Quantitative Modeling of Stemness in Single-Cell RNA Sequencing Data: A Nonlinear One-Class Support Vector Machine Method
JOURNAL OF COMPUTATIONAL BIOLOGY(2024)
摘要
Intratumoral heterogeneity and the presence of cancer stem cells are challenging issues in cancer therapy. An appropriate quantification of the stemness of individual cells for assessing the potential for self-renewal and differentiation from the cell of origin can define a measurement for quantifying different cell states, which is important in understanding the dynamics of cancer evolution, and might further provide possible targeted therapies aimed at tumor stem cells. Nevertheless, it is usually difficult to quantify the stemness of a cell based on molecular information associated with the cell. In this study, we proposed a stemness definition method with one-class Hadamard kernel support vector machine (OCHSVM) based on single-cell RNA sequencing (scRNA-seq) data. Applications of the proposed OCHSVM stemness are assessed by various data sets, including preimplantation embryo cells, induced pluripotent stem cells, or tumor cells. We further compared the OCHSVM model with state-of-the-art methods CytoTRACE, one-class logistic regression, or one-class SVM methods with different kernels. The computational results demonstrate that the OCHSVM method is more suitable for stemness identification using scRNA-seq data.
更多查看译文
关键词
machine learning,nonlinear SVM,single-cell RNA-sequencing,stemness quantification
AI 理解论文
溯源树
样例
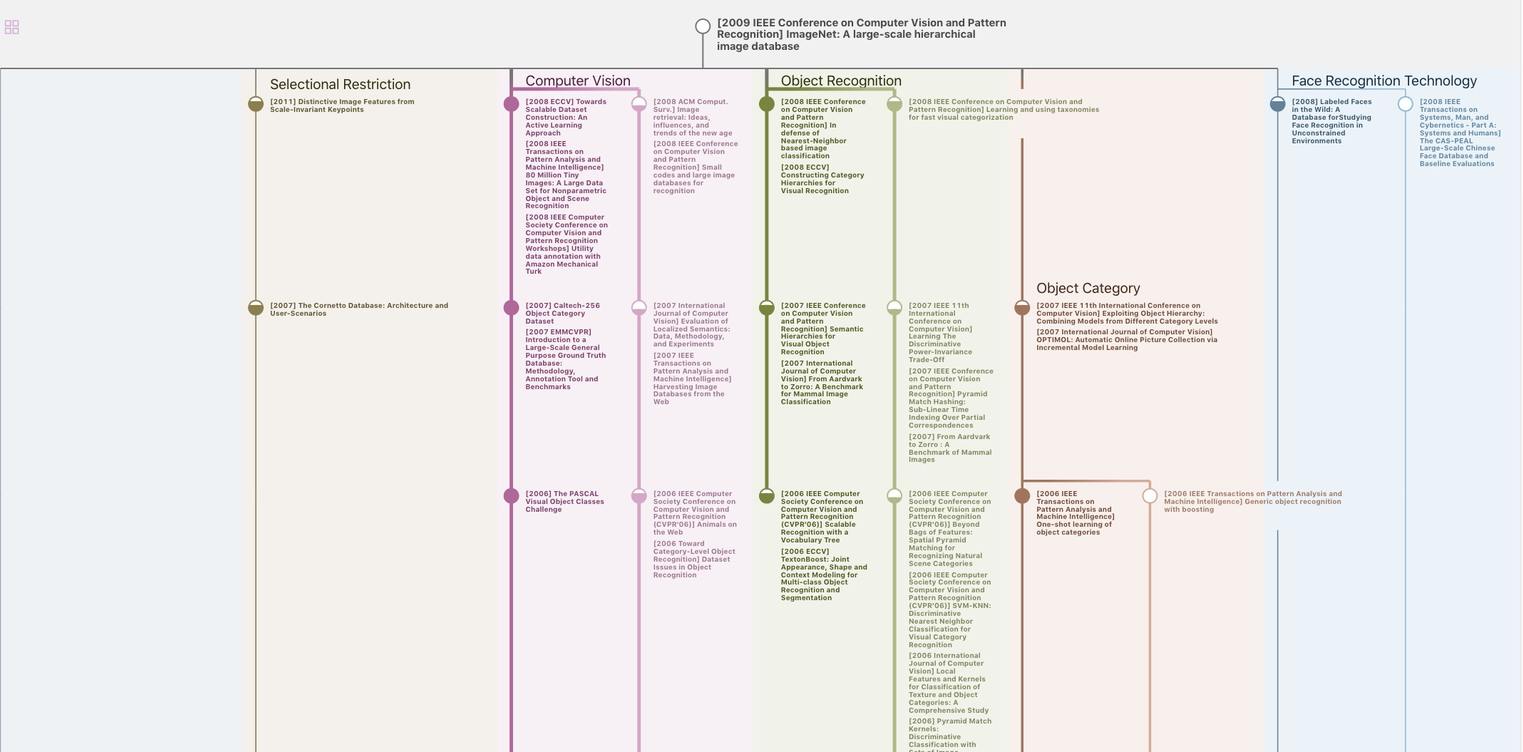
生成溯源树,研究论文发展脉络
Chat Paper
正在生成论文摘要