Transfer Learning Based Photovoltaic Power Forecasting with XGBoost
2023 Panda Forum on Power and Energy (PandaFPE)(2023)
Abstract
Photovoltaic power forecasting plays a significant role in the operation of power system with high renewables. Owing to the powerful data mining ability, the artificial intelligence learning based models have achieved impressive success in photovoltaic power forecasting. However, most of these methods cannot be directly employed in the cases where there is not sufficient historical data to train a reliable model. Fortunately, transfer learning can be utilized to address this problem by exploiting the knowledge learned from related areas with sufficient data. In this paper, a novel adaptive transfer learning framework based on extreme gradient boosting is proposed. In the proposed framework, several (one) extreme gradient boosting based models are firstly trained based on the data of source domains (target domain). Then, transferability analysis is conducted by comparing the similarity of SHAP value distribution between each of the obtained models in source domain and the one obtained in target domain. The model in the source domain with the high similarity is selected to transfer knowledge to target domain. Case studies conducted on open dataset demonstrates that the proposed framework can improve the forecasting accuracy of photovoltaic power with limited data.
MoreTranslated text
Key words
photovoltaic power forecasting,transfer learning,XGBoost,transferability analysis,SHAP value
AI Read Science
Must-Reading Tree
Example
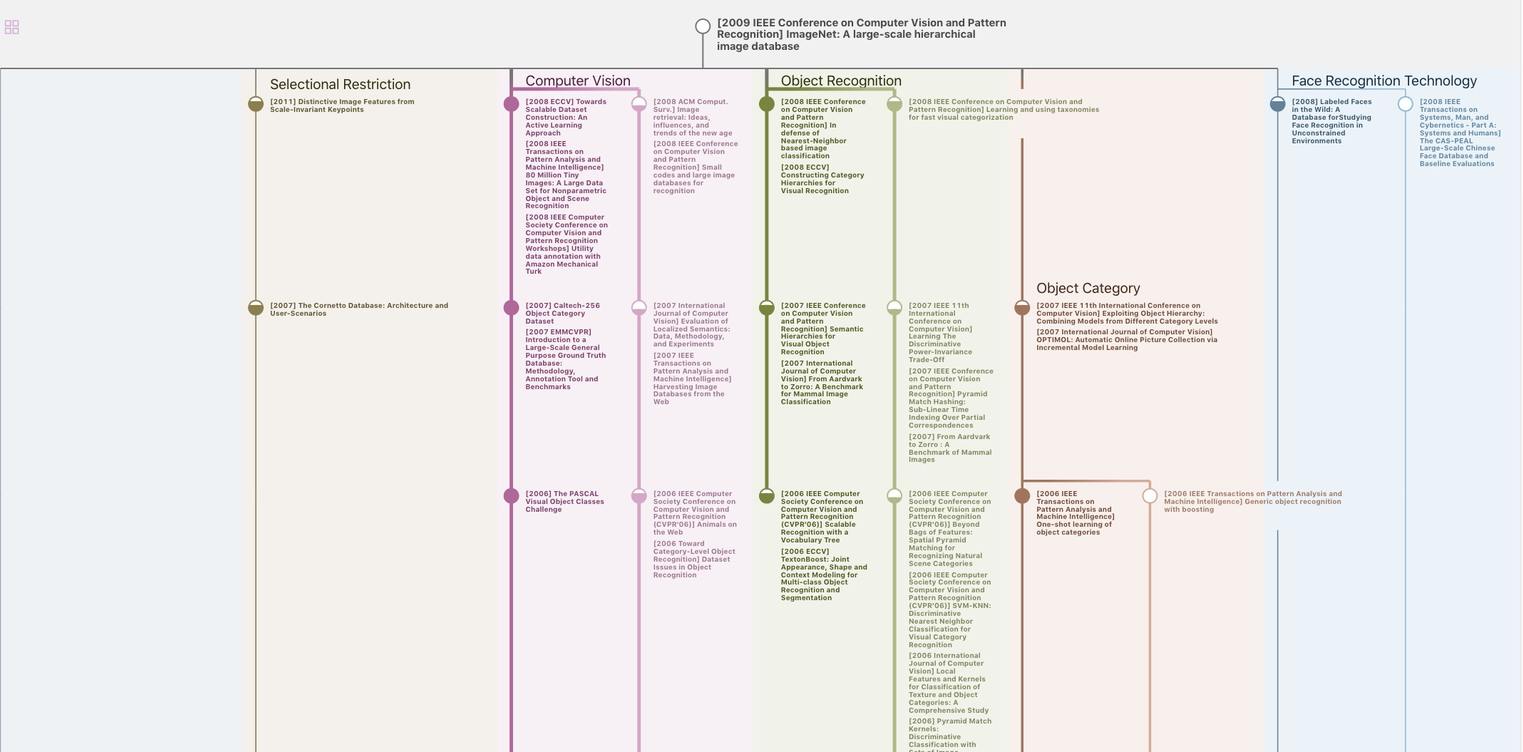
Generate MRT to find the research sequence of this paper
Chat Paper
Summary is being generated by the instructions you defined