Learning Dynamic Scene-Conditioned 3D Object Detectors
IEEE TRANSACTIONS ON PATTERN ANALYSIS AND MACHINE INTELLIGENCE(2024)
摘要
In this paper, we propose a dynamic 3D object detector named HyperDet3D, which is adaptively adjusted based on the hyper scene-level knowledge on the fly. Existing methods strive for object-level representations of local elements and their relations without scene-level priors, which suffer from ambiguity between similarly-structured objects only based on the understanding of individual points and object candidates. Instead, we design scene-conditioned hypernetworks to simultaneously learn scene-agnostic embeddings to exploit sharable abstracts from various 3D scenes, and scene-specific knowledge which adapts the 3D detector to the given scene at test time. As a result, the lower-level ambiguity in object representations can be addressed by hierarchical context in scene priors. However, since the upstream hypernetwork in HyperDet3D takes raw scenes as input which contain noises and redundancy, it leads to sub-optimal parameters produced for the 3D detector simply under the constraint of downstream detection losses. Based on the fact that the downstream 3D detection task can be factorized into object-level semantic classification and bounding box regression, we furtherly propose HyperFormer3D by correspondingly designing their scene-level prior tasks in upstream hypernetworks, namely Semantic Occurrence and Objectness Localization. To this end, we design a transformer-based hypernetwork that translates the task-oriented scene priors into parameters of the downstream detector, which refrains from noises and redundancy of the scenes. Extensive experimental results on the ScanNet, SUN RGB-D and MatterPort3D datasets demonstrate the effectiveness of the proposed methods.
更多查看译文
关键词
3D object detection,dynamic hypernetwork,point cloud,prior knowledge,scene-conditioned learning
AI 理解论文
溯源树
样例
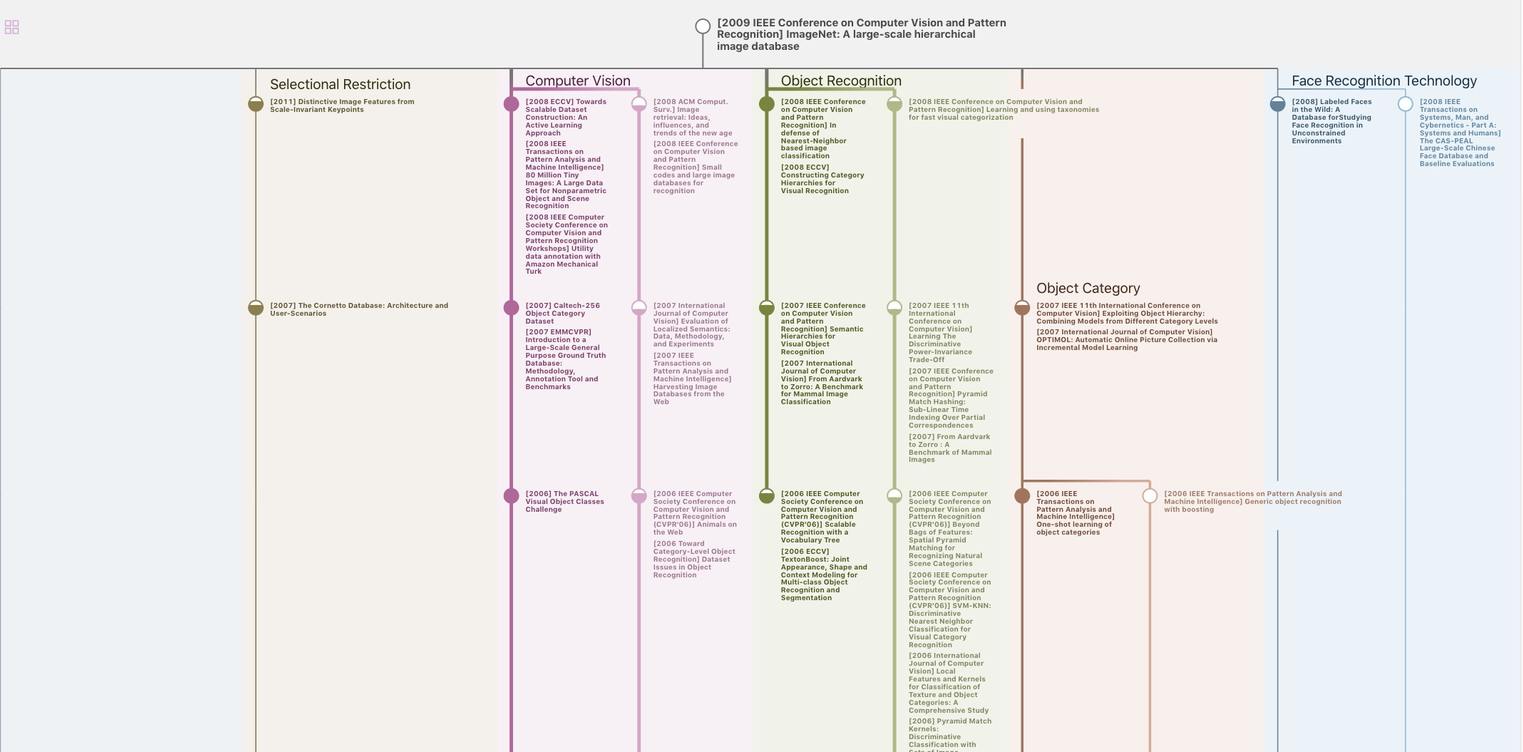
生成溯源树,研究论文发展脉络
Chat Paper
正在生成论文摘要