Spatial and Temporal Water Quality Data Prediction of Transboundary Watershed Using Multiview Neural Network Coupling
IEEE TRANSACTIONS ON GEOSCIENCE AND REMOTE SENSING(2023)
摘要
There exists an inherent contradiction between the continuous accumulation of environmental-geographical spatiotemporal data and the ongoing refinement of analysis granularity. Algorithms aimed at mining spatiotemporal water quality data in river networks are confronted with constraints posed by statistical factors, including sparse distribution, heterogeneity, and spatiotemporal autocorrelation features. Consequently, prevailing machine-learning methods encounter challenges when attempting to encapsulate the evolving characteristics of nonstationarity over time and the cyclic evolution patterns of intricate watershed geographical processes. These challenges serve as notable impediments to achieving precise and resilient predictions for watershed water quality. In consideration of these circumstances, we propose a predictive framework built upon the fusion of deep-learning and multiview semisupervised learning. This framework involves the modeling of correlations between time series feature factors and water quality, the discernment of relationships between the spatial-physical structure and temporal features of river networks, and the identification of similar features related to the environmental context across distinct river segments. In addition, we introduce coefficients for adjusting feature weights to mitigate the adverse impact stemming from noise or data sparsity in low-quality views. We establish a feature classifier that upholds interview consistency and global spatiotemporal correlations, leveraging complementary knowledge from different feature perspectives to capture long-range spatiotemporal dependencies among river segments. This, in turn, enhances cross-watershed global prediction accuracy. Through comprehensive performance comparisons with eight competing algorithms, the results underscore that the proposed approach demonstrates enhanced robustness and superiority, particularly within intricate and complex river network systems.
更多查看译文
关键词
Spatiotemporal phenomena,Rivers,Water quality,Watersheds,Predictive models,Adaptation models,Water pollution,Interbasin water diversion,international rivers,multiview learning,spatiotemporal autocorrelation,spatiotemporal prediction,water quality prediction
AI 理解论文
溯源树
样例
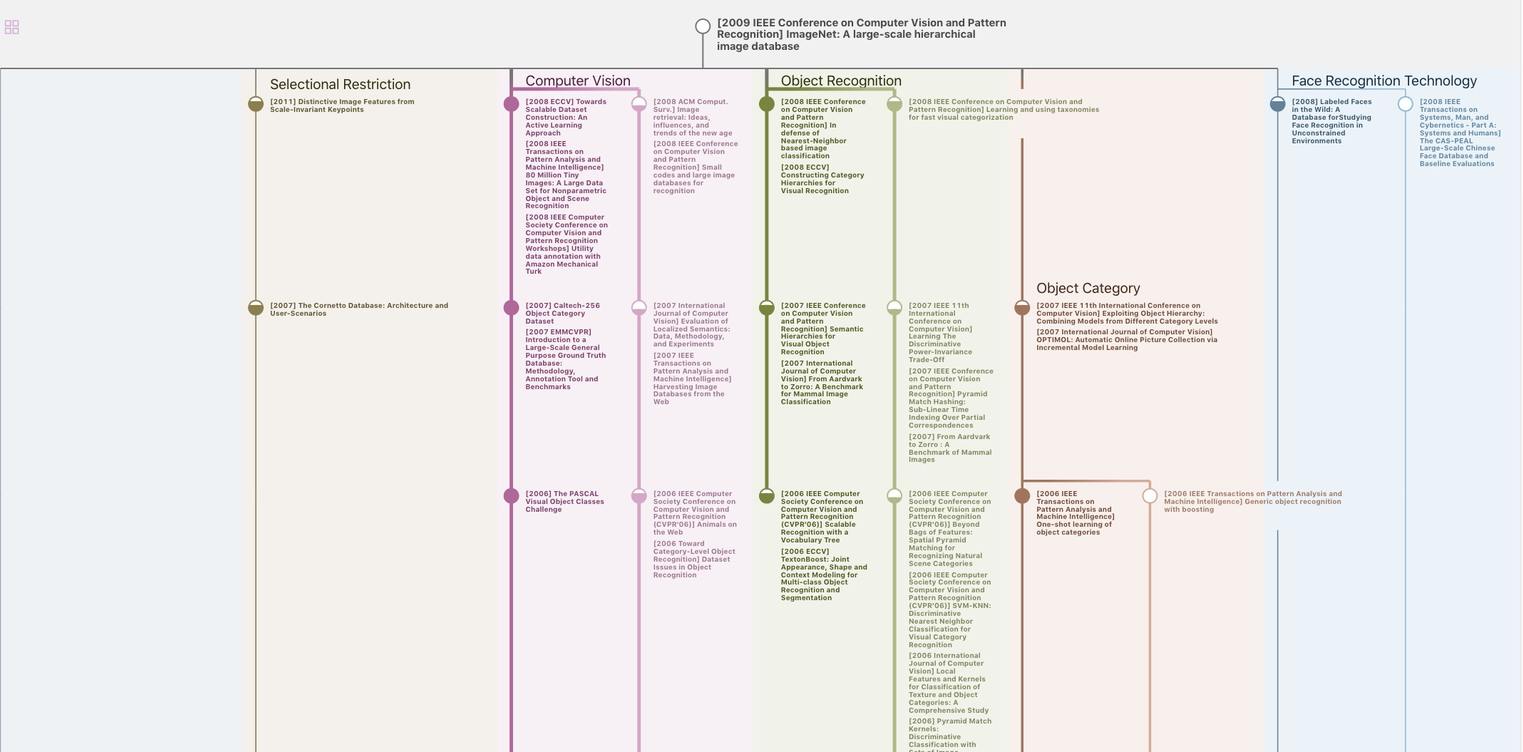
生成溯源树,研究论文发展脉络
Chat Paper
正在生成论文摘要