Data-Driven Defenses Against Adversarial Attacks for Autonomous Vehicles
2023 IEEE International Automated Vehicle Validation Conference (IAVVC)(2023)
摘要
Deep Learning (DL) is a growing technological frontier supporting many autonomous driving applications. Past decade with the growth in deep learning concepts and models, we also witnessed a growth in cyber-security concerns related to these models. Adversarial attacks are well-known attacks on these models where slight noise/ perturbations are introduced with the input image, forcing the DL models to misclassify with high confidence. These are significant threats and concerns when using DL models for real-world autonomous vehicle applications. However, various defensive mechanisms have been suggested to improve the resiliency of these models. In this study, we worked on the LISA dataset and tested with four transfer learning models, EfficientNet, SqueezeNet, ResNet-18 and Inception-V3, with accuracies of 95%, 95%, 94% and 97%, respectively. Based on the severity of adversarial attacks, we selected three attacks, Fast Gradient Sign Method (FGSM), Basic Iterative Method (BIM) and Project Gradient Descent (PGD) attacks ranging from less intensive to highly intensive adversarial attacks. When subjected to FGSM, BIM, and PGD attacks, we discovered that the model performance for EfficientNet decreased to 92%, 38%, and 50%, that for SqueezeNet decreased to 87%, 53%, and 59%, that for ResNet-18 decreased to 95%, 53%, and 49%, and that for Inception-V3decreased to 93%, 45%, and 56%. respectively. The combination of Total Variance Minimization and Spatial Smoothing was determined to be the most effective defensive method combination to increase performance during adversarial attacks. Despite only slight improvements in the performance of FGSM attacks, all models significantly outperformed during BIM and PGD attacks, with performance accuracy of 83% and higher.
更多查看译文
关键词
Adversarial Attacks,Autonomous Vehicle,Defense Strategies,LISA Dataset,Traffic Sign Classification
AI 理解论文
溯源树
样例
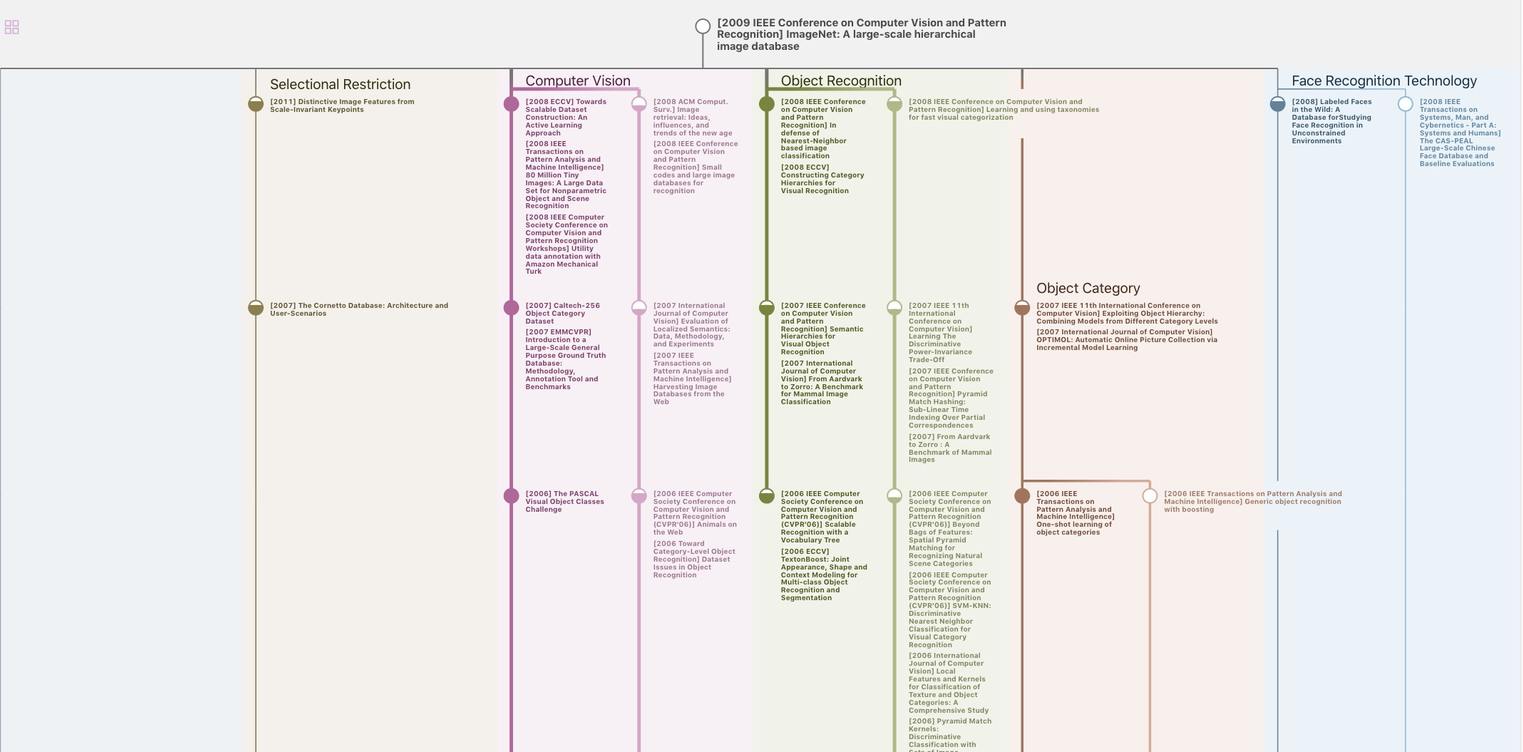
生成溯源树,研究论文发展脉络
Chat Paper
正在生成论文摘要