A Novel Meta Control Framework for Robot Arm Reaching with Changeable Configuration
2023 32ND IEEE INTERNATIONAL CONFERENCE ON ROBOT AND HUMAN INTERACTIVE COMMUNICATION, RO-MAN(2023)
摘要
When deploying a robot to real-world environments, it is crucial to execute tasks amidst constantly changing surroundings. The conventional kinematics control of robot arms is primarily reliant on the inverse kinematics model. Unfortunately, due to the lack of adaptability, high-precision control models often falter when the robot utilizes tools of varying lengths or when the robot arm is worn out. This work aims to address this issue by proposing a meta-learning-based control framework. We achieve rapid and seamless online adaptation by updating control models when the robot arm's configuration changes. The control framework comprises an Adaptive Global Inverse Model (Adaptive GIM) and an Adaptive Local Inverse Model (Adaptive LIM). The Adaptive GIM employs configuration-independent meta-learning, which allows the control model to swiftly adapt to different arm configurations. The Adaptive LIM adopts a meta-learning approach for location-independent training, enabling the robot to adapt to diverse local positions. As the Adaptive GIM suffers from the adverse effects stemming from the multiple solutions of inverse kinematics, utilizing the Adaptive LIM with relative position as input can alleviate this issue and enable more precise reaching towards the target. Extensive validation conducted on PKU-HR6.0 demonstrates that the proposed approach significantly enhances online adaptation speed and precision compared to existing methods.
更多查看译文
AI 理解论文
溯源树
样例
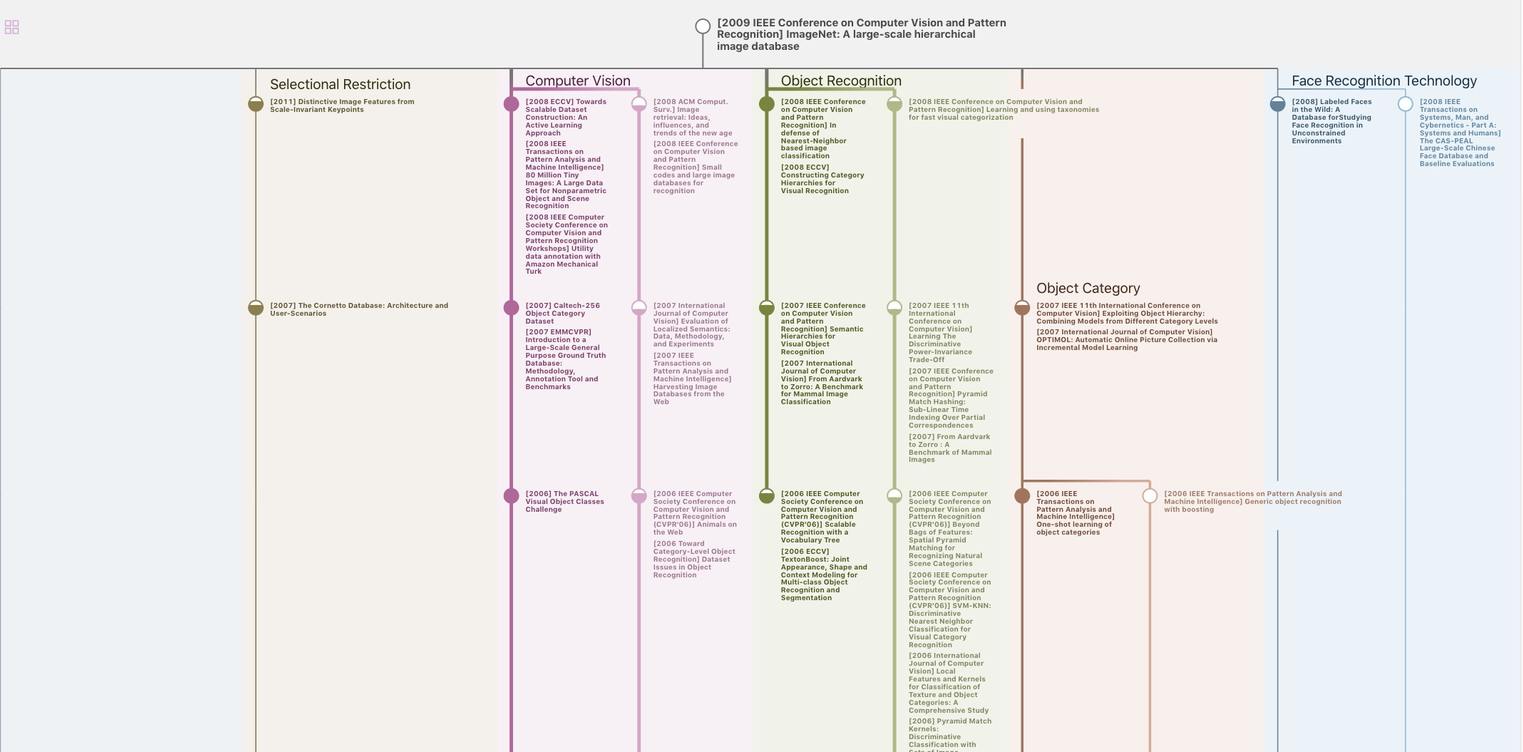
生成溯源树,研究论文发展脉络
Chat Paper
正在生成论文摘要