RETSim: Resilient and Efficient Text Similarity
ICLR 2024(2023)
摘要
This paper introduces RETSim (Resilient and Efficient Text Similarity), a
lightweight, multilingual deep learning model trained to produce robust metric
embeddings for near-duplicate text retrieval, clustering, and dataset
deduplication tasks. We demonstrate that RETSim is significantly more robust
and accurate than MinHash and neural text embeddings, achieving new
state-of-the-art performance on dataset deduplication, adversarial text
retrieval benchmarks, and spam clustering tasks. We also introduce the W4NT3D
benchmark (Wiki-40B 4dversarial Near-T3xt Dataset) for evaluating multilingual,
near-duplicate text retrieval capabilities under adversarial settings. RETSim
and the W4NT3D benchmark are open-sourced under the MIT License at
https://github.com/google/unisim.
更多查看译文
关键词
text similarity,text embedding,metric learning,near-duplicate detection,dataset deduplication
AI 理解论文
溯源树
样例
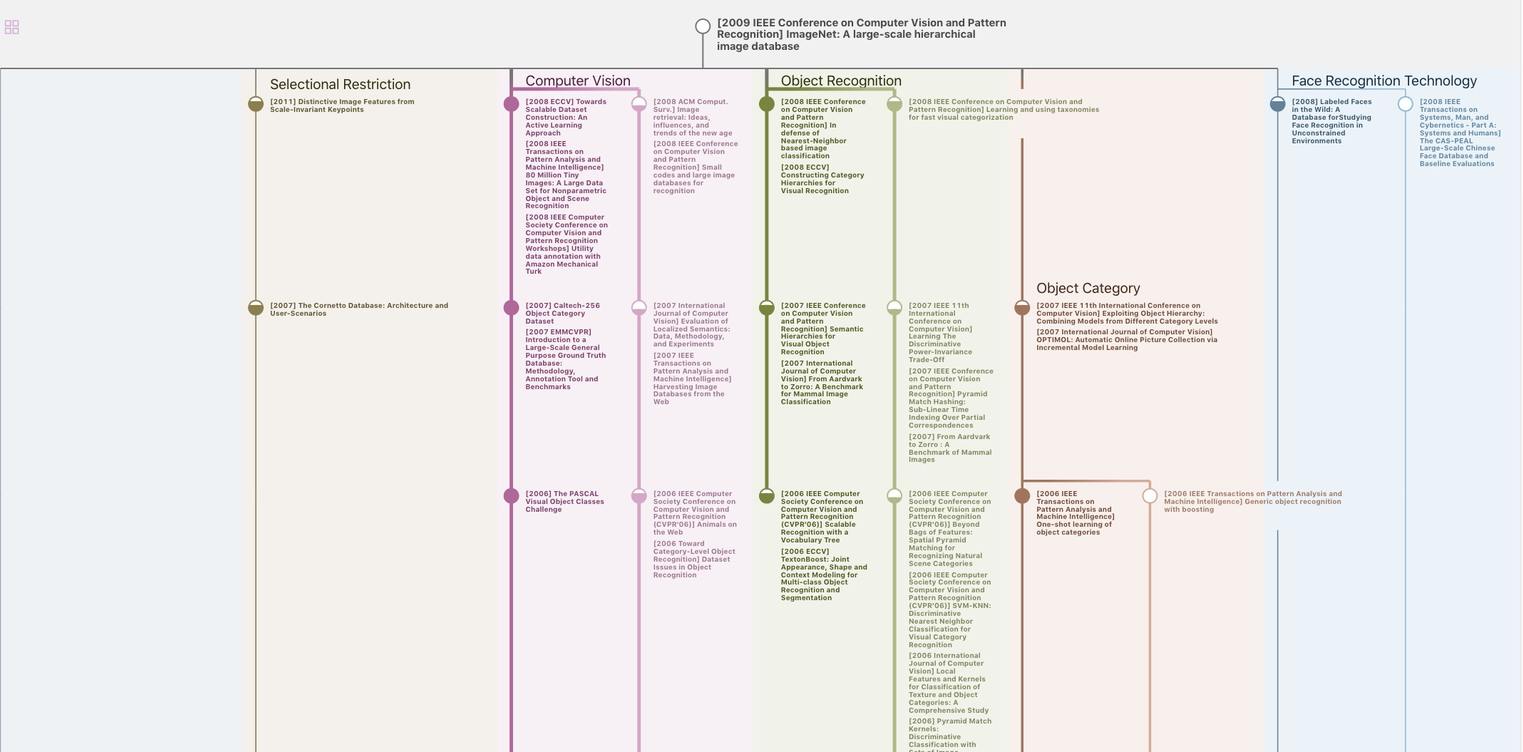
生成溯源树,研究论文发展脉络
Chat Paper
正在生成论文摘要