Method for generating gas leakage data based on Generative Adversarial Networks
2023 7th International Conference on Transportation Information and Safety (ICTIS)(2023)
摘要
To address the problem of small sample sizes in sound signal collection for $SF_{6}$ gas leak fault detection in high-voltage switchgear equipment, an improved StyleGAN model with a U-Net structure as the discriminator is proposed. The model uses a WGAN-GP loss term to reduce the problem of training mode collapse. A consistency loss term and a gradient penalty term are added to the loss evaluation to achieve regularization effects and generate spectrograms with corresponding features for data augmentation of small sample images of leak signal spectrograms. Feasibility experiments are conducted by simulating $SF_{6}$ gas leak audio signal collection. The t-SNE method is used to visually compare the spectrograms of real gas leak data and the “Pseudo-samples” generated by the improved StyleGAN model. The results prove the effectiveness of the proposed method.
更多查看译文
关键词
fault detection,domain generalization,generative adversarial network,few-shot learning,data augmentation
AI 理解论文
溯源树
样例
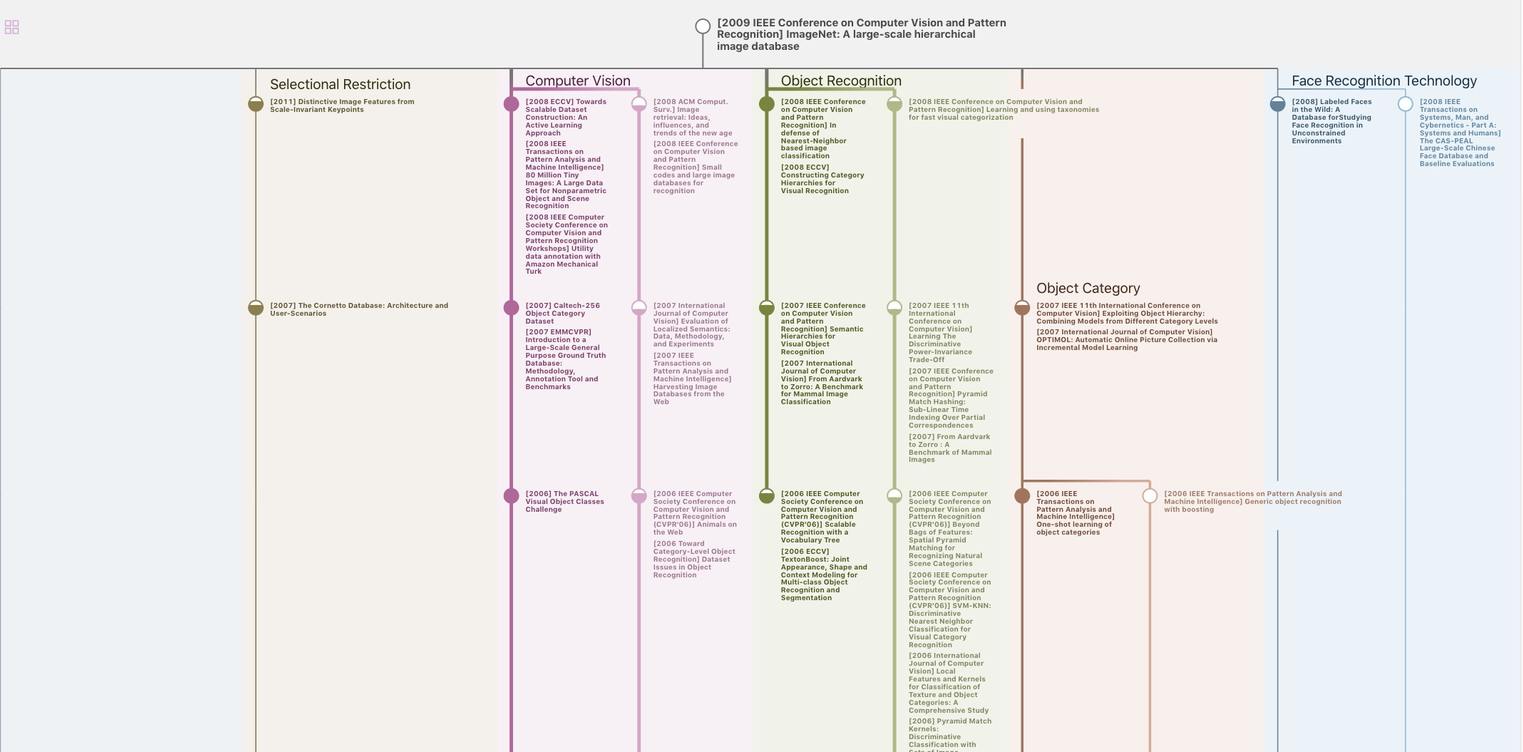
生成溯源树,研究论文发展脉络
Chat Paper
正在生成论文摘要