever-changing real-world driving scenes, where unknown objects that do not belong to the training set are commonly encountered. Confusion about known and unknown objects could result in severe and dangerous consequences for road safety. To address this problem, we improve the reliability of autonomous driving systems by formulating open-set 3D object detection task. An Open-set 3D Detector (Open3Det) is proposed to reject unknown instances while maintaining performance on known categories. Distinct from 2D objects, clear space separation exists between each 3D instance. Motivated by this, we propose selective forgetting, a novel method capable of filtering out misleading predictions. Given a close-set teacher model, knowledge distillation is introduced to build a open-set student model. The student model preserves its predictions for known objects, whereas predictions of backgrounds and unknown instances are discarded to minimize misleading results. Extensive experiments and visualizations reveal the efficacy of the proposed method.
更多查看译文
AI 理解论文
溯源树
样例
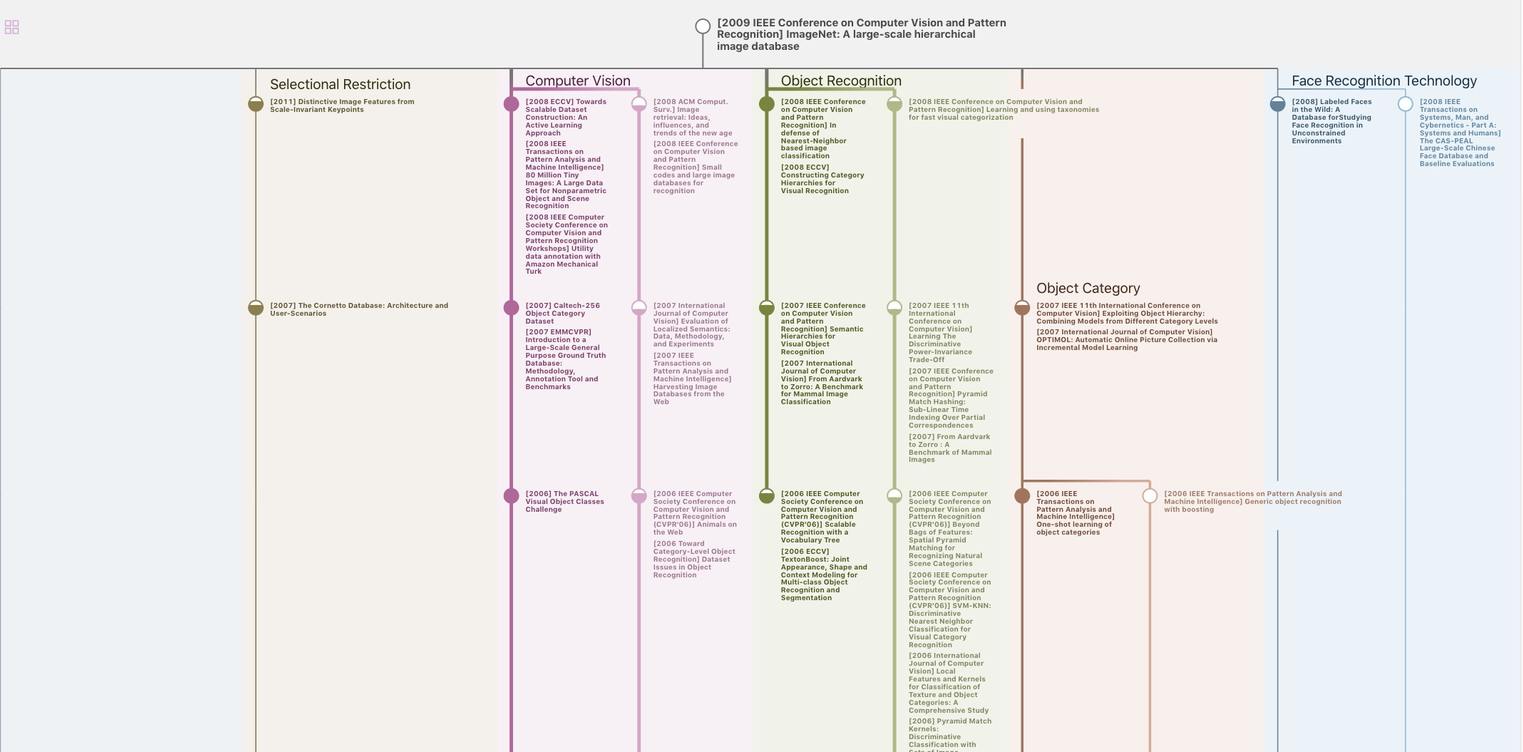
生成溯源树,研究论文发展脉络
Chat Paper
正在生成论文摘要