Easing Predictors Selection in Electricity Price Forecasting with Deep Learning Techniques
2023 19th International Conference on the European Energy Market (EEM)(2023)
摘要
This paper explores the application of Deep Learning techniques to forecast electricity market prices. Three Deep Learning (DL) techniques are tested: Dense Neural Networks (DNN), Long Short-Term Memory Networks (LSTM) and Convolutional Neural Networks (CNN); and two non-DL techniques: Multiple Linear Regression and Gradient Boosting (GB). First, this work compares the forecast skill of all techniques for electricity price forecasting. The results analysis showed that CNN consistently remained among the best performers when predicting the most unusual periods such as the Covid19 pandemic one. The second study evaluates the potential application of CNN for automatic feature extraction over a dataset composed by multiple explanatory variables of different types, overcoming part of the feature selection challenges. The results showed that CNNs can be used to reduce the need for a variable selection phase.
更多查看译文
关键词
Electricity Price Forecasting,Deep Learning,Convolutional Neural Networks,Dense Neural Networks,Long Short-Term Memory,Gradient Boosting,Feature selection
AI 理解论文
溯源树
样例
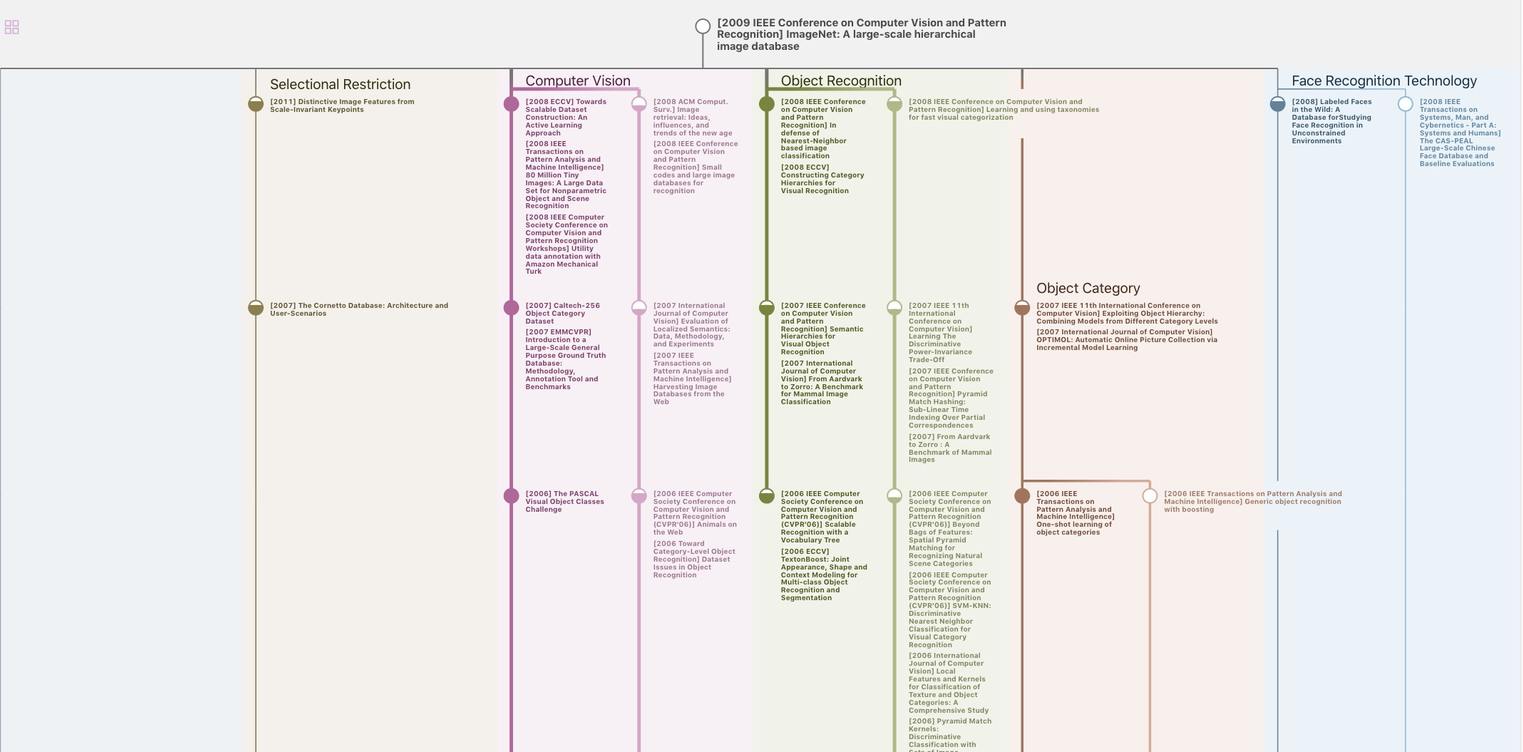
生成溯源树,研究论文发展脉络
Chat Paper
正在生成论文摘要