Sustainable ℓ2 -regularized actor-critic based on recursive least-squares temporal difference learning
2017 IEEE International Conference on Systems, Man, and Cybernetics (SMC)(2017)
摘要
Least-squares temporal difference learning (LSTD) has been used mainly for improving the data efficiency of the critic in actor-critic (AC). However, convergence analysis of the resulted algorithms is difficult when policy is changing. In this paper, a new AC method is proposed based on LSTD under discount criterion. The method comprises two components as the contribution: (1) LSTD works in an on-policy way to achieve a good convergence property of AC. (2) A sustainable ℓ
2
-regularization version of recursive LSTD, which is termed as RRLSTD, is proposed to solve the ℓ
2
-regularization problem of the critic in AC. To reduce the computation complexity of RRLSTD, we propose a fast version that is termed as FRRLSTD. Simulation results show that RRLSTD/FRRLSTD-based AC methods have better learning efficiency and faster convergence rate than conventional AC methods.
更多查看译文
关键词
ℓ2-regularization,actor-critic,least-squares temporal difference learning,value function approximation,reinforcement learning
AI 理解论文
溯源树
样例
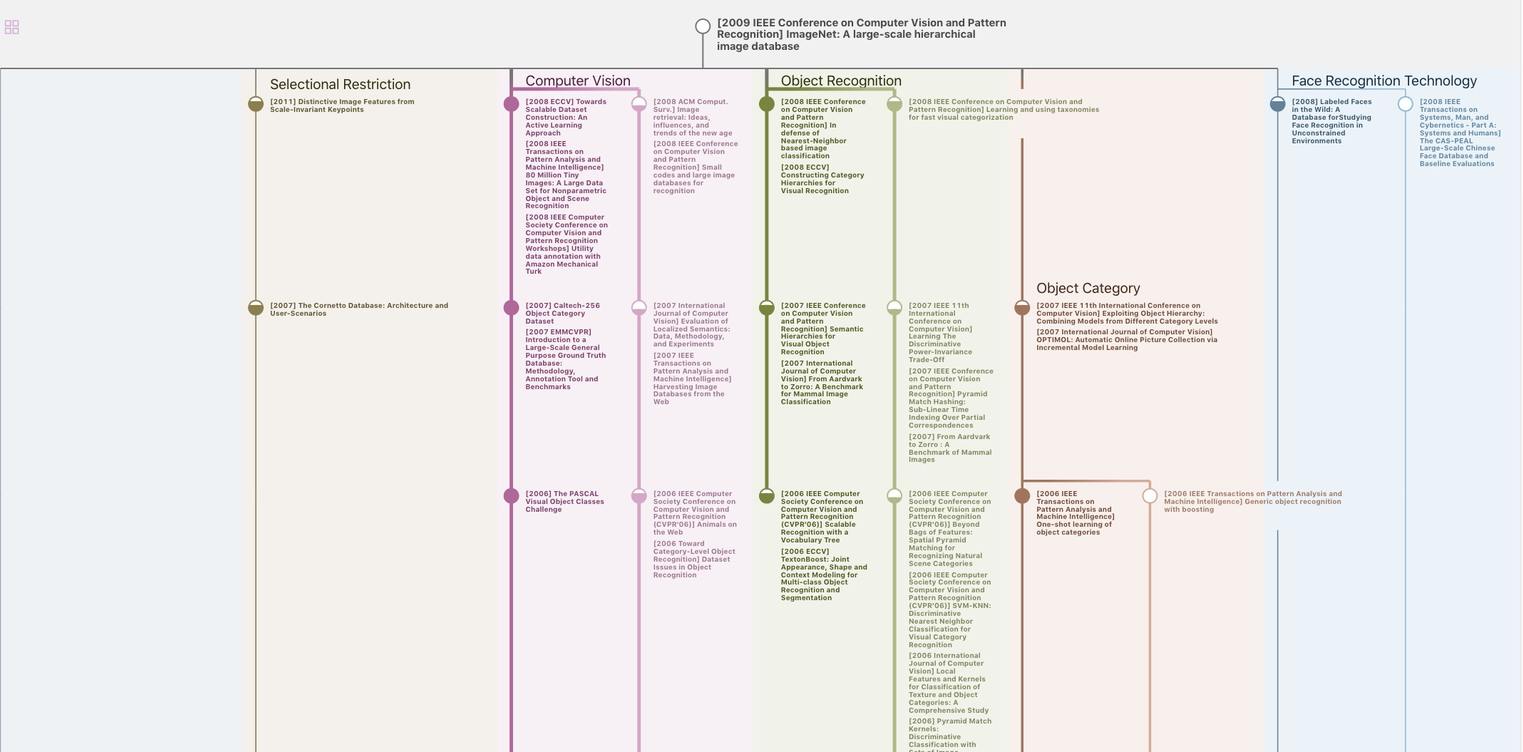
生成溯源树,研究论文发展脉络
Chat Paper
正在生成论文摘要