A Comparative Study on Satellite Image Analysis for Road Traffic Detection using YOLOv3-SPP, Keras RetinaNet and Full Convolutional Network
2023 8th International Conference on Business and Industrial Research (ICBIR)(2023)
Abstract
Satellite imagery has wide ranging applications that enable different fields including transportation. The authors would like to leverage this technology in terms of collecting traffic data. Traffic data is vital information in calibrating volume-delay functions to forecast demand to ensure efficient route plan outputs. This study aims to assess the readiness level of heavily trained object detection models in detecting vehicles occupying small pixels on open source satellite images. The results of the study show that the Full Convolutional Network outperforms YOLOv3-SPP and RetinaNet models with an accuracy of 92%, 81%, and 48% respectively. Considering other factors, the proponents have concluded as well that the YOLOv3-SPP model has the potential to surpass FCN given certain preconditions indicated in the recommendation section of the paper. Despite the poor performance of RetinaNet in this study, its capability was not discounted completely. In fact, RetinaNet architecture can be seen as more strategic than the YOLO family because it pays close attention to regions, which is a good fit for satellite images. Although it falls short on detecting as many vehicles as possible, it has a very high confidence level in its detection, so misclassification is almost not an issue for this model.
MoreTranslated text
Key words
deep learning,neural network,road traffic,satellite imaging,semantic segmentation
AI Read Science
Must-Reading Tree
Example
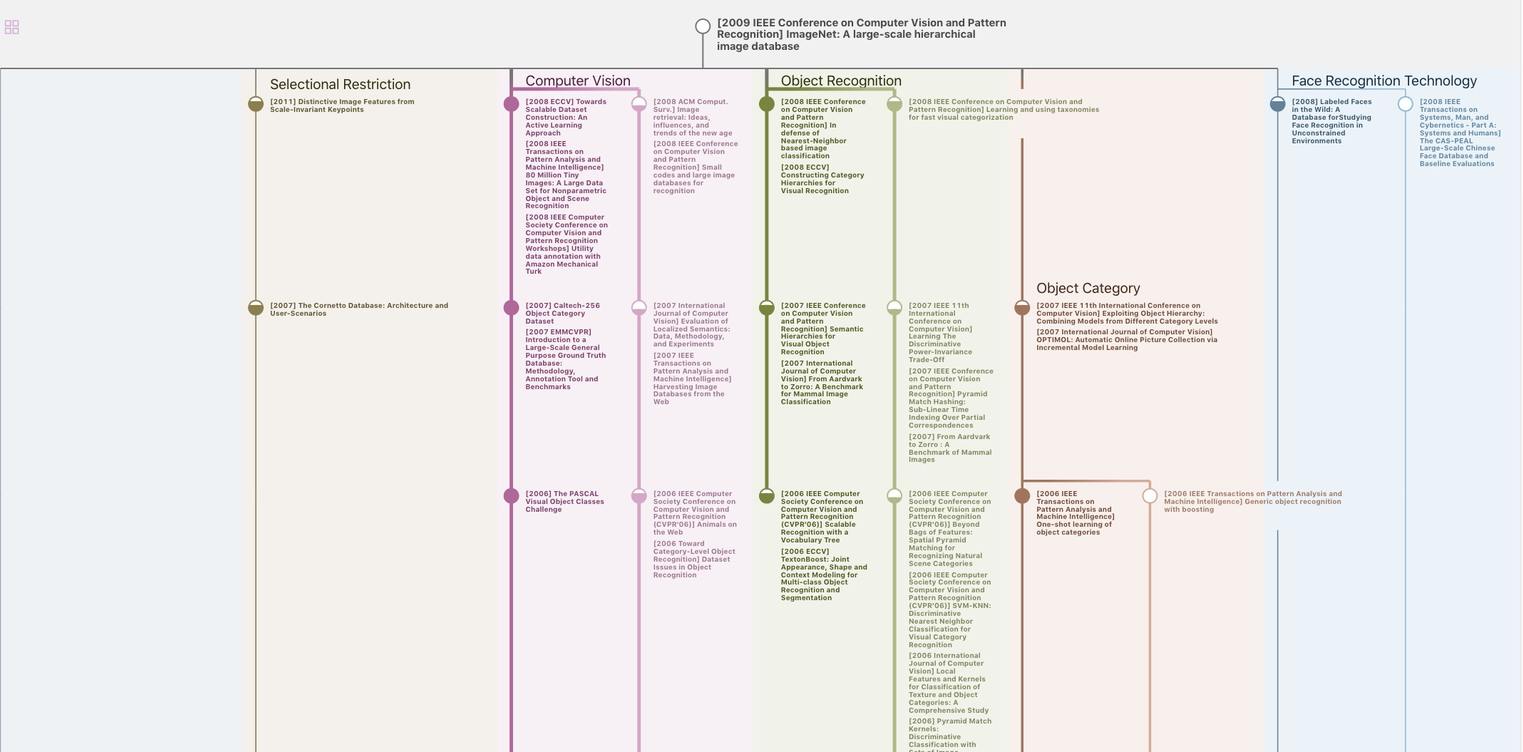
Generate MRT to find the research sequence of this paper
Chat Paper
Summary is being generated by the instructions you defined