Forecasting heating and cooling loads in residential buildings using machine learning: a comparative study of techniques and influential indicators
Asian Journal of Civil Engineering(2023)
摘要
Residential buildings are a significant source of energy consumption and greenhouse gas emissions, making it crucial to accurately predict their energy demand for reducing their environmental impact. In this study, machine-learning techniques such as linear regression, decision tree classification, logistic regression, and neural networks were applied to forecast the heating and cooling loads of 12 different building types using their area and height attributes. The correlation coefficient was utilized to assign weights to the predictors in linear regression, and the models’ performance was evaluated using metrics such as equations of R2, MAE, and RMSE. The decision tree technique demonstrated the highest accuracy of 98.96% and 93.24% for predicting heating and cooling loads, respectively, among the classification methods. Notably, the cooling load prediction was more accurate than the heating load prediction. The height and area of the roof and floor, along with the relative compactness of the building, were identified as the most influential factors in the heating and cooling loads. These findings have significant implications for optimizing energy efficiency in residential buildings and mitigating their impact on climate change.
更多查看译文
关键词
machine learning,forecasting,residential buildings
AI 理解论文
溯源树
样例
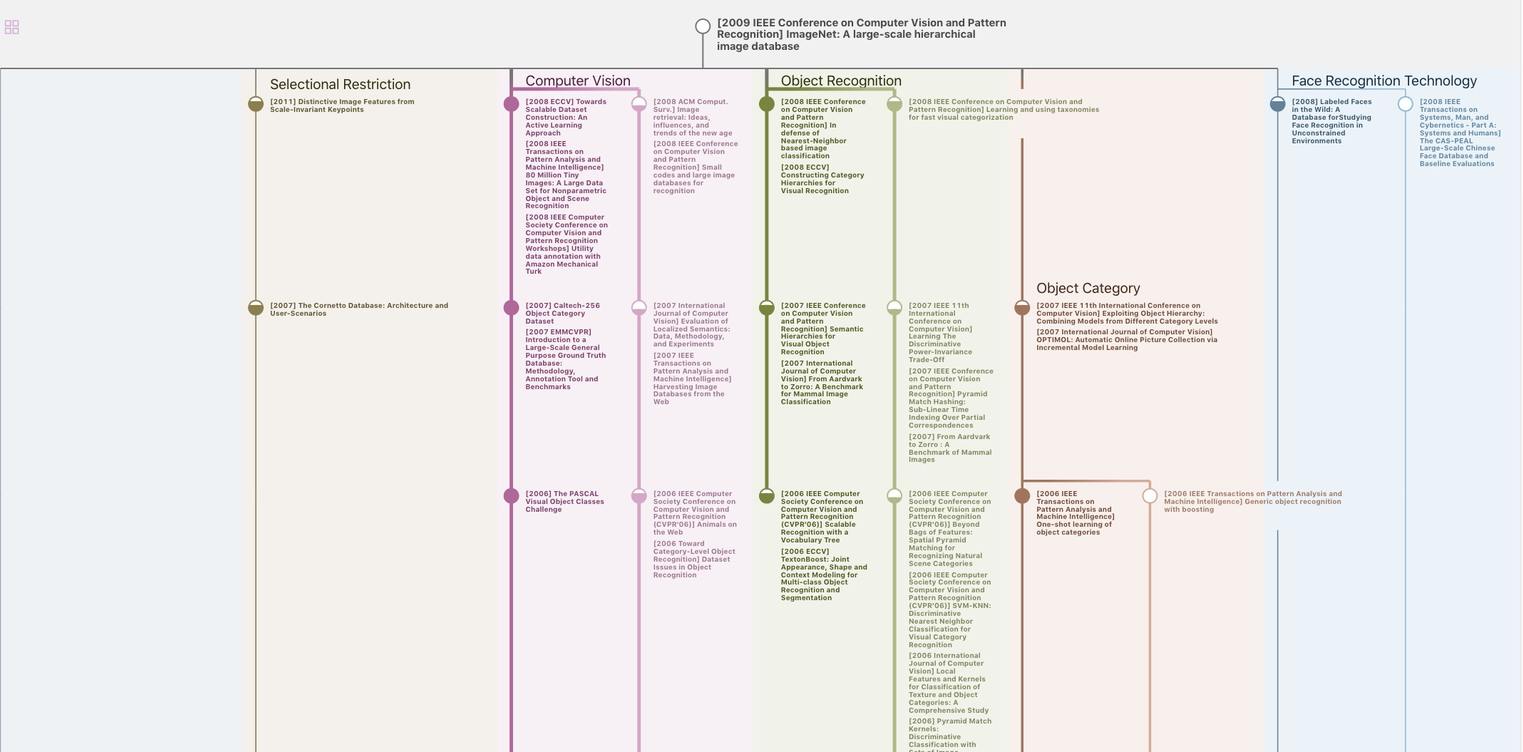
生成溯源树,研究论文发展脉络
Chat Paper
正在生成论文摘要