Stacked Dual-stream LSTM based Feature Extraction Network for Hyperspectral Image Classification
2023 42nd Chinese Control Conference (CCC)(2023)
摘要
Remote sensing technology plays a significant role in classifying and detecting on-ground objects accurately. Specifically, hyperspectral imagery outperforms other remote sensing tools in terms of its abundant spatial and spectral information. However, analyzing hyperspectral images is always hampered by their redundant spectral dimensionality. In this paper, we proposed a novel stacked dual-stream long short-term memory (LSTM) based feature extraction network (SDLSTMnet) for hyperspectral image classification. The whole network consists of two streams for spatial and spectral information, respectively. In this network, convolutional layers are absolutely removed because of their time-consuming calculating and optimizing processes. As a substitute, stacked LSTM layers are utilized to capture the spatial information within sample neighbourhoods. Notably, the latent sequential features are captured to simulate the neighbouring regions of samples. Furthermore, the sequential features along the spectral dimension are also extracted by stacked LSTM layers because these bands are continuous locally. Finally, the presented fusion module is capable to fuse and align these extracted features for classification. Multiple experiments are conducted to prove the effectiveness of the proposed SDLSTMnet. Compared with other state-of-the-art techniques, SDLSTMnet is capable to reduce the spectral dimension and increase the classification accuracies simultaneously.
更多查看译文
关键词
Deep Learning,Hyperspectral Image,Classification,Long Short-Term Memory,Remote Sensing
AI 理解论文
溯源树
样例
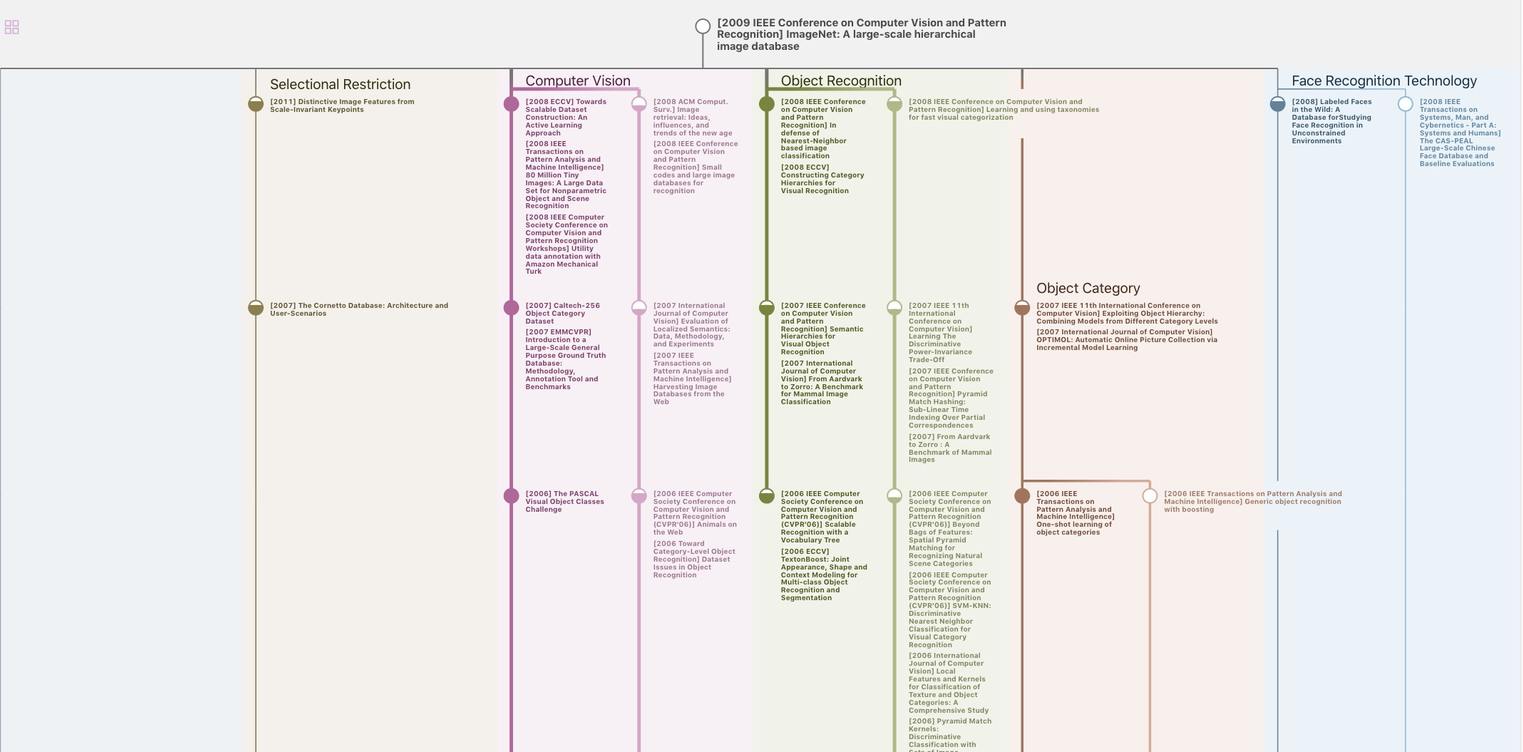
生成溯源树,研究论文发展脉络
Chat Paper
正在生成论文摘要