Security Concerns of Adversarial Attack for LSTM/BiLSTM Based Solar Power Forecasting
2023 IEEE Power & Energy Society General Meeting (PESGM)(2023)
摘要
Solar photovoltaics (PV) power generation forecasting has become more crucial with the high use of solar PV resources and high impact on grid stability and reliability. The integration of solar PV can cause extreme stability and reliability issues due to its high dependence on weather conditions. This study investigates the impact of adversarial attack and training methods on Long Short-Term Memory (LSTM) and Bidirectional (BiLSTM) based solar power generation forecasts. Results are evaluated for models' responses against adversarial attacks with and without adversarial training in terms of the selected performance metrics. The results show that LSTM and BiLSTM-based models are dramatically vulnerable to adversarial attacks without adversarial training. It is also indicated that the adversarial training method effectively defends the LSTM and BiLSTM-based solar power generation models against adversarial attacks.
更多查看译文
关键词
Long Short-Term Memory (LSTM),Bidirectional (BiLSTM),adversarial attack,adversarial training,solar power generation forecasting
AI 理解论文
溯源树
样例
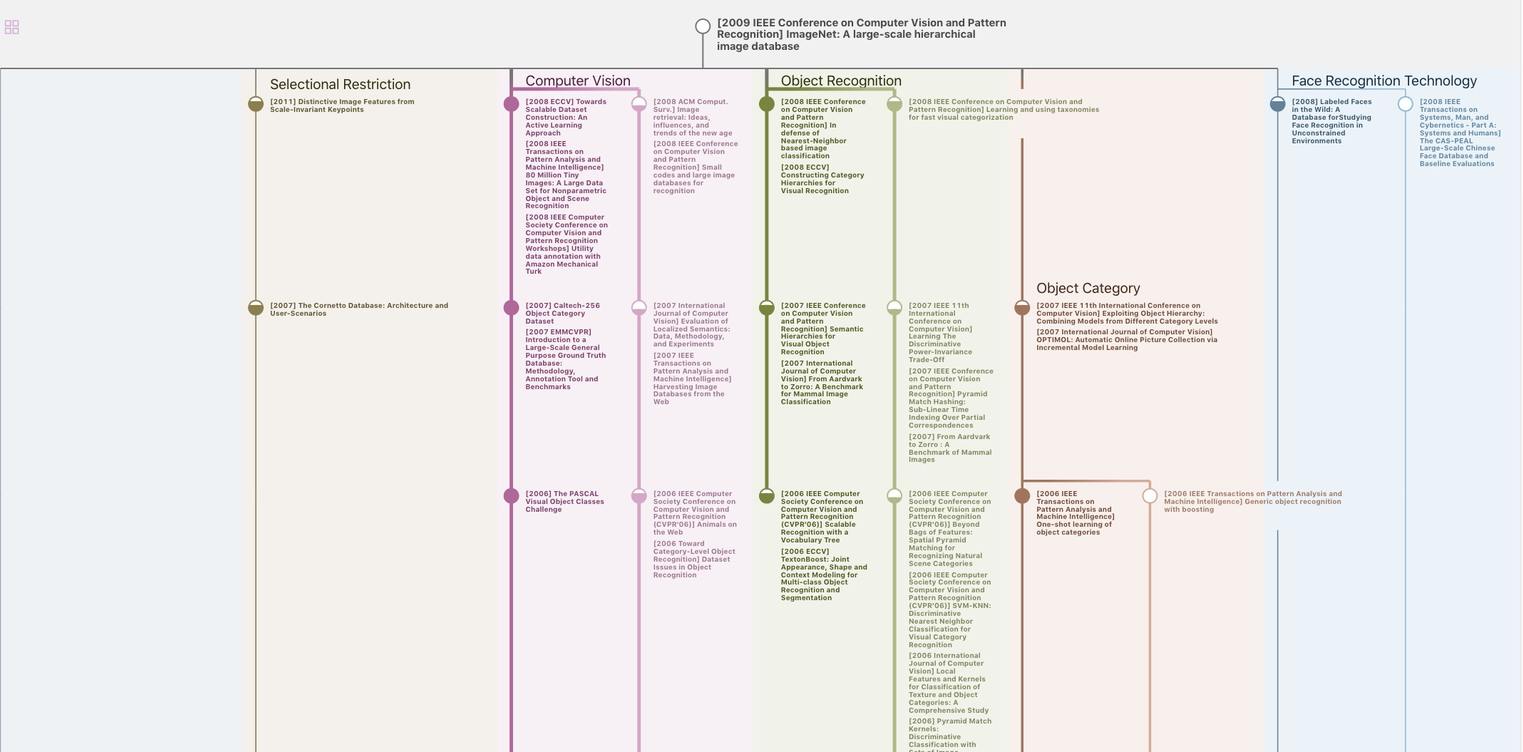
生成溯源树,研究论文发展脉络
Chat Paper
正在生成论文摘要