Consistency Theory for the General Nonparametric Classification Method
PSYCHOMETRIKA(2019)
摘要
Parametric likelihood estimation is the prevailing method for fitting cognitive diagnosis models—also called diagnostic classification models (DCMs). Nonparametric concepts and methods that do not rely on a parametric statistical model have been proposed for cognitive diagnosis. These methods are particularly useful when sample sizes are small. The general nonparametric classification (GNPC) method for assigning examinees to proficiency classes can accommodate assessment data conforming to any diagnostic classification model that describes the probability of a correct item response as an increasing function of the number of required attributes mastered by an examinee (known as the “monotonicity assumption”). Hence, the GNPC method can be used with any model that can be represented as a general DCM. However, the statistical properties of the estimator of examinees’ proficiency class are currently unknown. In this article, the consistency theory of the GNPC proficiency-class estimator is developed and its statistical consistency is proven.
更多查看译文
关键词
cognitive diagnosis,Q-matrix,DINA model,DINO model,general DCM,G-DINA model,nonparametric classification,general nonparametric classification method
AI 理解论文
溯源树
样例
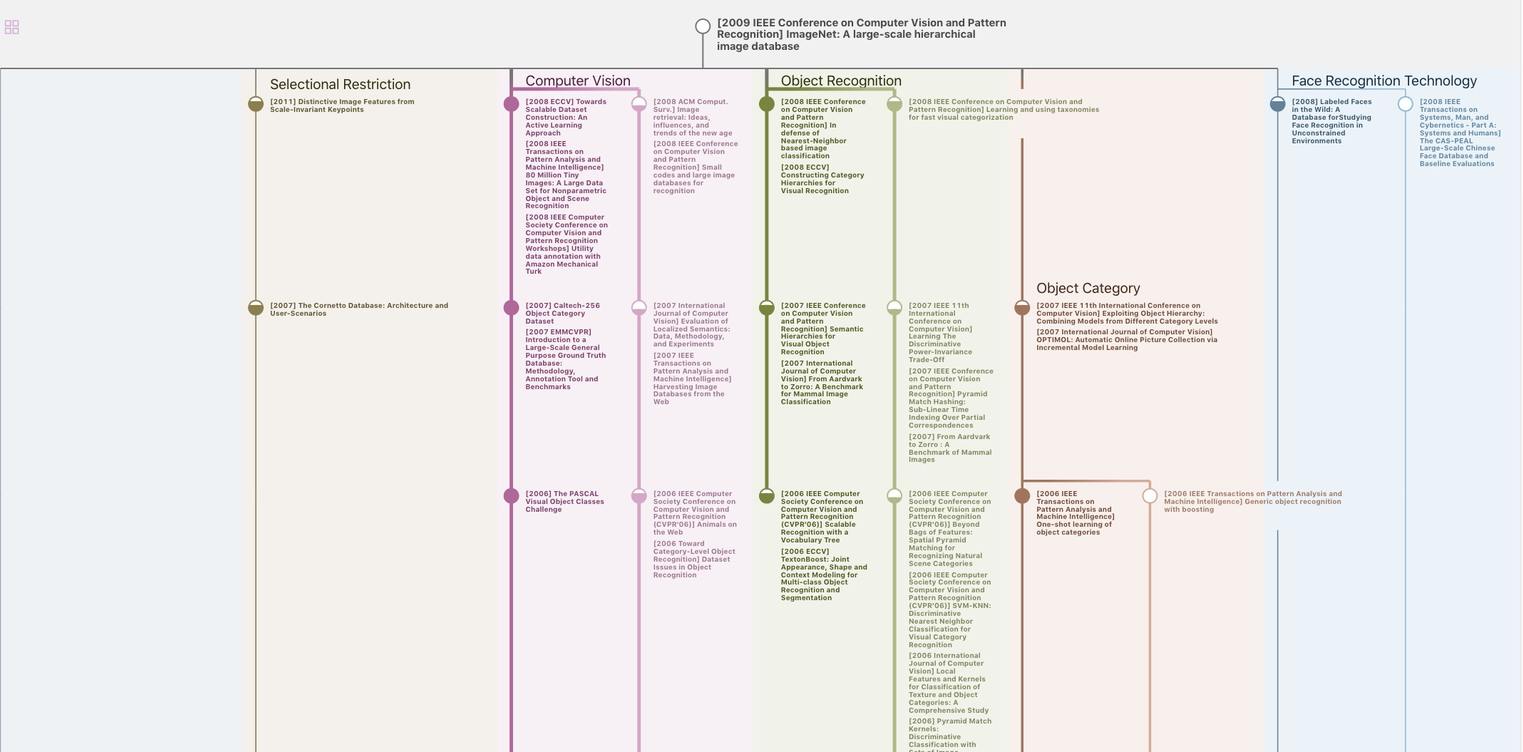
生成溯源树,研究论文发展脉络
Chat Paper
正在生成论文摘要