Ensemble Learning-Based Mortality Prediction After Acute Myocardial Infarction
Journal of Shanghai Jiaotong University (Science)(2023)
摘要
A mortality prediction model based on small acute myocardial infarction (AMI) patients coherent with low death rate is established. In total, 1 639 AMI patients are selected as research objects who received treatment in seven tertiary and secondary hospitals in Shanghai between January 1, 2016 and January 1, 2018. Among them, 72 patients deceased during the two-year follow-up. Models are established with ensemble learning framework and machine learning algorithms based on 51 physiological indicators of the patient. Shapley additive explanations algorithm and univariate test with point-biserial and phi correlation coefficients are employed to determine significant features and rank feature importance. Based on 5-fold cross validation experiment and external validation, prediction model with self-paced ensemble framework and random forest algorithm achieves the best performance with area under receiver operating characteristic curve (AUROC) score of 0.911 and recall of 0.864. Both feature ranking methods showed that ejection fractions, serum creatinine (admission), hemoglobin and Killip class are the most important features. With these top-ranked features, the simplified prediction model is capable of achieving a comparable result with AUROC score of 0.872 and recall of 0.818. This work proposes a new method to establish mortality prediction models for AMI patients based on self-paced ensemble framework, which allows models to achieve high performance with small scale of patients coherent with low death rate. It will assist in medical decision and prognosis as a new reference.
更多查看译文
关键词
acute myocardial infarction (AMI), ensemble learning, machine learning, feature engineering, R 542.2+2, A, 急性心肌梗死, 集成学习, 机器学习, 特征工程
AI 理解论文
溯源树
样例
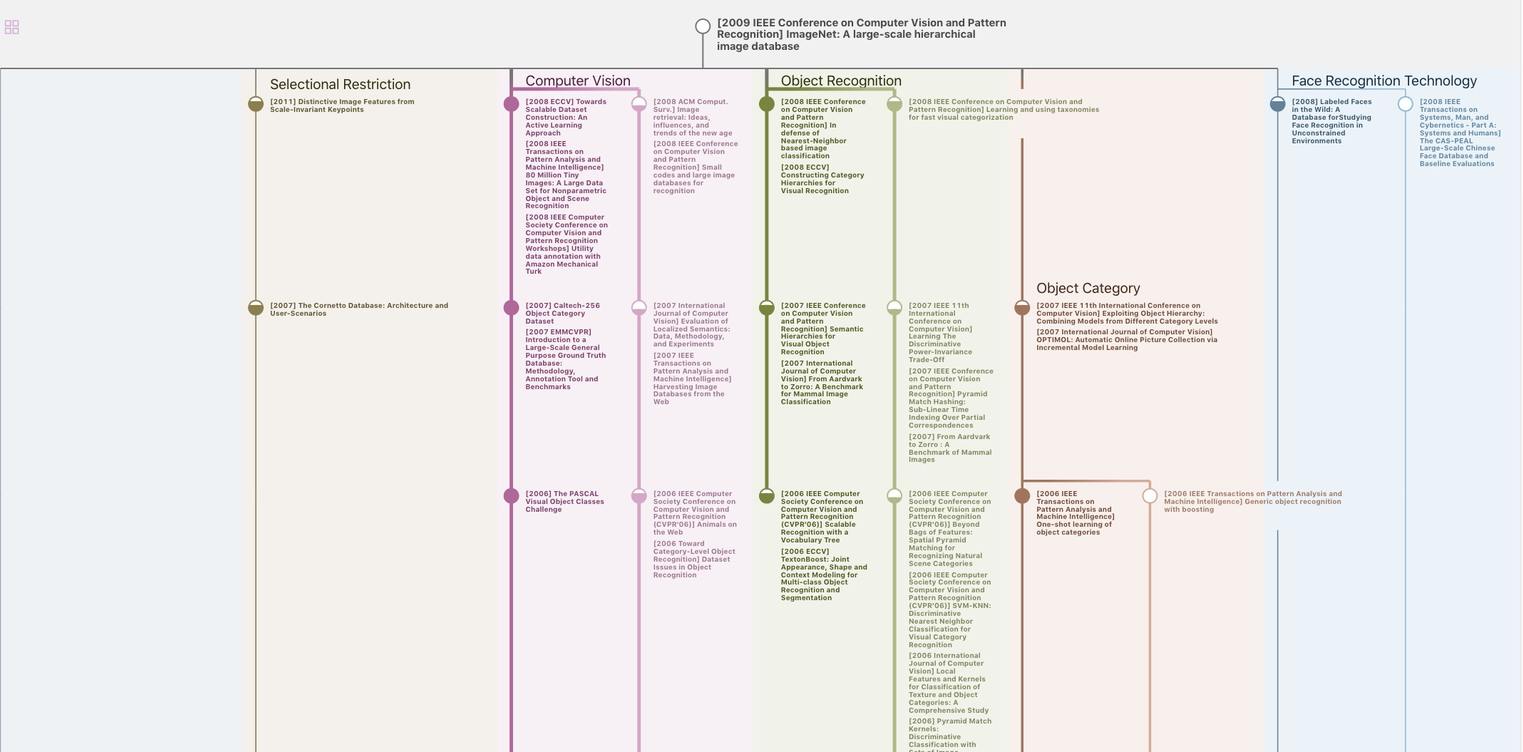
生成溯源树,研究论文发展脉络
Chat Paper
正在生成论文摘要