Multi-Scale Anomaly Detection in Fiber Optic Current Transformers
2023 IEEE 6th International Electrical and Energy Conference (CIEEC)(2023)
摘要
As an important component for measuring direct current in converter stations, fiber optical current transformers (FOCT) are widely applied in the smart power grid to ensure the safety of AC/DC conversion processes by quantifying the polarization rotation when light passes through a magnetic field. Predicting the states of FOCTs is crucial for the security of smart power gird. However, the states of FOCTs are associated with many external factors, and it is challenging to predict the anomalies timely. To this end, in this paper we present an ensemble-based prediction model that leverages the strengths of XGBoost, LSTM, and Informer to predict the states of FOCTs in a multi-scale way. Our proposed model takes into account the influences of multiple variables on FOCT operation and considers the accuracy of long-sequence and short-sequence time-series forecasting while avoiding the randomness, nonlinearity, and instability of external factors. Finally, we evaluate our proposed ensemble model on a large-scale dataset collected from state grid corporation of China. Our experimental results demonstrate that our proposed fusion model has higher stability and generalization capability compared to traditional single models, and it performs well on both the short-term and long-term prediction tasks.
更多查看译文
关键词
Fiber Optical Current Transformer,Ensemble Learning,Long Short-Term Time Series Prediction,Blending model
AI 理解论文
溯源树
样例
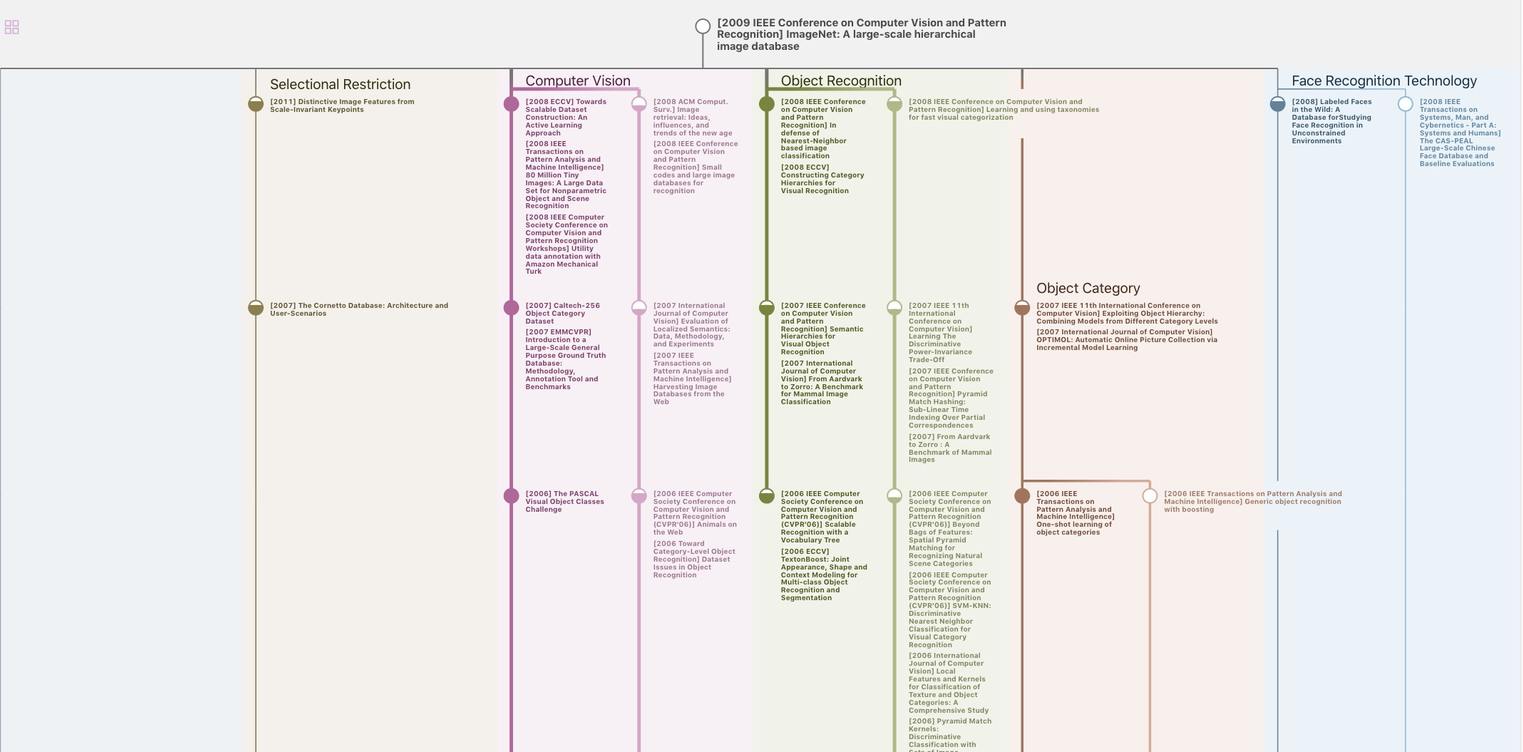
生成溯源树,研究论文发展脉络
Chat Paper
正在生成论文摘要