FisherRF: Active View Selection and Uncertainty Quantification for Radiance Fields using Fisher Information
CoRR(2023)
Abstract
This study addresses the challenging problem of active view selection and uncertainty quantification within the domain of Radiance Fields. Neural Radiance Fields (NeRF) have greatly advanced image rendering and reconstruction, but the limited availability of 2D images poses uncertainties stemming from occlusions, depth ambiguities, and imaging errors. Efficiently selecting informative views becomes crucial, and quantifying NeRF model uncertainty presents intricate challenges. Existing approaches either depend on model architecture or are based on assumptions regarding density distributions that are not generally applicable. By leveraging Fisher Information, we efficiently quantify observed information within Radiance Fields without ground truth data. This can be used for the next best view selection and pixel-wise uncertainty quantification. Our method overcomes existing limitations on model architecture and effectiveness, achieving state-of-the-art results in both view selection and uncertainty quantification, demonstrating its potential to advance the field of Radiance Fields. Our method with the 3D Gaussian Splatting backend could perform view selections at 70 fps.
MoreTranslated text
AI Read Science
Must-Reading Tree
Example
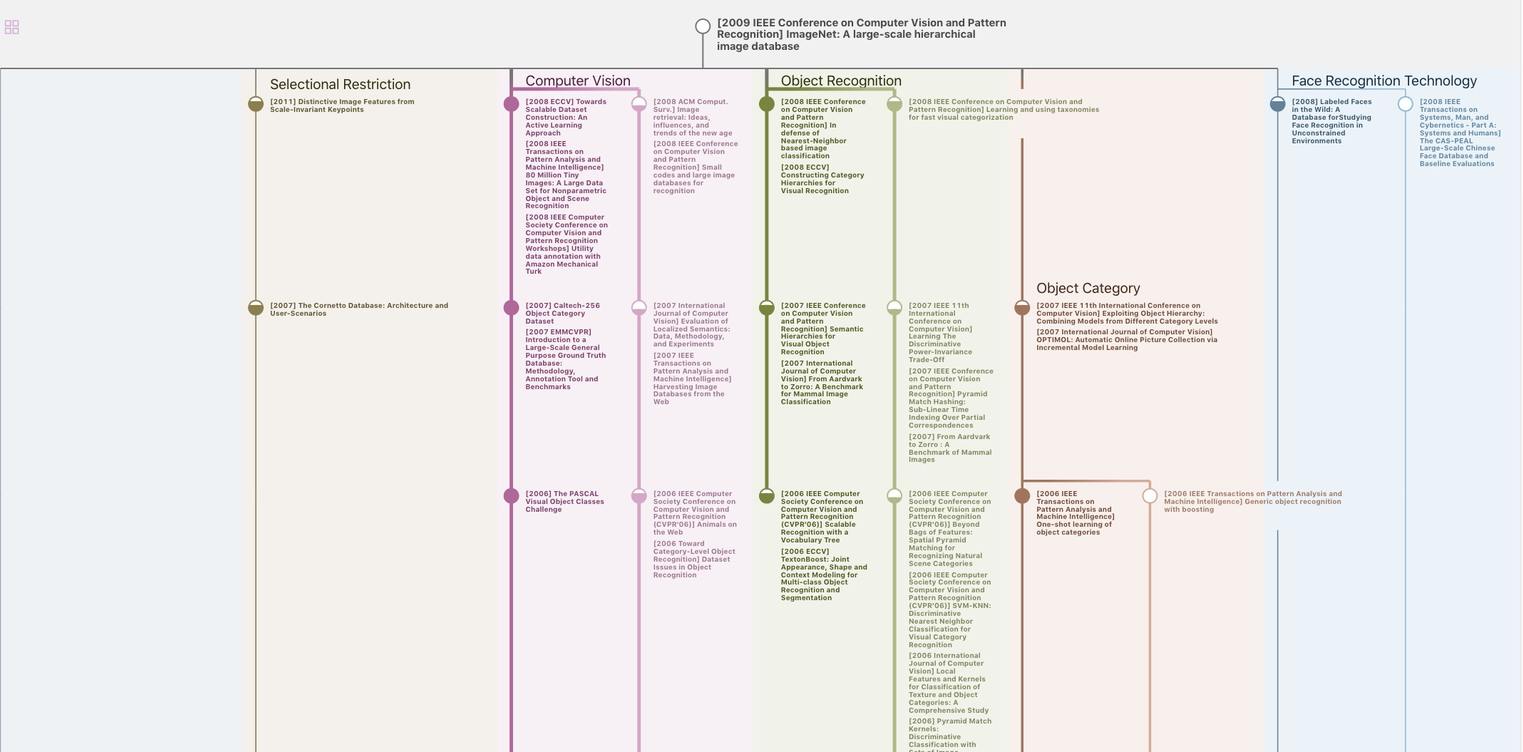
Generate MRT to find the research sequence of this paper
Chat Paper
Summary is being generated by the instructions you defined