Rapid quantization of battery degradation mode based on multi-head attention model of Variational autoencoder
2023 IEEE 6th International Electrical and Energy Conference (CIEEC)(2023)
摘要
Lithium battery is widely used in various industries due to its excellent performance. Due to the huge demand, the diagnosis and prediction of lithium battery are paid more and more attention by researchers. This paper aims at the rapid quantification of battery degradation patterns. The rapid diagnosis of battery degradation patterns can optimize the battery management system and better prevent the adverse consequences of battery aging. In this paper, a data-driven method based on Variational autoencoder(VAE) is used to quantity the battery degradation patterns quickly and convert the sequence signals to image signals. The VAE network is used as the feature extractor and transformer module is used as the battery degradation patterns quantifier. At the same time, through training and testing with existing advanced methods and some common neural networks on common commercial battery data sets, the results show that the proposed model has achieved good experimental results, and verifies the feasibility and effectiveness of the model.
更多查看译文
关键词
lithium battery degradation mode,Rapid diagnosis,VAE,transformer
AI 理解论文
溯源树
样例
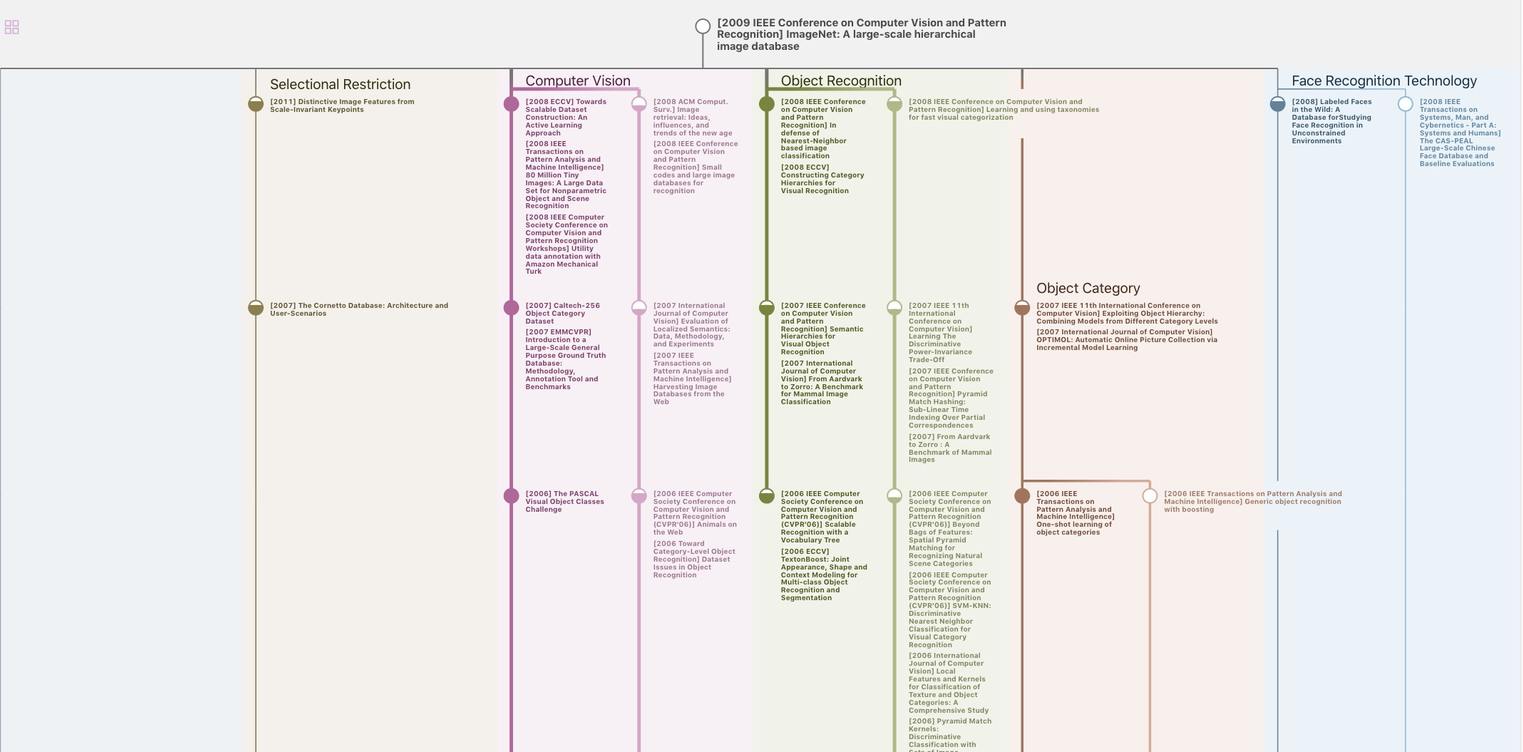
生成溯源树,研究论文发展脉络
Chat Paper
正在生成论文摘要