Modelling fractional-order moisture transport in irrigation using artificial neural networks
SeMA Journal(2023)
摘要
The paper considers the issue of choosing a mathematical model for predicting the dynamics of moisture transport in fractal-structured soils with specific restrictions that arise from the application in irrigated agriculture. In particular, our goal is to evaluate the possibilities and effectiveness of using, along with non-local fractional-differential models, the simpler classical model based on Richards equation. Fractional-order models with Caputo and distributed-order derivatives are studied with their numerical solutions obtained according to the Crank–Nicholson finite-difference scheme. On the base of the generated sets of initial-boundary value problems’ solutions, an artificial neural network was built and trained. It allows obtaining estimates of the values of classical model’s parameters which describe the input dynamics of pressures. The use of the created neural network allowed in 69% of cases to restore with an error < 20% pressure dynamics according to the classical model. In the case of two fractional-differential models, this percentage decreases to 59–62%. The parameter of the form of functional dependency between hydraulic conductivity and moisture content was a critical parameter that influences the possibility of an approximate description of the fractional-differential dynamics by the classical model. We also show that the variation of classical model’s parameters does not allow to adequately describe the process of moisture transport with significant manifestation of non-local effects. However, the developed technique can be used, in the case of its applicability for a specific observed process, to increase the speed of modelling.
更多查看译文
关键词
irrigation,artificial neural networks,neural networks,modelling,fractional-order
AI 理解论文
溯源树
样例
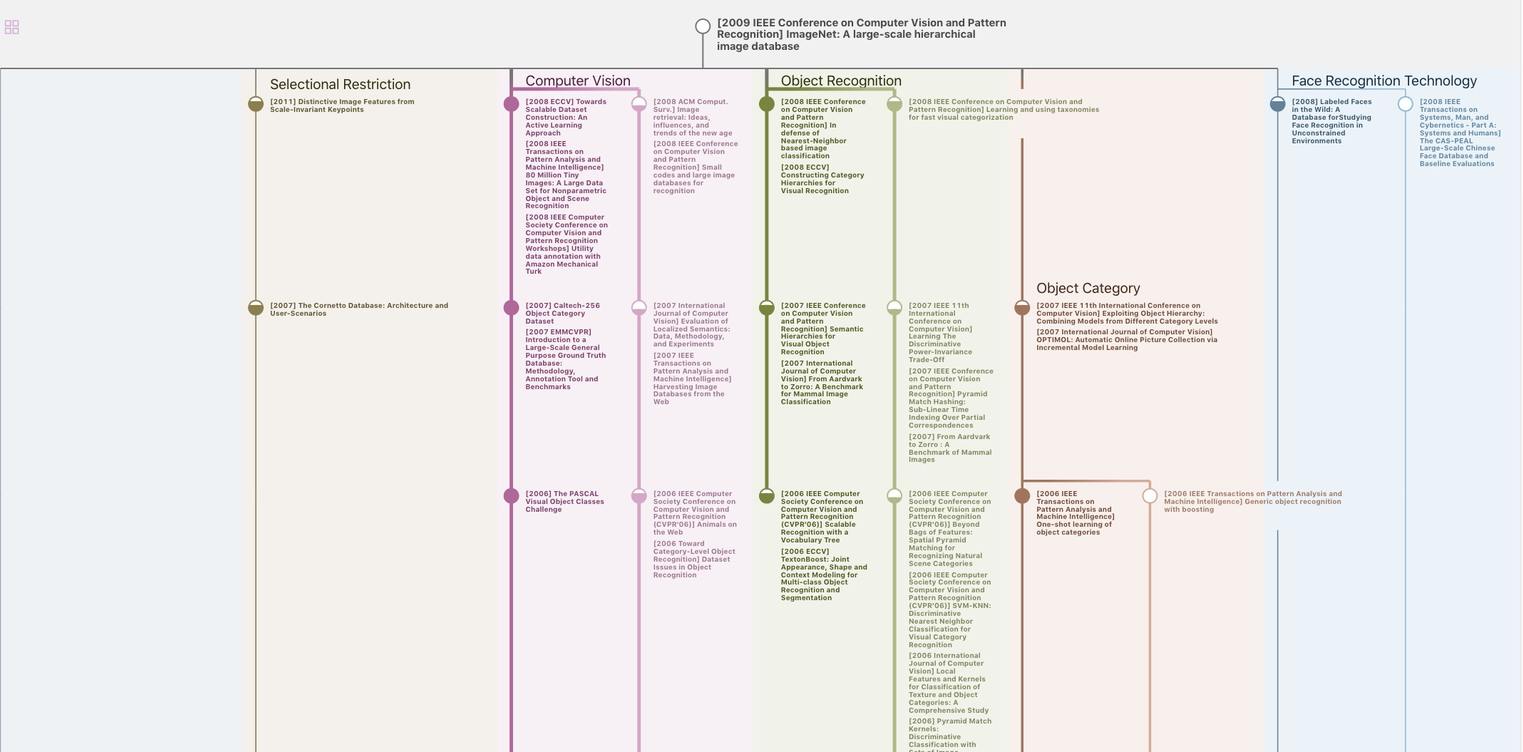
生成溯源树,研究论文发展脉络
Chat Paper
正在生成论文摘要