A Graph Scale Reduction Method for Graph Deep Learning-Based Transient Stability Assessment after Stability Controls
2023 IEEE Power & Energy Society General Meeting (PESGM)(2023)
Abstract
A contingency-based power system stability control strategy is a necessary technique to avoid the effects of severe “N-k” faults. In China, Transient Stability Assessment After Controls (TSAAC) is fundamental for operating and planning the power system. Data-driven graph deep learning (GDL) is promising to promote TSAAC in the graph-type power system in an efficient way. However, GDL applications in real power systems are rare because it is difficult to handle extremely high-dimensional graphs without a reliable scale reduction method. In this paper, an interpretable graph scale reduction method is proposed to generate low-dimensional graphs for the GDL model and apply it to graph deep learning transient stability assessment. First, the improved edge weights are constructed based on the key parameters of transient stability, and partitioning is performed using a hierarchical clustering algorithm. Then, a reduced-dimensional graph is constructed by partitioning and equivalenting the original graph. Finally, a graph attention deep learning model for evaluating the transient stability of complex networks is built using the reduced dimensional graph as input. The validity and accuracy of the model are verified through an analysis of an actual system calculation case in the China Southern Power Grid.
MoreTranslated text
Key words
graph scale reduction, graph attention deep learning, stability assessment, transient stability assessment after controls (TSAAC), transient power angle
AI Read Science
Must-Reading Tree
Example
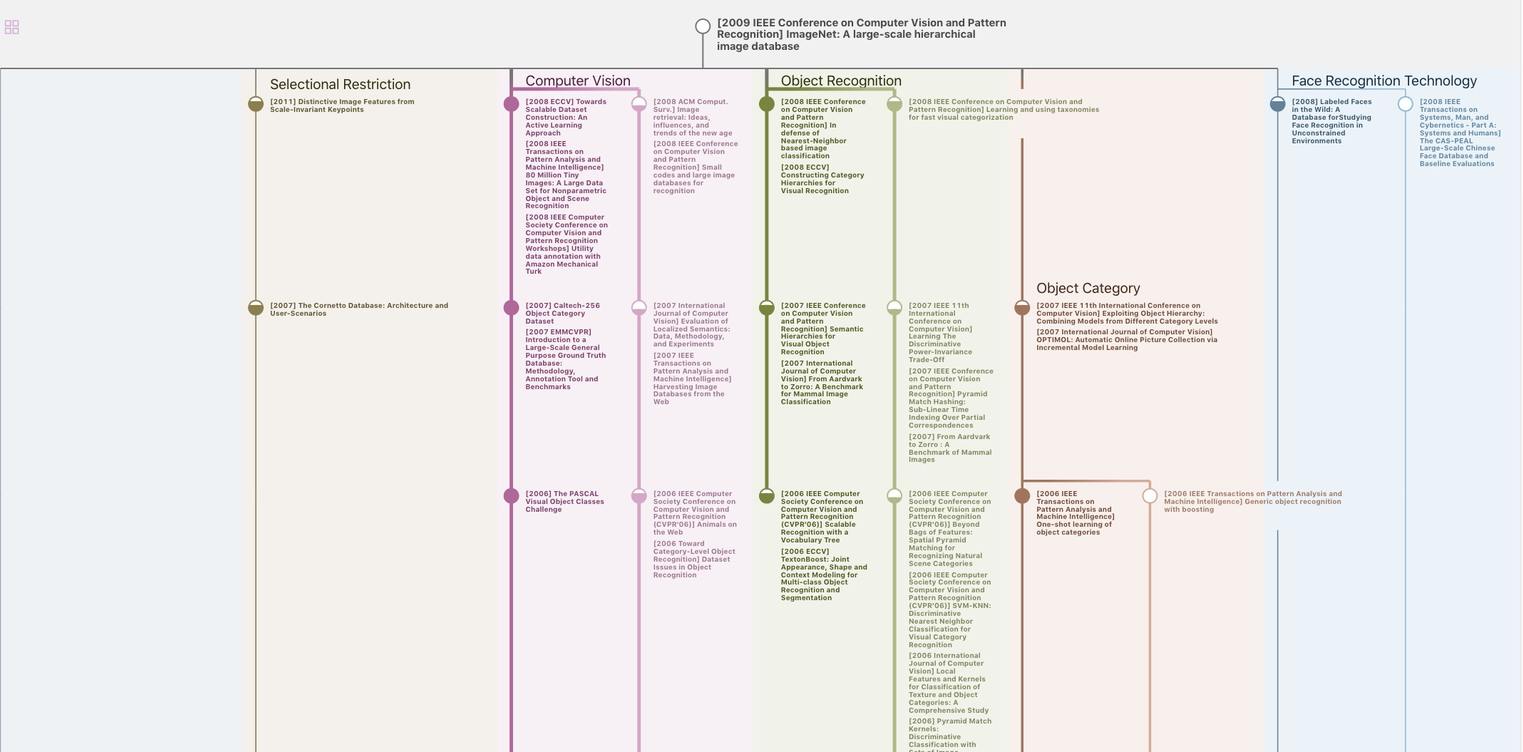
Generate MRT to find the research sequence of this paper
Chat Paper
Summary is being generated by the instructions you defined