Robust Repetitive Learning Control for a Class of Nonlinear Systems
2023 42nd Chinese Control Conference (CCC)(2023)
Abstract
This paper proposes a framework for designing a repetitive learning controller (RLC) to track a periodic reference signal for a class of nonlinear systems in the presence of periodic uncertainties. The design process consists of several decoupled steps. First, a tracking controller is presented to track the reference without considering the uncertainties. Second, a robust controller is developed for linearized model around the equilibrium to handle uncertainties without taking into account the tracking performance. Third, by using a complementary robust control structure, a tuning parameter is introduced to balance the tracking performance and robustness. If the optimal parameter exists, by tuning this parameter with a data-driven optimization technique over repetition, the optimal performance can be obtained. Under the RLC setting, the existence of the optimal parameter makes this framework directly applicable to RLC. In this work, a well-known nonlinear output regulation is selected to track the periodic reference signal, while
$\mathcal{H}_{\infty}$
is selected as the robust controller, and extremum seeking is picked as a data-driven optimization, to demonstrate our idea. The effectiveness and performance of our proposed control framework are analyzed and verified via the Furuta inverted pendulum system.
MoreTranslated text
Key words
Nonlinear systems,Repetitive learning control,Tracking control,Robust control,Data-driven optimization
AI Read Science
Must-Reading Tree
Example
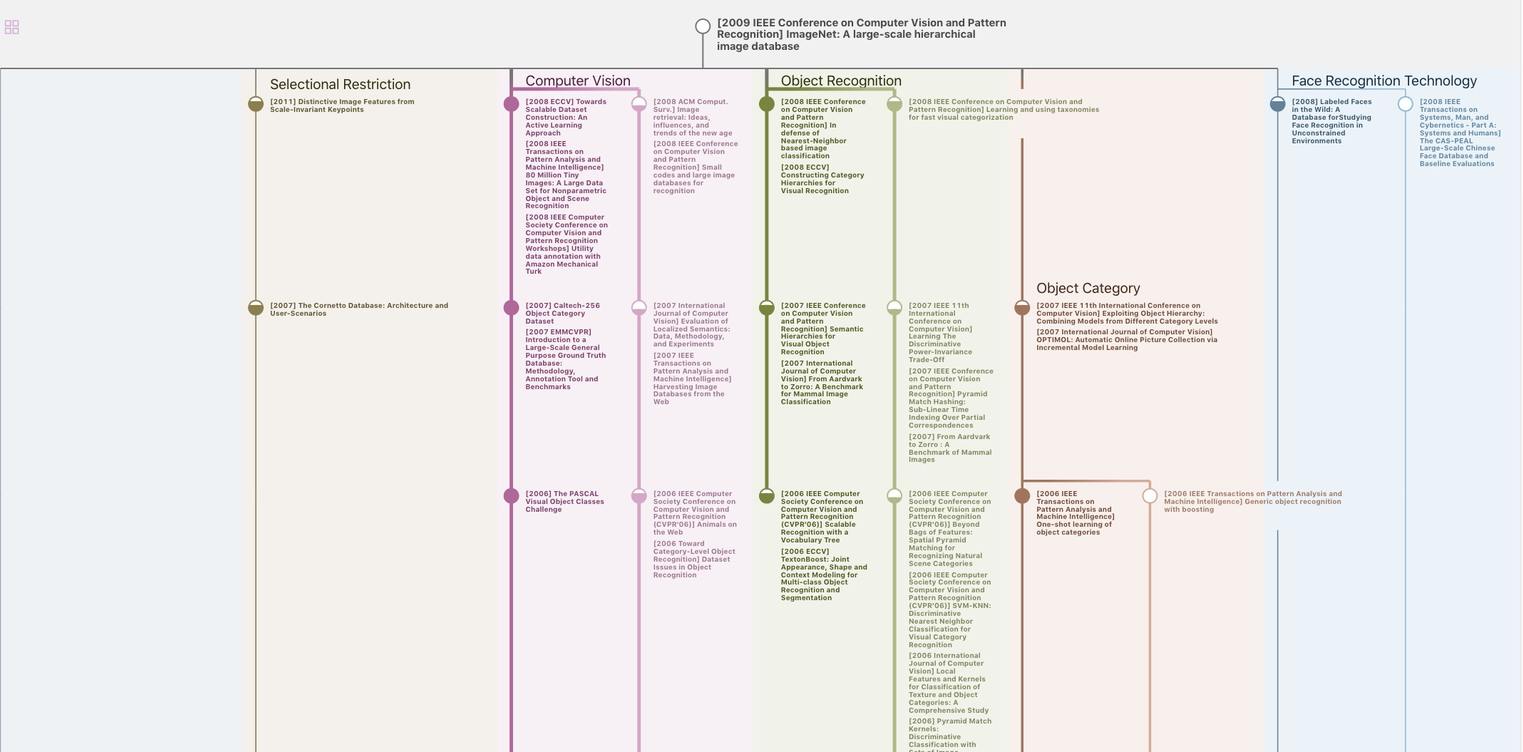
Generate MRT to find the research sequence of this paper
Chat Paper
Summary is being generated by the instructions you defined