A Data-Driven Forecast Model of Ionospheric Slant Total Electron Content Based on Decision Trees
2023 International Applied Computational Electromagnetics Society Symposium (ACES-China)(2023)
Abstract
The variability of ionospheric plasma exhibits irregularity at the micro-scale, but at the macro-level, the variation of ionospheric electron density can be predicted. Accurate prediction of ionospheric electron density is of great significance in wireless communication, navigation, and space weather forecasting. In this paper, we develop a data-driven prediction model using decision tree methodology based on the global navigation satellite system (GNSS) observation data and solar activity index, which achieves a single-station forecast model for ionospheric slant total electron content (STEC) one hour in advance. The prediction results for station (35°N,114°E) show that the root mean square error (RMSE) of STEC prediction using this method is 0.32 TECU and 0.48 TECU for the next ten and twenty minutes, respectively. After incorporating the solar activity index and geomagnetic disturbance index into the prediction factors, the accuracy of the model increases by 9.53%. Our research results demonstrate that the data-driven decision tree model for short-term ionospheric STEC prediction has high accuracy and reliability. This method has potential application values in improving GNSS positioning and navigation accuracy, optimizing communication system performance, and enhancing the monitoring capability of ionospheric anomalous behavior.
MoreTranslated text
Key words
Data-driven,STEC prediction,decision tree,single- station modeling
AI Read Science
Must-Reading Tree
Example
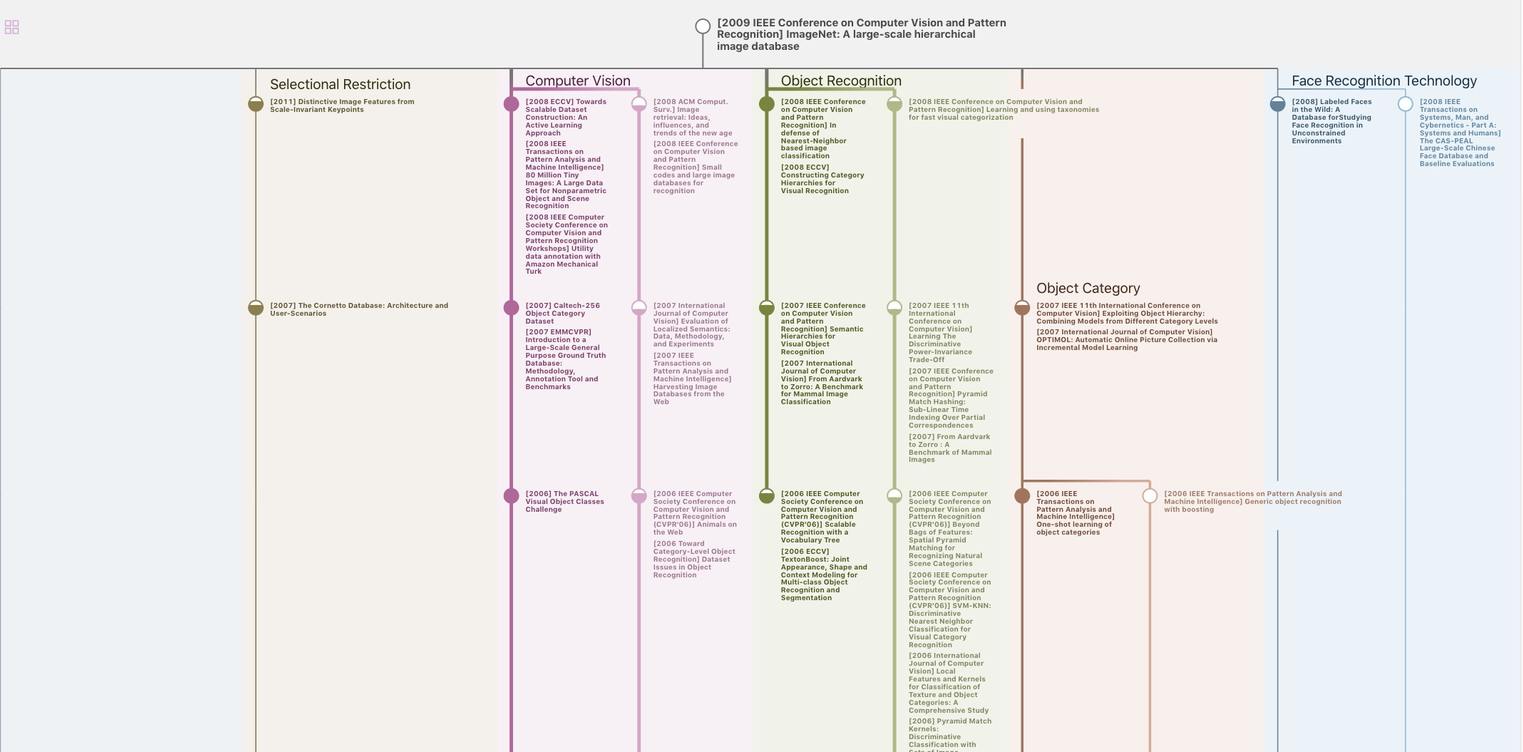
Generate MRT to find the research sequence of this paper
Chat Paper
Summary is being generated by the instructions you defined