Path Smoothing Through Learning to Optimize
2023 42nd Chinese Control Conference (CCC)(2023)
摘要
In this paper, we propose a novel method based on the learning-to-optimize (L2O) framework for path smoothing. We adopt a hierarchical path-planning framework with a global collision-free guiding path generated beforehand. This path is then broken into segments to be fed into the L2O framework. We present a new cost function in L2O to indicate the smoothness of the expected path, which explicitly constrains the changes between successive path segments. The optimizer in the L20 framework takes as the input the gradient of the designed cost function and the hidden state of the last time step. It incrementally updates the path vertex coordinates and iteratively optimizes the path. The developed framework is verified through simulation experiments. It is observed that with the dedicated designed cost function, the Long Short-Term Memory (LSTM) unit in the developed L2O framework relies more on the input guiding path and releases the dependence on analyzing the environment, which further prompts the generation of the optimal path.
更多查看译文
关键词
Path Smoothing,Learning to Optimize,Recurrent Neural Network
AI 理解论文
溯源树
样例
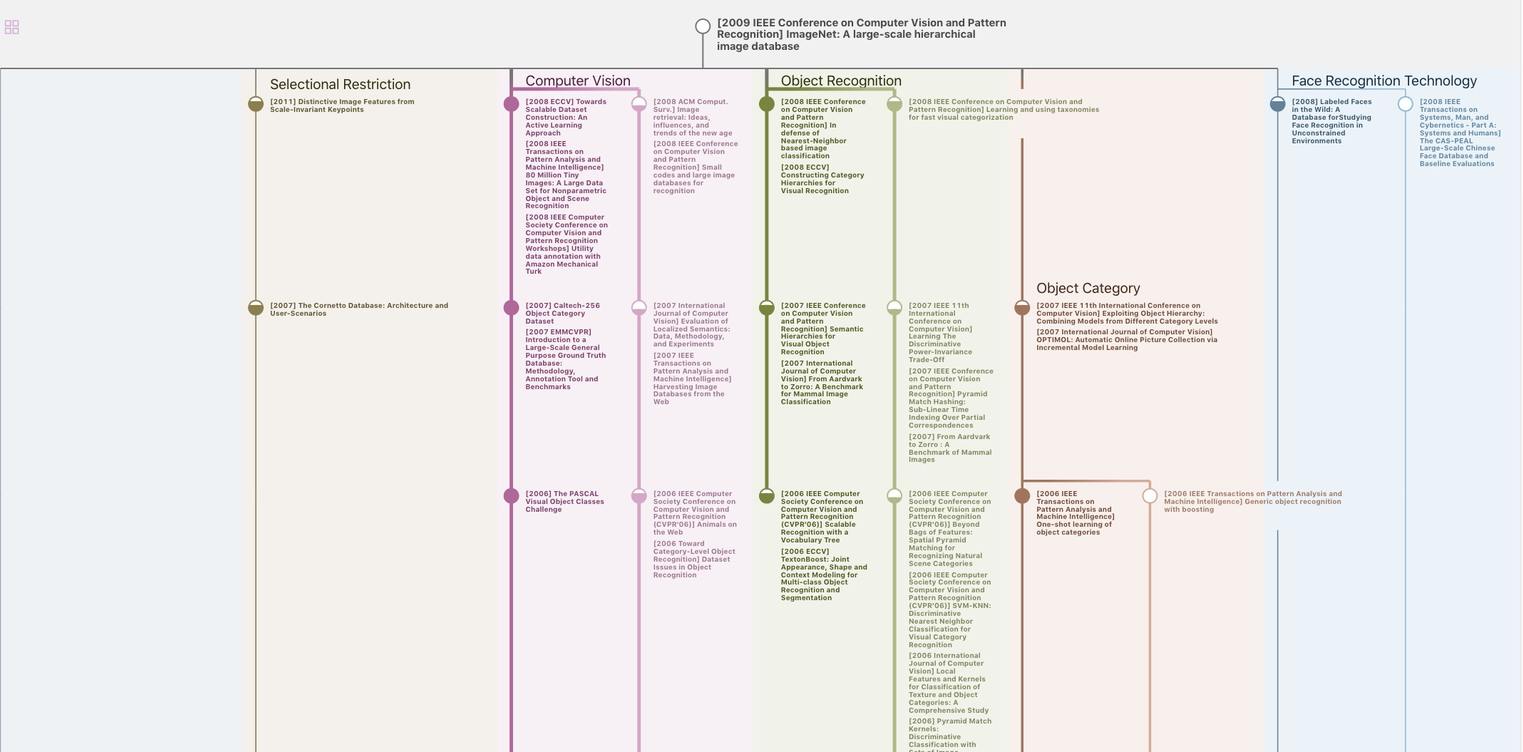
生成溯源树,研究论文发展脉络
Chat Paper
正在生成论文摘要