Improving Generalization of Model-Agnostic Meta-Learning by Channel Exchanging
2022 International Conference on Electronics and Devices, Computational Science (ICEDCS)(2022)
摘要
Among the few-shot learning algorithms, model-agnostic meta-learning (MAML)can quickly learn new tasks with only a small amount of labeled training data, and achieve impressive results. However, due to the small number of samples, the generalization of the model is poor. In this regard, a method called channel exchanging is adopted, which uses the scaling factor of the batch normalization layer to measure the importance of each channel, and replaces the unimportant channels of each class with the feature mean of the channels of other classes. At the same time, contrastive loss is used to perform contrastive learning between the original channel and the exchanged channel, and the obtained loss value is passed into the external circulation as additional priori knowledge for training to obtain a better optimization direction. This method can enhance the ability to learn information interactively between classes in model-agnostic meta-learning, and mine potential differences and connections between classes, thereby improving the ability to generalize to new things. A model-agnostic meta-learning framework based on channel exchanging (EX-MAML) is built. The method fits with the way humans learn new things. Finally, the experimental results show that the performance of EX-MAML is improved on traditional few-shot datasets, and its generalization performance is further verified on other few-shot datasets from different sources.
更多查看译文
关键词
Machine learning,Few-shot learning,Model-agnostic Meta-learning,Channel exchanging,Contrastive loss
AI 理解论文
溯源树
样例
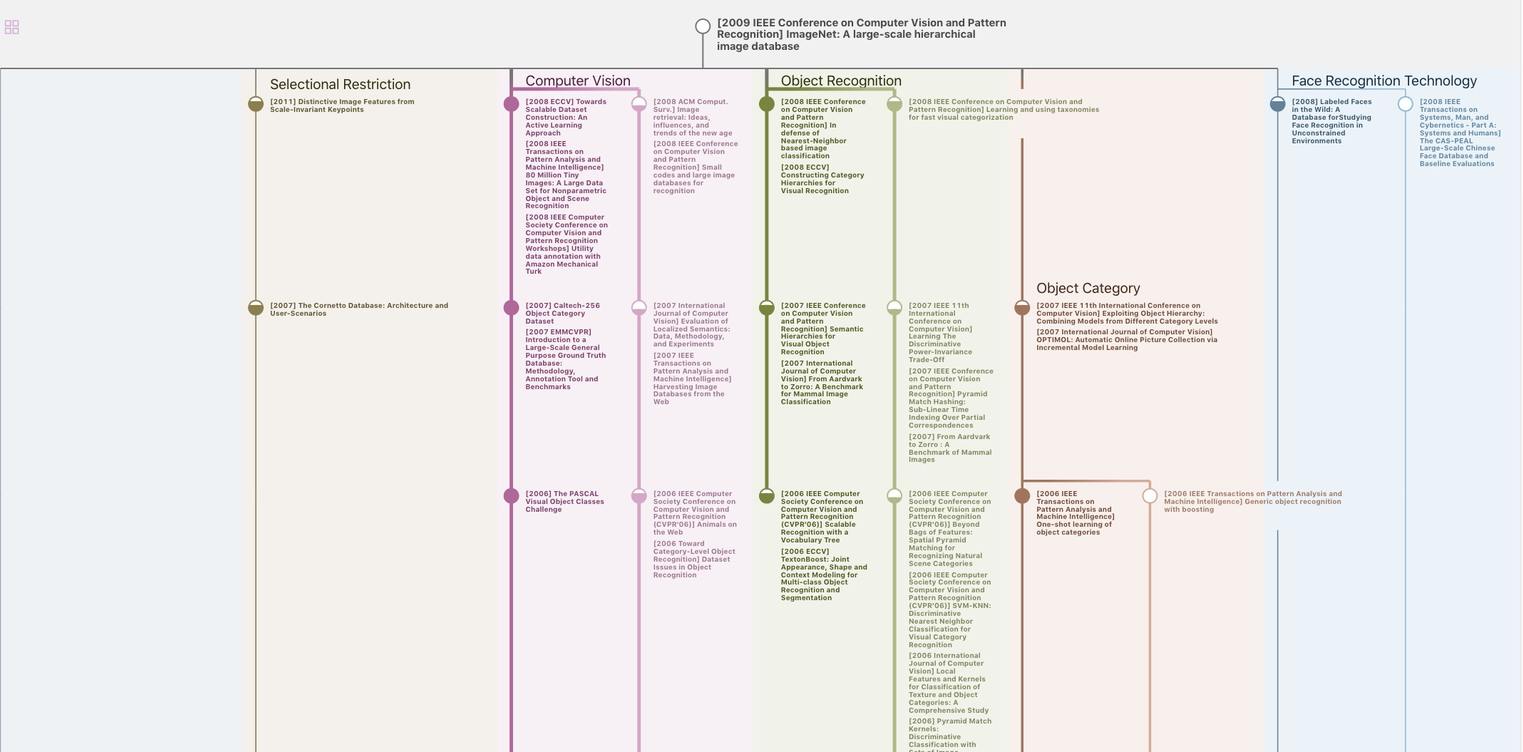
生成溯源树,研究论文发展脉络
Chat Paper
正在生成论文摘要