Pixelhop++: A Small Successive-Subspace-Learning-Based (Ssl-Based) Model For Image Classification
2020 IEEE International Conference on Image Processing (ICIP)(2020)
摘要
The successive subspace learning (SSL) principle was developed and used to design an interpretable learning model, known as the PixelHop method, for image classification in our prior work. Here, we propose an improved PixelHop method and call it Pixel-Hop++. First, to make the PixelHop model size smaller, we decouple a joint spatial-spectral input tensor to multiple spatial tensors (one for each spectral component) under the spatial-spectral separability assumption and perform the Saab transform in a channel-wise manner, called the channel-wise (c/w) Saab transform. Second, by performing this operation from one hop to another successively, we construct a channel-decomposed feature tree whose leaf nodes contain features of one dimension (1D). Third, these 1D features are ranked according to their cross-entropy values, which allows us to select a subset of discriminant features for image classification. In Pixel-Hop++, one can control the learning model size of fine-granularity, offering a flexible tradeoff between the model size and the classification performance. We demonstrate the flexibility of Pixel-Hop++ on MNIST, Fashion MNIST, and CIFAR-10 three datasets.
更多查看译文
关键词
Small learning models,interpretable learning models,successive subspace learning,image classification
AI 理解论文
溯源树
样例
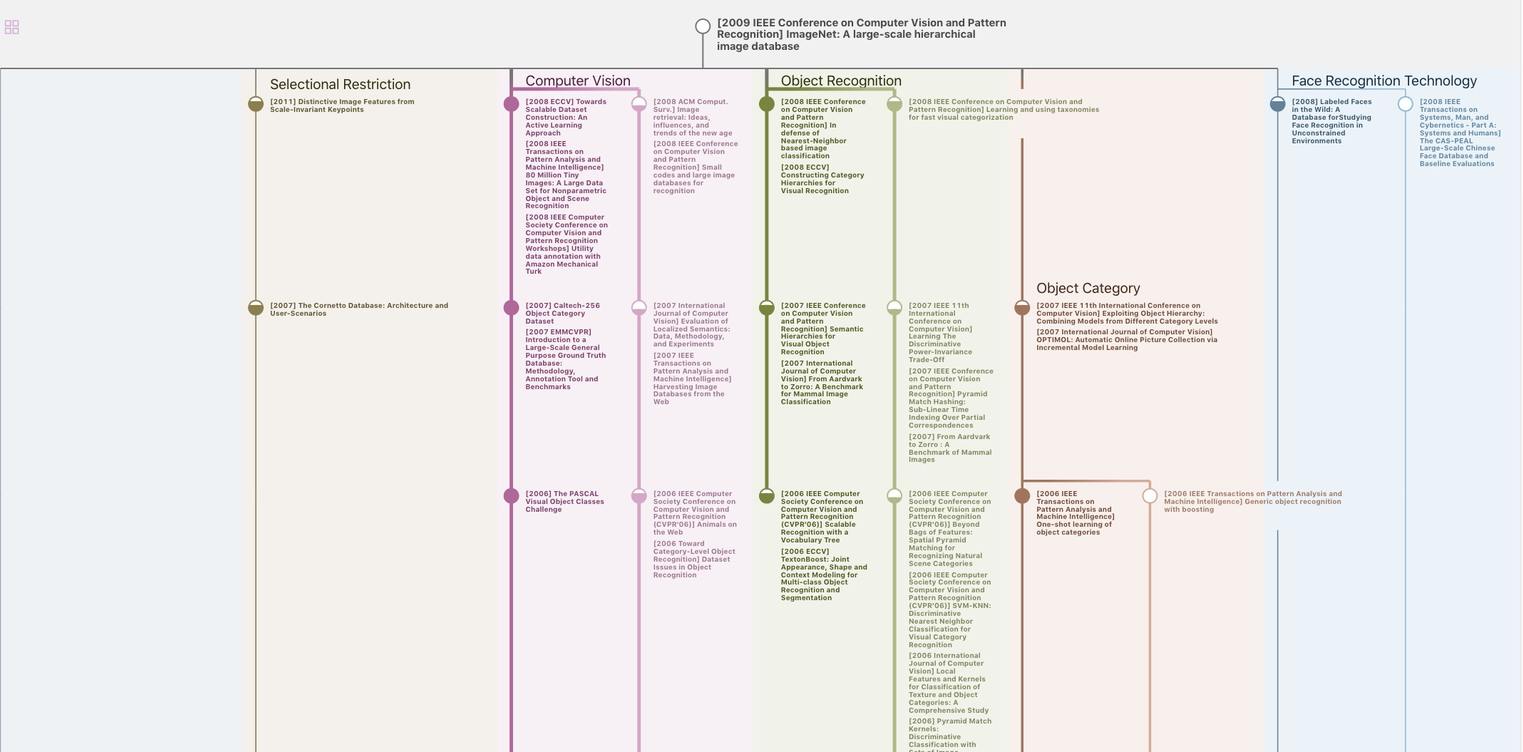
生成溯源树,研究论文发展脉络
Chat Paper
正在生成论文摘要