A Simple Attention Block Embedded in Standard CNN for Image Classification
2022 International Conference on Applied Artificial Intelligence and Computing (ICAAIC)(2022)
Abstract
The revival of convolution neural networks (CNN) has enhanced feature extraction by reducing the manual hand-engineered methods. These CNN proved their versatility in various domains, such as image classification, object detection, and image segmentation (ROI). Variant neural architectures have been proposed over the past decade with increasing depth, width, and channels for precise feature extraction. In images, feature extraction is a crucial step. The CNN will be able to extract the spatial information, but they can't retrieve the spatial orientations of the entities residing in the image. Further, attention to the required features is not seen. These points are considered challenges, and a neural architecture is to be constructed by overhauling these loops. Hence, we propose an attention block which can be easily embedded into standard convolution neural networks and eventually outperforms the plain CNN on CIFAR-10 and the NIH Malaria Data Set. The code is publicly available at: https://github.com/barulalithb/scaled-mean-attention.
MoreTranslated text
Key words
Convolution Neural Networks,Computer Vision,Deep learning,Attention Mechanism,Malaria Data set
AI Read Science
Must-Reading Tree
Example
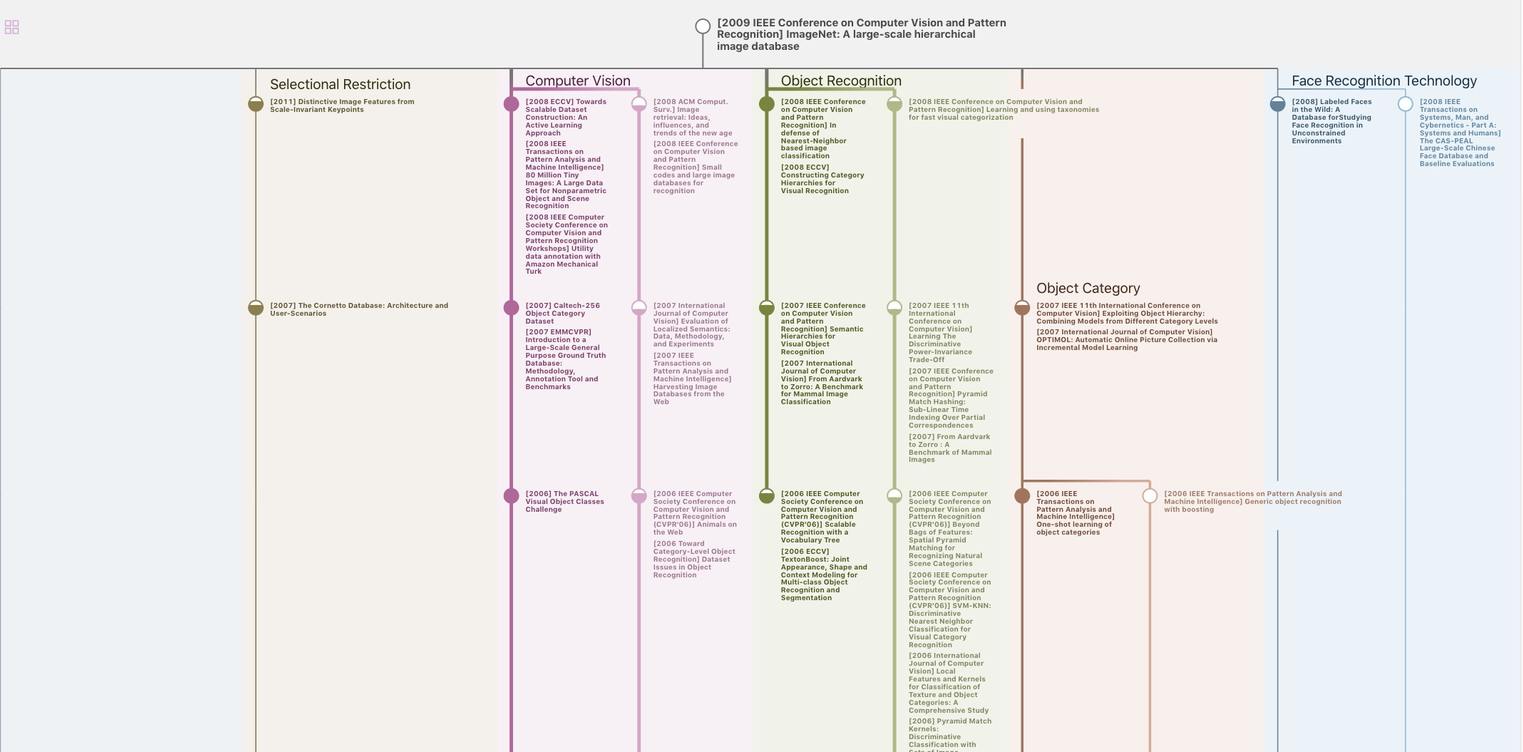
Generate MRT to find the research sequence of this paper
Chat Paper
Summary is being generated by the instructions you defined