Extracting Temporal-Spectral-Spatial Representation of EEG Using Self-Supervised Learning for the Identification of Children with ASD
2023 IEEE 13th International Conference on CYBER Technology in Automation, Control, and Intelligent Systems (CYBER)(2023)
摘要
Autism spectrum disorder is one of the prevalent developmental disabilities, affecting 1%-4% children. Identification of children with ASD is the start of proper treatment, and electroencephalogram (EEG) can be helpful as an important neuroimaging tool. Deep learning models showed surprising performance and are expected to solve the problem, but training the model might be impractical due to the limited size of dataset. In this study, we provided a solution for self-supervised EEG pretraining, making the vision transformer (ViT) become available for the learn of internal EEG feature from large dataset, benefiting from the temporal-spectral-spatial localization and long-range correlation within the data structure. The model was pretrained using more than one billion EEG samples collected from more than 4500 participants, and then transferred for the identification of ASD children. The experiment results showed that the pretrained model can achieve an accuracy of 91.3%, and outperformed the model obtained by supervised learning. We demonstrated the big deep learning models can be well trained with the help of universal temporal-spectral-spatial data structure, and the representation can be efficiently extracted using self-supervised learning.
更多查看译文
关键词
ASD children,autism spectrum disorder,big deep learning models,billion EEG samples,electroencephalogram,important neuroimaging tool,long-range correlation,pretrained model,prevalent developmental disabilities,self-supervised EEG pretraining,self-supervised learning,surprising performance,temporal-spectral-spatial data structure,temporal-spectral-spatial localization,temporal-spectral-spatial representation,vision transformer
AI 理解论文
溯源树
样例
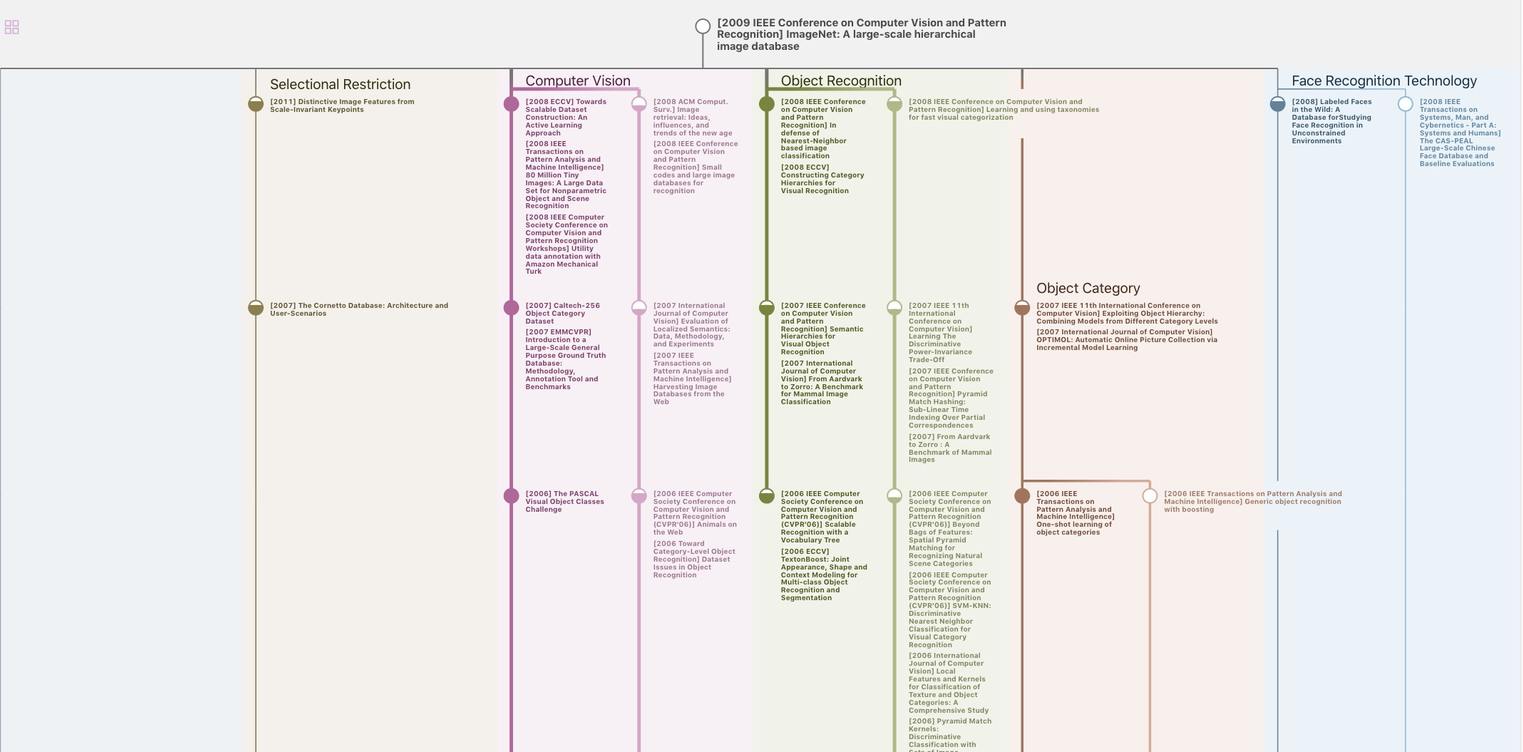
生成溯源树,研究论文发展脉络
Chat Paper
正在生成论文摘要