Better Semantic Segmentation For 3D Printing Concrete Surface Pore Detection
2023 42nd Chinese Control Conference (CCC)(2023)
摘要
As the most common defect of 3D printing concrete components, pores will seriously affect the mechanical properties of 3D printing concrete. In recent years, deep learning methods have been widely used in defect recognition tasks because of their high efficiency, accuracy, and autonomous learning of data features. The DeepLabv3+ segmentation model has achieved outstanding image segmentation results. This is due to its innovative Encoder-Decoder structure, which incorporates high-level semantic information and shallow target features. Additionally, it employs the deep separation convolution technique. However, in the process of coding module training, the spatial dimension of input data is gradually reduced, resulting in the loss of useful information, which brings certain limitations to the recognition of small targets with different scales. This paper presents a novel approach to identifying concrete pores using the deep learning method. Specifically, we propose a semantic segmentation method based on an improved version of DeepLabv3+. The proposed method incorporates a pore feature layer combination module and CBAM module to expand the receptive field and obtain more accurate pore feature maps. In order to evaluate the effectiveness of the improved algorithm, we utilize the pore images generated by the laboratory as the original dataset. Using the same dataset, we compared these images with current representative image segmentation models, such as Unet and DeepLab V3+. The results indicate that the recall rate of this algorithm is 2.55% higher than Unet and 2.15% higher than DeepLabv3+. The mean average intersection ratio reached 88.30%, and the average pixel accuracy reached 92.84%. The algorithm is more suitable for 3D printing concrete pore segmentation tasks and has practical engineering application significance.
更多查看译文
关键词
3D printing concrete,Deeplabv3+,Pore segmentation,Semantic segmentation
AI 理解论文
溯源树
样例
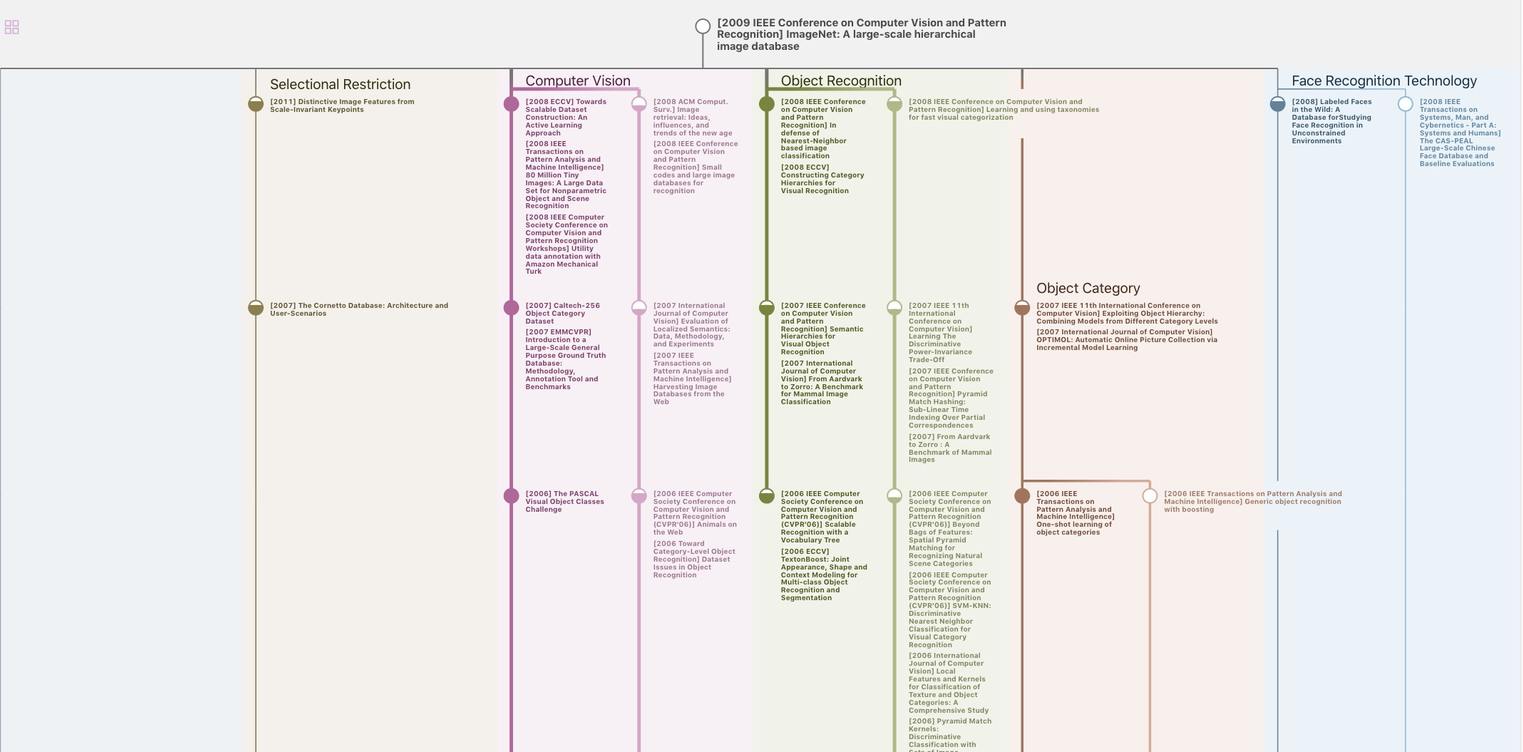
生成溯源树,研究论文发展脉络
Chat Paper
正在生成论文摘要