Domain Generalized Solar Cell Defect Segmentation Based on Shape-Aware and Multi-View Meta-Learning
2023 42nd Chinese Control Conference (CCC)(2023)
摘要
Various defects are inevitably generated in the manufacturing process of solar cells. Deep learning-based methods for defect segmentation under closed situation have achieved remarkable progress. Due to the difference of imaging condition and camera parameter under different production line, there are large differences in brightness distribution of solar cell images. The model trained under closed situation cannot achieve good performance in opened situation such as multi-production lines. In this paper, a new shape-aware and multi-view meta-learning scheme is proposed to improve the model generalization performance for single-domain solar cell defect segmentation. The scheme roots in gradient-based meta-learning. Multi-brightness view information is yielded from the generated augmented images, and explicitly simulating domain shift with virtual meta-train and meta-test during training to mitigate overfitting in single-source domain train and unstable prediction. Importantly, considering tiny and faint solar cell defects are not easily identified under different image brightness conditions, shape edge constraint loss is proposed to encourage shape compactness and shape smoothness of segmentations. Experimental results show that the proposed method has high segmentation accuracy, which outperforms the state-of-the-art methods.
更多查看译文
关键词
Solar cell,Domain generalization,Semantic segmentation,Meta-learning
AI 理解论文
溯源树
样例
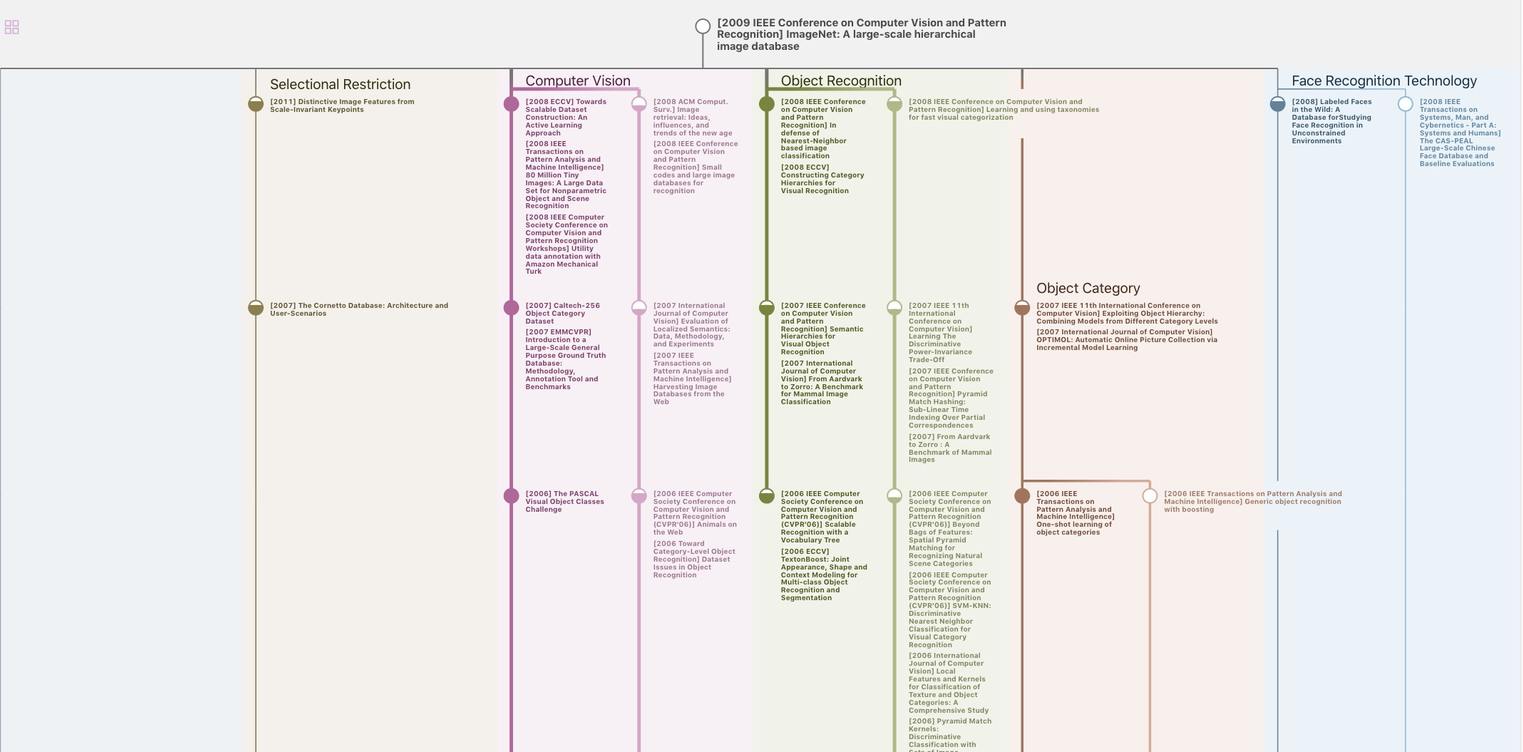
生成溯源树,研究论文发展脉络
Chat Paper
正在生成论文摘要