Enhancing Model Performance via Vertical Federated Learning for Non-Overlapping Data Utilization
2023 4th International Conference on Information Science, Parallel and Distributed Systems (ISPDS)(2023)
摘要
Collaborative training of machine learning models is essential in the era of big data. Federated learning ensures secure data sharing among multiple parties without compromising privacy. It includes various approaches like horizontal federated learning, vertical federated learning, and federated transfer learning. Vertical federated learning enables participants to train on different feature spaces while sharing sample labels. However, existing vertical federated learning schemes rely on participants having sufficient overlapping samples, limiting their effectiveness in scenarios with limited overlapping data. This poses challenges, particularly in domains like the medical industry where collecting enough overlapping samples is difficult. Traditional approaches fail to utilize the non-overlapping portion of the sample data, resulting in suboptimal model performance due to insufficient training data. To address this issue, we propose a novel scheme for training neural network models within the vertical federated learning framework using non-overlapping samples. Our scheme leverages fuzzy prediction to handle non-overlapping samples, improving data utilization and enhancing model performance. Crucially, our approach ensures participants' data privacy by not requiring the sharing of original data or model parameters. Experimental results validate the efficacy and efficiency of our proposed scheme.
更多查看译文
关键词
Vertical federated learning,Non-overlapping data,Neural network,Data privacy
AI 理解论文
溯源树
样例
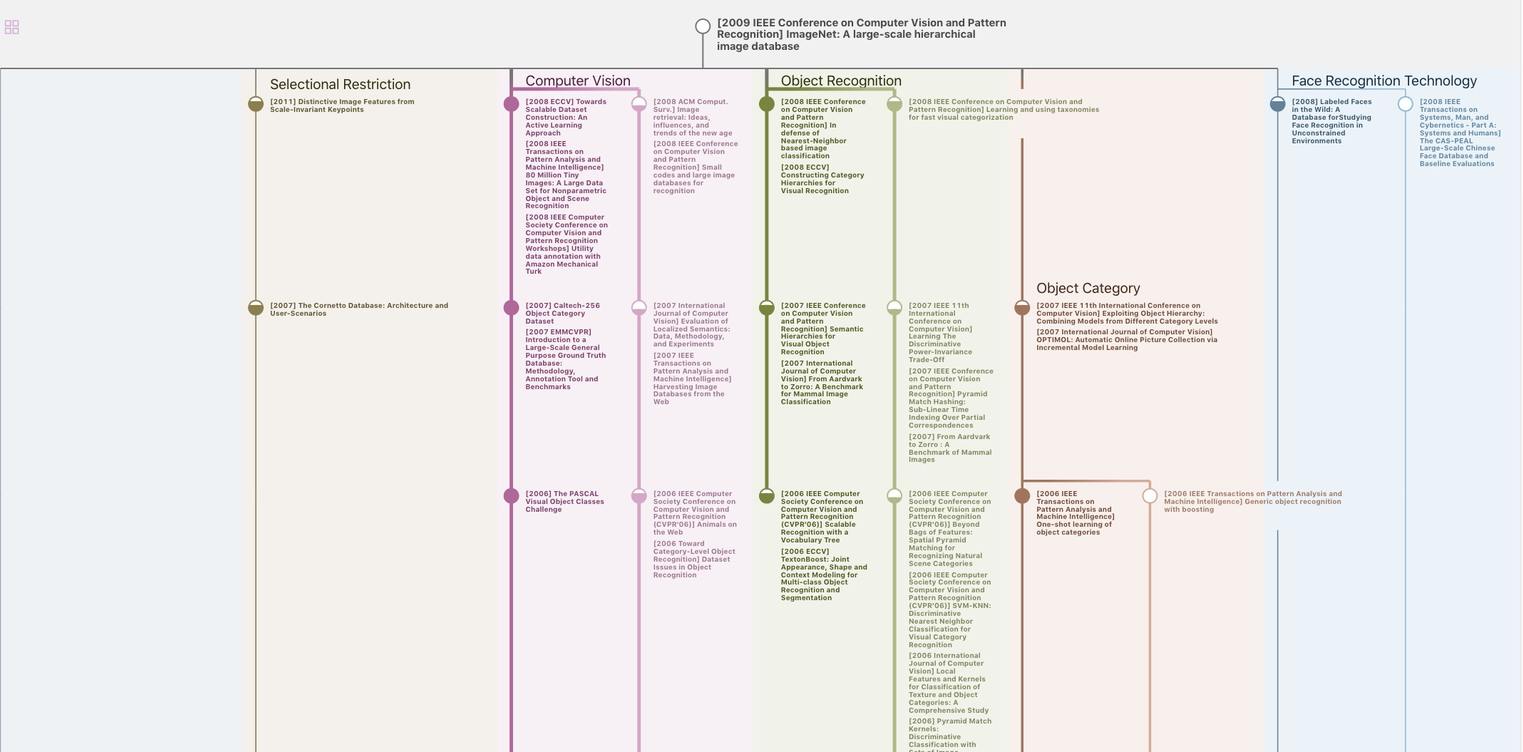
生成溯源树,研究论文发展脉络
Chat Paper
正在生成论文摘要