Physics-Informed Graph Neural Network for Electromagnetic Simulations
2023 XXXVth General Assembly and Scientific Symposium of the International Union of Radio Science (URSI GASS)(2023)
摘要
Physic-informed neural networks (PINNs) have been widely used in computational physics including electromagnetic simulations. Unlike conventional numerical solvers, PINNs leverage deep neural networks and optimization to find the solution of Maxwell’s equations given specific source and boundary conditions. When the material in the solving domain is heterogeneous, it’s not easy to enforce continuity of electromagnetic fields due to the meshless property of PINNs. On the contrary, graph neural networks (GNNs) explicitly construct a graph (mesh) and apply message passing between the nodes in the graph. In this work, GNNs and PINNs are combined to build physics-informed graph neural networks (PIGNNs) that are flexible in mesh discretization and straightforward to enforce continuity of electromagnetic fields. Moreover, PIGNNs get easier to be trained and converge by resorting to the variational formulation of Maxwell’s equations.
更多查看译文
AI 理解论文
溯源树
样例
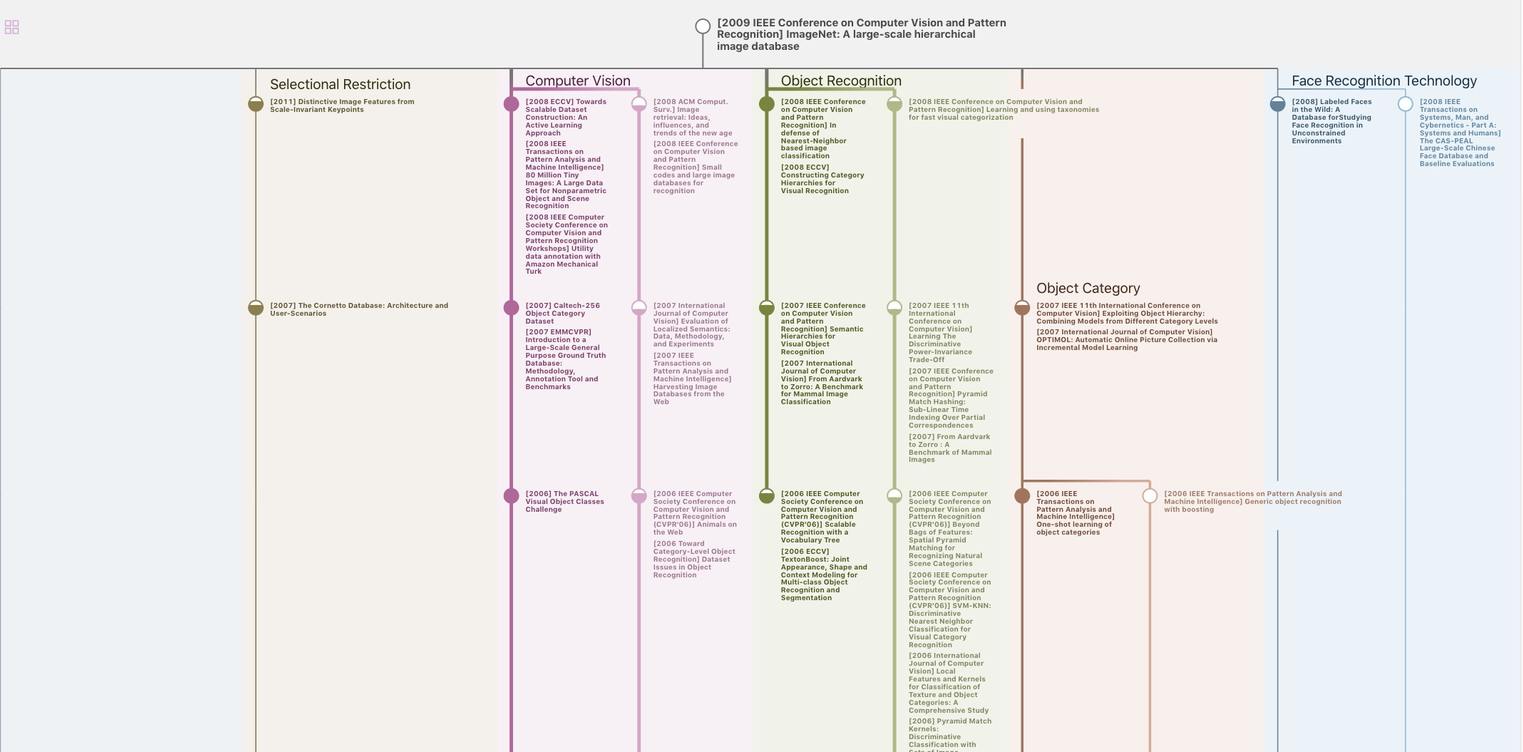
生成溯源树,研究论文发展脉络
Chat Paper
正在生成论文摘要