An AdaBoost-based algorithm to detect hospital-acquired pressure injury in the presence of conflicting annotations
COMPUTERS IN BIOLOGY AND MEDICINE(2024)
摘要
Hospital-acquired pressure injury is one of the most harmful events in clinical settings. Patients who do not receive early prevention and treatment can experience a significant financial burden and physical trauma. Several hospital-acquired pressure injury prediction algorithms have been developed to tackle this problem, but these models assume a consensus, gold-standard label (i.e., presence of pressure injury or not) is present for all training data. Existing definitions for identifying hospital-acquired pressure injuries are inconsistent due to the lack of high-quality documentation surrounding pressure injuries. To address this issue, we propose in this paper an ensemble-based algorithm that leverages truth inference methods to resolve label inconsistencies between various case definitions and the level of disagreements in annotations. Application of our method to MIMIC-III, a publicly available intensive care unit dataset, gives empirical results that illustrate the promise of learning a prediction model using truth inference-based labels and observed conflict among annotators.
更多查看译文
关键词
Clinical notes,Ensemble,Hospital-acquired pressure injury,Machine learning
AI 理解论文
溯源树
样例
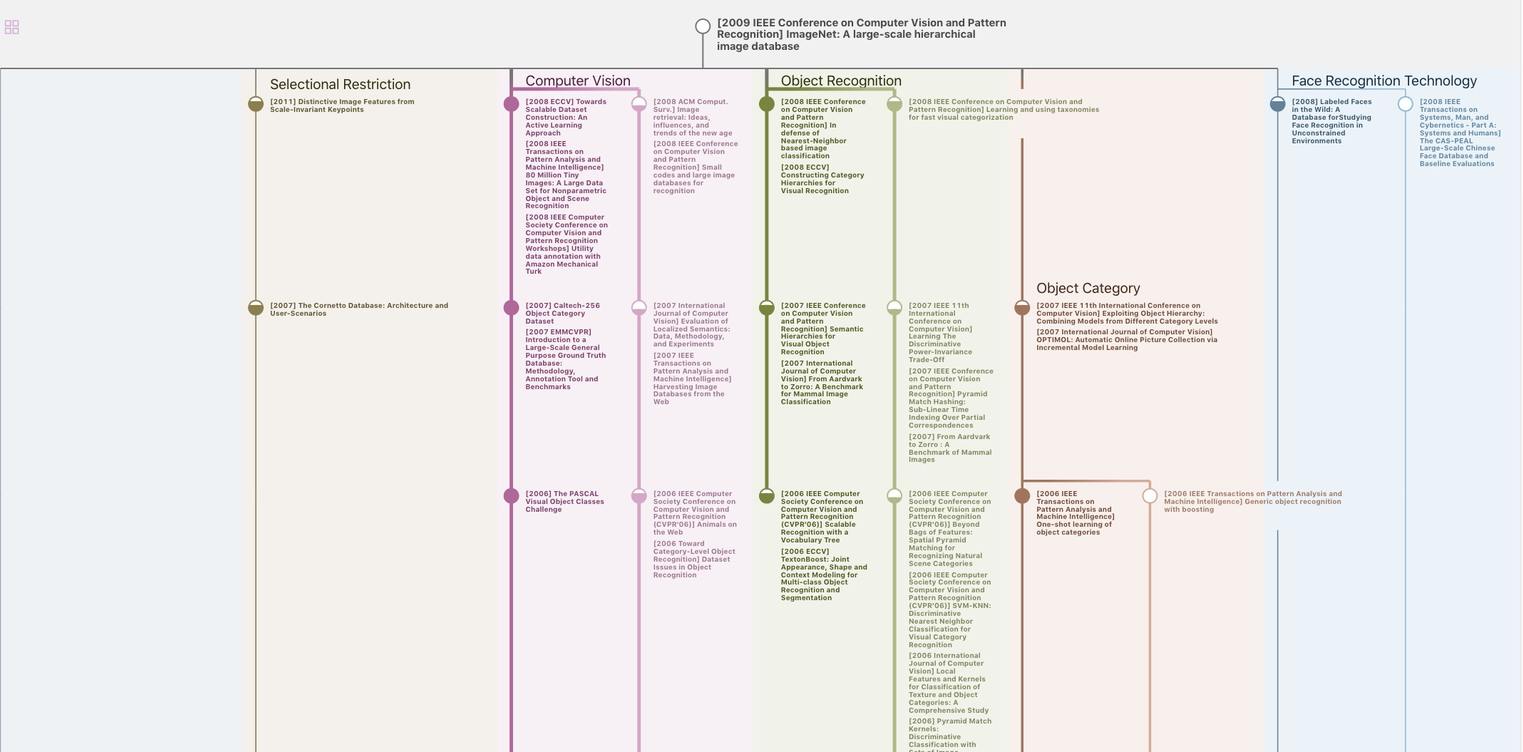
生成溯源树,研究论文发展脉络
Chat Paper
正在生成论文摘要