AlignFuse: Multi-feature Alignment and Fusion for Medical Image Segmentation
2023 8th International Conference on Intelligent Computing and Signal Processing (ICSP)(2023)
Abstract
U-Net, which is a U-shaped network, has made great progress on numerous medical image segmentation tracks. However, even if convolutional neural network (CNN) has a talent for extracting local information, it falls short in capturing global information due to the inherent drawback of the convolution operation. In contrast, whereby the design of tokenized images and self-attention mechanism, Transformer has a brilliant performance in modeling global contextual information but has a poor performance in grasping low-level details on account of the patch division which leads to ignorance of local correlation in each patch. In this paper, we develop a hybrid network structure, AlignFuse, which is in possession of the advantages of both CNN and Transformer. AlignFuse fits CNN and Transformer together in a parallel style, where Transformer leverages the high-level features and CNN pays more attention to details. Moreover, owing to the downsampling operation in CNNs, traditional context-free feature aggregation operations lead to misalignment issues between feature maps. To alleviate this problem, we propose to use Feature Aligned Fusion Module (FAFM) to effectively align and fuse the feature maps from CNN and Transformer in each decoder stage. We conduct experiments on Gland, breast cancer, and automatic cardiac segmentation, with competitive results obtained.
MoreTranslated text
Key words
Alignment,Feature Fusion,Transformer,CNN,Medical Image Segmentation
AI Read Science
Must-Reading Tree
Example
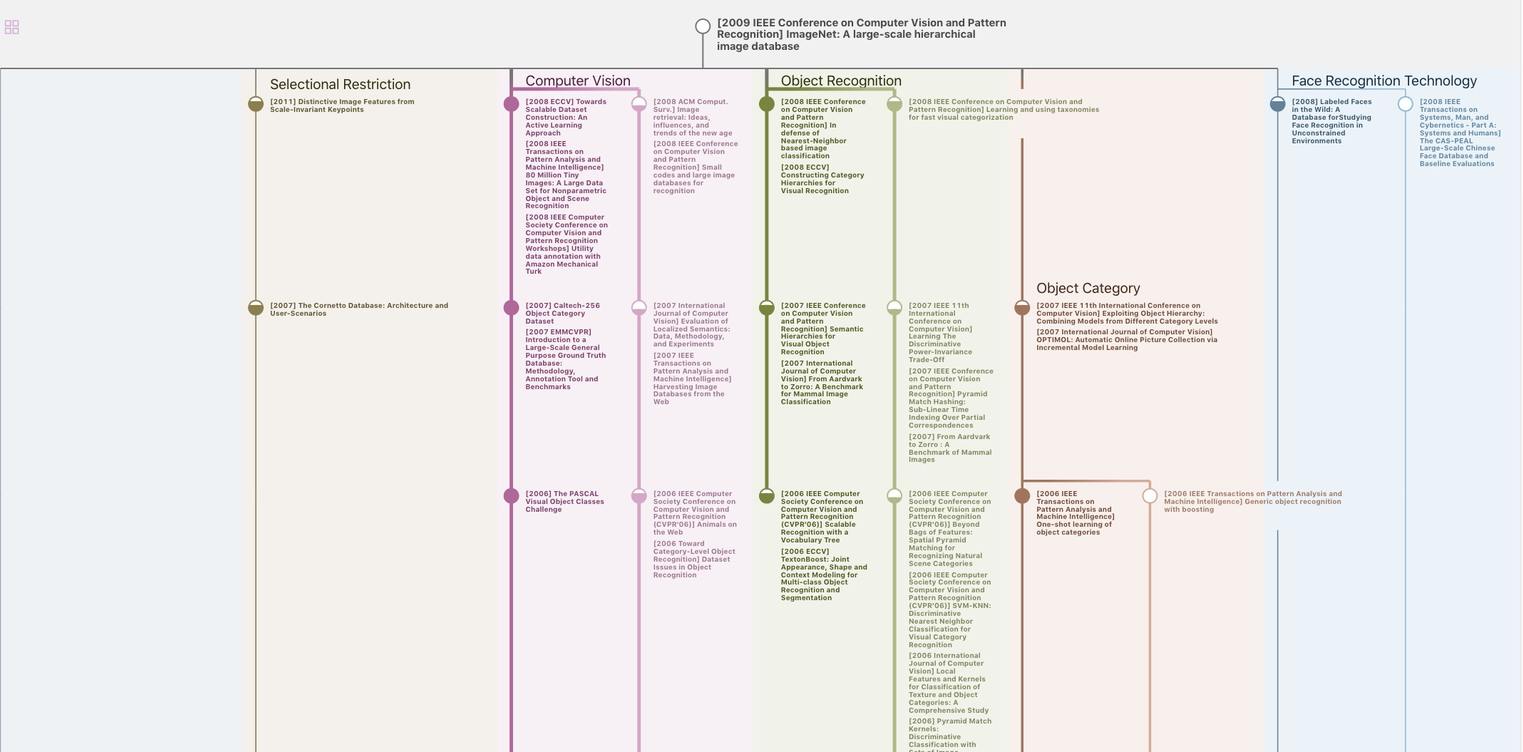
Generate MRT to find the research sequence of this paper
Chat Paper
Summary is being generated by the instructions you defined